ultralytics 8.0.101
mosaic9() and loss bug fixes (#2608)
Co-authored-by: Laughing <61612323+Laughing-q@users.noreply.github.com> Co-authored-by: pre-commit-ci[bot] <66853113+pre-commit-ci[bot]@users.noreply.github.com> Co-authored-by: Yonghye Kwon <developer.0hye@gmail.com>
This commit is contained in:
@ -29,9 +29,8 @@ RUN pip install --no-cache tqdm matplotlib pyyaml psutil thop pandas onnx "numpy
|
||||
RUN pip install --no-cache -e .
|
||||
|
||||
# Resolve duplicate OpenCV installation issues in https://github.com/ultralytics/ultralytics/issues/2407
|
||||
RUN apt-get remove `dpkg -l | grep opencv | awk '{print $2}'`
|
||||
RUN apt_packages=$(dpkg -l | grep opencv | awk '{print $2}') && [ -n "$apt_packages" ] && apt-get remove -y $apt_packages || true
|
||||
RUN pip uninstall -y opencv-python
|
||||
RUN rm /usr/local/lib/python3.8/dist-packages/cv2 # Optional
|
||||
RUN pip install "opencv-python<4.7"
|
||||
|
||||
# Set environment variables
|
||||
|
@ -1,7 +1,87 @@
|
||||
---
|
||||
comments: true
|
||||
description: Discover the Objects365 dataset, designed for object detection research with a focus on diverse objects, featuring 365 categories, 2 million images, and 30 million bounding boxes.
|
||||
---
|
||||
|
||||
# 🚧 Page Under Construction ⚒
|
||||
# Objects365 Dataset
|
||||
|
||||
This page is currently under construction!️ 👷Please check back later for updates. 😃🔜
|
||||
The [Objects365](https://www.objects365.org/) dataset is a large-scale, high-quality dataset designed to foster object detection research with a focus on diverse objects in the wild. Created by a team of [Megvii](https://en.megvii.com/) researchers, the dataset offers a wide range of high-resolution images with a comprehensive set of annotated bounding boxes covering 365 object categories.
|
||||
|
||||
## Key Features
|
||||
|
||||
- Objects365 contains 365 object categories, with 2 million images and over 30 million bounding boxes.
|
||||
- The dataset includes diverse objects in various scenarios, providing a rich and challenging benchmark for object detection tasks.
|
||||
- Annotations include bounding boxes for objects, making it suitable for training and evaluating object detection models.
|
||||
- Objects365 pre-trained models significantly outperform ImageNet pre-trained models, leading to better generalization on various tasks.
|
||||
|
||||
## Dataset Structure
|
||||
|
||||
The Objects365 dataset is organized into a single set of images with corresponding annotations:
|
||||
|
||||
- **Images**: The dataset includes 2 million high-resolution images, each containing a variety of objects across 365 categories.
|
||||
- **Annotations**: The images are annotated with over 30 million bounding boxes, providing comprehensive ground truth information for object detection tasks.
|
||||
|
||||
## Applications
|
||||
|
||||
The Objects365 dataset is widely used for training and evaluating deep learning models in object detection tasks. The dataset's diverse set of object categories and high-quality annotations make it a valuable resource for researchers and practitioners in the field of computer vision.
|
||||
|
||||
## Dataset YAML
|
||||
|
||||
A YAML (Yet Another Markup Language) file is used to define the dataset configuration. It contains information about the dataset's paths, classes, and other relevant information. For the case of the Objects365 Dataset, the `Objects365.yaml` file is maintained at [https://github.com/ultralytics/ultralytics/blob/main/ultralytics/datasets/Objects365.yaml](https://github.com/ultralytics/ultralytics/blob/main/ultralytics/datasets/Objects365.yaml).
|
||||
|
||||
!!! example "ultralytics/datasets/Objects365.yaml"
|
||||
|
||||
```yaml
|
||||
--8<-- "ultralytics/datasets/Objects365.yaml"
|
||||
```
|
||||
|
||||
## Usage
|
||||
|
||||
To train a YOLOv8n model on the Objects365 dataset for 100 epochs with an image size of 640, you can use the following code snippets. For a comprehensive list of available arguments, refer to the model [Training](../../modes/train.md) page.
|
||||
|
||||
!!! example "Train Example"
|
||||
|
||||
=== "Python"
|
||||
|
||||
```python
|
||||
from ultralytics import YOLO
|
||||
|
||||
# Load a model
|
||||
model = YOLO('yolov8n.pt') # load a pretrained model (recommended for training)
|
||||
|
||||
# Train the model
|
||||
model.train(data='Objects365.yaml', epochs=100, imgsz=640)
|
||||
```
|
||||
|
||||
=== "CLI"
|
||||
|
||||
```bash
|
||||
# Start training from a pretrained *.pt model
|
||||
yolo detect train data=Objects365.yaml model=yolov8n.pt epochs=100 imgsz=640
|
||||
```
|
||||
|
||||
## Sample Data and Annotations
|
||||
|
||||
The Objects365 dataset contains a diverse set of high-resolution images with objects from 365 categories, providing rich context for object detection tasks. Here are some examples of the images in the dataset:
|
||||
|
||||
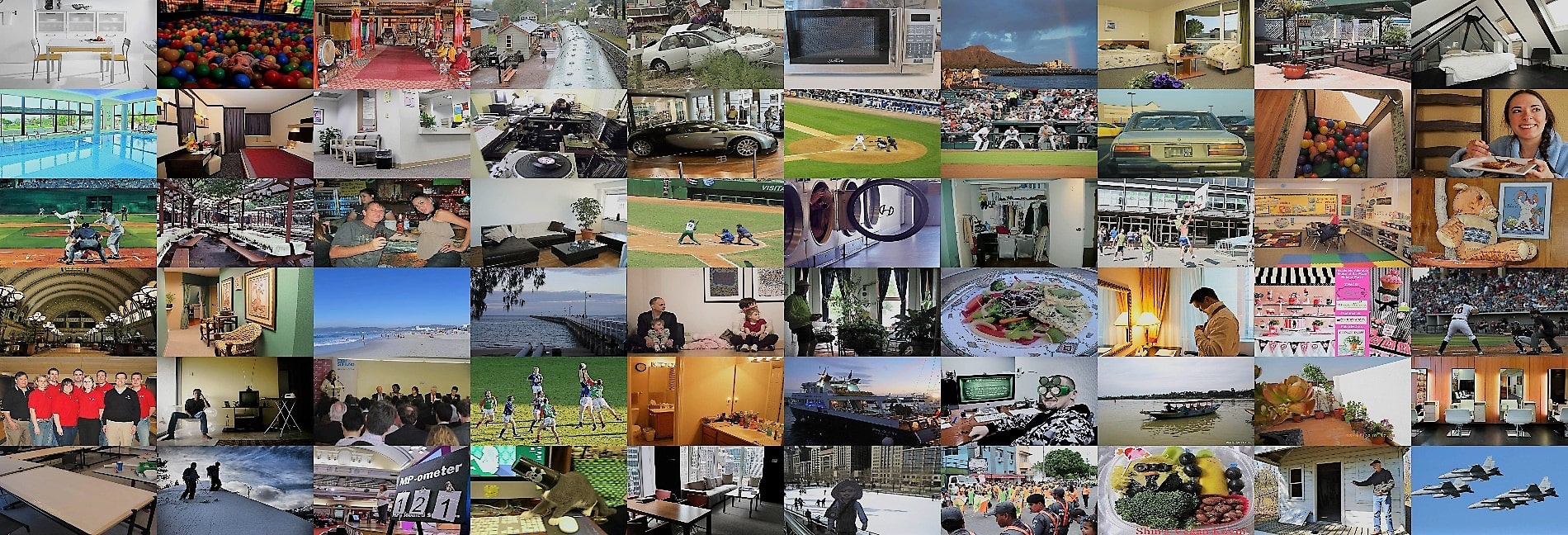
|
||||
|
||||
- **Objects365**: This image demonstrates an example of object detection, where objects are annotated with bounding boxes. The dataset provides a wide range of images to facilitate the development of models for this task.
|
||||
|
||||
The example showcases the variety and complexity of the data in the Objects365 dataset and highlights the importance of accurate object detection for computer vision applications.
|
||||
|
||||
## Citations and Acknowledgments
|
||||
|
||||
If you use the Objects365 dataset in your research or development work, please cite the following paper:
|
||||
|
||||
```bibtex
|
||||
@inproceedings{shao2019objects365,
|
||||
title={Objects365: A Large-scale, High-quality Dataset for Object Detection},
|
||||
author={Shao, Shuai and Li, Zeming and Zhang, Tianyuan and Peng, Chao and Yu, Gang and Li, Jing and Zhang, Xiangyu and Sun, Jian},
|
||||
booktitle={Proceedings of the IEEE/CVF International Conference on Computer Vision},
|
||||
pages={8425--8434},
|
||||
year={2019}
|
||||
}
|
||||
```
|
||||
|
||||
We would like to acknowledge the team of researchers who created and maintain the Objects365 dataset as a valuable resource for the computer vision research community. For more information about the Objects365 dataset and its creators, visit the [Objects365 dataset website](https://www.objects365.org/).
|
||||
|
@ -1,7 +1,87 @@
|
||||
---
|
||||
comments: true
|
||||
description: Explore the SKU-110k dataset, designed for object detection in densely packed retail shelf images, featuring over 110k unique SKU categories and annotations.
|
||||
---
|
||||
|
||||
# 🚧 Page Under Construction ⚒
|
||||
# SKU-110k Dataset
|
||||
|
||||
This page is currently under construction!️ 👷Please check back later for updates. 😃🔜
|
||||
The [SKU-110k](https://github.com/eg4000/SKU110K_CVPR19) dataset is a collection of densely packed retail shelf images, designed to support research in object detection tasks. Developed by Eran Goldman et al., the dataset contains over 110,000 unique store keeping unit (SKU) categories with densely packed objects, often looking similar or even identical, positioned in close proximity.
|
||||
|
||||

|
||||
|
||||
## Key Features
|
||||
|
||||
- SKU-110k contains images of store shelves from around the world, featuring densely packed objects that pose challenges for state-of-the-art object detectors.
|
||||
- The dataset includes over 110,000 unique SKU categories, providing a diverse range of object appearances.
|
||||
- Annotations include bounding boxes for objects and SKU category labels.
|
||||
|
||||
## Dataset Structure
|
||||
|
||||
The SKU-110k dataset is organized into three main subsets:
|
||||
|
||||
1. **Training set**: This subset contains images and annotations used for training object detection models.
|
||||
2. **Validation set**: This subset consists of images and annotations used for model validation during training.
|
||||
3. **Test set**: This subset is designed for the final evaluation of trained object detection models.
|
||||
|
||||
## Applications
|
||||
|
||||
The SKU-110k dataset is widely used for training and evaluating deep learning models in object detection tasks, especially in densely packed scenes such as retail shelf displays. The dataset's diverse set of SKU categories and densely packed object arrangements make it a valuable resource for researchers and practitioners in the field of computer vision.
|
||||
|
||||
## Dataset YAML
|
||||
|
||||
A YAML (Yet Another Markup Language) file is used to define the dataset configuration. It contains information about the dataset's paths, classes, and other relevant information. For the case of the SKU-110K dataset, the `SKU-110K.yaml` file is maintained at [https://github.com/ultralytics/ultralytics/blob/main/ultralytics/datasets/SKU-110K.yaml](https://github.com/ultralytics/ultralytics/blob/main/ultralytics/datasets/SKU-110K.yaml).
|
||||
|
||||
!!! example "ultralytics/datasets/SKU-110K.yaml"
|
||||
|
||||
```yaml
|
||||
--8<-- "ultralytics/datasets/SKU-110K.yaml"
|
||||
```
|
||||
|
||||
## Usage
|
||||
|
||||
To train a YOLOv8n model on the SKU-110K dataset for 100 epochs with an image size of 640, you can use the following code snippets. For a comprehensive list of available arguments, refer to the model [Training](../../modes/train.md) page.
|
||||
|
||||
!!! example "Train Example"
|
||||
|
||||
=== "Python"
|
||||
|
||||
```python
|
||||
from ultralytics import YOLO
|
||||
|
||||
# Load a model
|
||||
model = YOLO('yolov8n.pt') # load a pretrained model (recommended for training)
|
||||
|
||||
# Train the model
|
||||
model.train(data='SKU-110K.yaml', epochs=100, imgsz=640)
|
||||
```
|
||||
|
||||
=== "CLI"
|
||||
|
||||
```bash
|
||||
# Start training from a pretrained *.pt model
|
||||
yolo detect train data=SKU-110K.yaml model=yolov8n.pt epochs=100 imgsz=640
|
||||
|
||||
## Sample Data and Annotations
|
||||
|
||||
The SKU-110k dataset contains a diverse set of retail shelf images with densely packed objects, providing rich context for object detection tasks. Here are some examples of data from the dataset, along with their corresponding annotations:
|
||||
|
||||
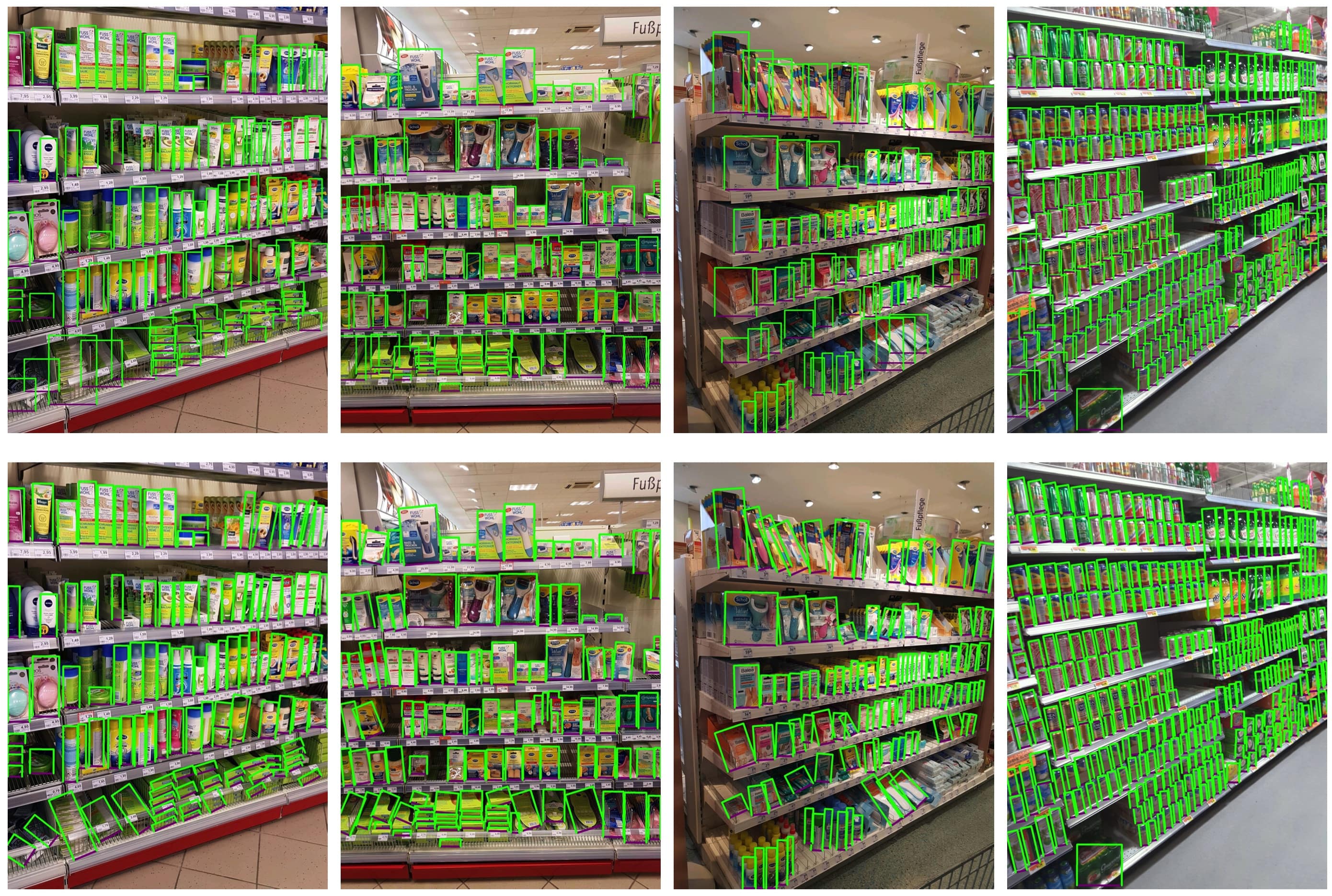
|
||||
|
||||
- **Densely packed retail shelf image**: This image demonstrates an example of densely packed objects in a retail shelf setting. Objects are annotated with bounding boxes and SKU category labels.
|
||||
|
||||
The example showcases the variety and complexity of the data in the SKU-110k dataset and highlights the importance of high-quality data for object detection tasks.
|
||||
|
||||
## Citations and Acknowledgments
|
||||
|
||||
If you use the SKU-110k dataset in your research or development work, please cite the following paper:
|
||||
|
||||
```bibtex
|
||||
@inproceedings{goldman2019dense,
|
||||
author = {Eran Goldman and Roei Herzig and Aviv Eisenschtat and Jacob Goldberger and Tal Hassner},
|
||||
title = {Precise Detection in Densely Packed Scenes},
|
||||
booktitle = {Proc. Conf. Comput. Vision Pattern Recognition (CVPR)},
|
||||
year = {2019}
|
||||
}
|
||||
```
|
||||
|
||||
We would like to acknowledge Eran Goldman et al. for creating and maintaining the SKU-110k dataset as a valuable resource for the computer vision research community. For more information about the SKU-110k dataset and its creators, visit the [SKU-110k dataset GitHub repository](https://github.com/eg4000/SKU110K_CVPR19).
|
@ -1,7 +1,111 @@
|
||||
---
|
||||
comments: true
|
||||
description: Discover the VisDrone dataset, a comprehensive benchmark for drone-based computer vision tasks, including object detection, tracking, and crowd counting.
|
||||
---
|
||||
|
||||
# 🚧 Page Under Construction ⚒
|
||||
# VisDrone Dataset
|
||||
|
||||
This page is currently under construction!️ 👷Please check back later for updates. 😃🔜
|
||||
The [VisDrone Dataset](https://github.com/VisDrone/VisDrone-Dataset) is a large-scale benchmark created by the AISKYEYE team at the Lab of Machine Learning and Data Mining, Tianjin University, China. It contains carefully annotated ground truth data for various computer vision tasks related to drone-based image and video analysis.
|
||||
|
||||
VisDrone is composed of 288 video clips with 261,908 frames and 10,209 static images, captured by various drone-mounted cameras. The dataset covers a wide range of aspects, including location (14 different cities across China), environment (urban and rural), objects (pedestrians, vehicles, bicycles, etc.), and density (sparse and crowded scenes). The dataset was collected using various drone platforms under different scenarios and weather and lighting conditions. These frames are manually annotated with over 2.6 million bounding boxes of targets such as pedestrians, cars, bicycles, and tricycles. Attributes like scene visibility, object class, and occlusion are also provided for better data utilization.
|
||||
|
||||
The challenge mainly focuses on five tasks:
|
||||
|
||||
1. **Task 1**: Object detection in images challenge - Detect objects of predefined categories (e.g., cars and pedestrians) from individual images taken from drones.
|
||||
2. **Task 2**: Object detection in videos challenge - Similar to Task 1, except that objects are required to be detected from videos.
|
||||
3. **Task 3**: Single-object tracking challenge - Estimate the state of a target, indicated in the first frame, in the subsequent video frames.
|
||||
4. **Task 4**: Multi-object tracking challenge - Recover the trajectories of objects in each video frame.
|
||||
5. **Task 5**: Crowd counting challenge - Count persons in each video frame.
|
||||
|
||||
## Citation
|
||||
|
||||
If you use the VisDrone dataset in your research or development work, please cite the following paper:
|
||||
|
||||
```bibtex
|
||||
@ARTICLE{9573394,
|
||||
author={Zhu, Pengfei and Wen, Longyin and Du, Dawei and Bian, Xiao and Fan, Heng and Hu, Qinghua and Ling, Haibin},
|
||||
journal={IEEE Transactions on Pattern Analysis and Machine Intelligence},
|
||||
title={Detection and Tracking Meet Drones Challenge},
|
||||
year={2021},
|
||||
volume={},
|
||||
number={},
|
||||
pages={1-1},
|
||||
doi={10.1109/TPAMI.2021.3119563}}
|
||||
```
|
||||
|
||||
## Dataset Structure
|
||||
|
||||
The VisDrone dataset is organized into five main subsets, each focusing on a specific task:
|
||||
|
||||
1. **Task 1**: Object detection in images
|
||||
2. **Task 2**: Object detection in videos
|
||||
3. **Task 3**: Single-object tracking
|
||||
4. **Task 4**: Multi-object tracking
|
||||
5. **Task 5**: Crowd counting
|
||||
|
||||
## Applications
|
||||
|
||||
The VisDrone dataset is widely used for training and evaluating deep learning models in drone-based computer vision tasks such as object detection, object tracking, and crowd counting. The dataset's diverse set of sensor data, object annotations, and attributes make it a valuable resource for researchers and practitioners in the field of drone-based computer vision.
|
||||
|
||||
## Dataset YAML
|
||||
|
||||
A YAML (Yet Another Markup Language) file is used to define the dataset configuration. It contains information about the dataset's paths, classes, and other relevant information. In the case of the Visdrone dataset, the `VisDrone.yaml` file is maintained at [https://github.com/ultralytics/ultralytics/blob/main/ultralytics/datasets/VisDrone.yaml](https://github.com/ultralytics/ultralytics/blob/main/ultralytics/datasets/VisDrone.yaml).
|
||||
|
||||
!!! example "ultralytics/datasets/VisDrone.yaml"
|
||||
|
||||
```yaml
|
||||
--8<-- "ultralytics/datasets/VisDrone.yaml"
|
||||
```
|
||||
|
||||
## Usage
|
||||
|
||||
To train a YOLOv8n model on the VisDrone dataset for 100 epochs with an image size of 640, you can use the following code snippets. For a comprehensive list of available arguments, refer to the model [Training](../../modes/train.md) page.
|
||||
|
||||
!!! example "Train Example"
|
||||
|
||||
=== "Python"
|
||||
|
||||
```python
|
||||
from ultralytics import YOLO
|
||||
|
||||
# Load a model
|
||||
model = YOLO('yolov8n.pt') # load a pretrained model (recommended for training)
|
||||
|
||||
# Train the model
|
||||
model.train(data='VisDrone.yaml', epochs=100, imgsz=640)
|
||||
```
|
||||
|
||||
=== "CLI"
|
||||
|
||||
```bash
|
||||
# Start training from a pretrained *.pt model
|
||||
yolo detect train data=VisDrone.yaml model=yolov8n.pt epochs=100 imgsz=640
|
||||
```
|
||||
|
||||
## Sample Data and Annotations
|
||||
|
||||
The VisDrone dataset contains a diverse set of images and videos captured by drone-mounted cameras. Here are some examples of data from the dataset, along with their corresponding annotations:
|
||||
|
||||
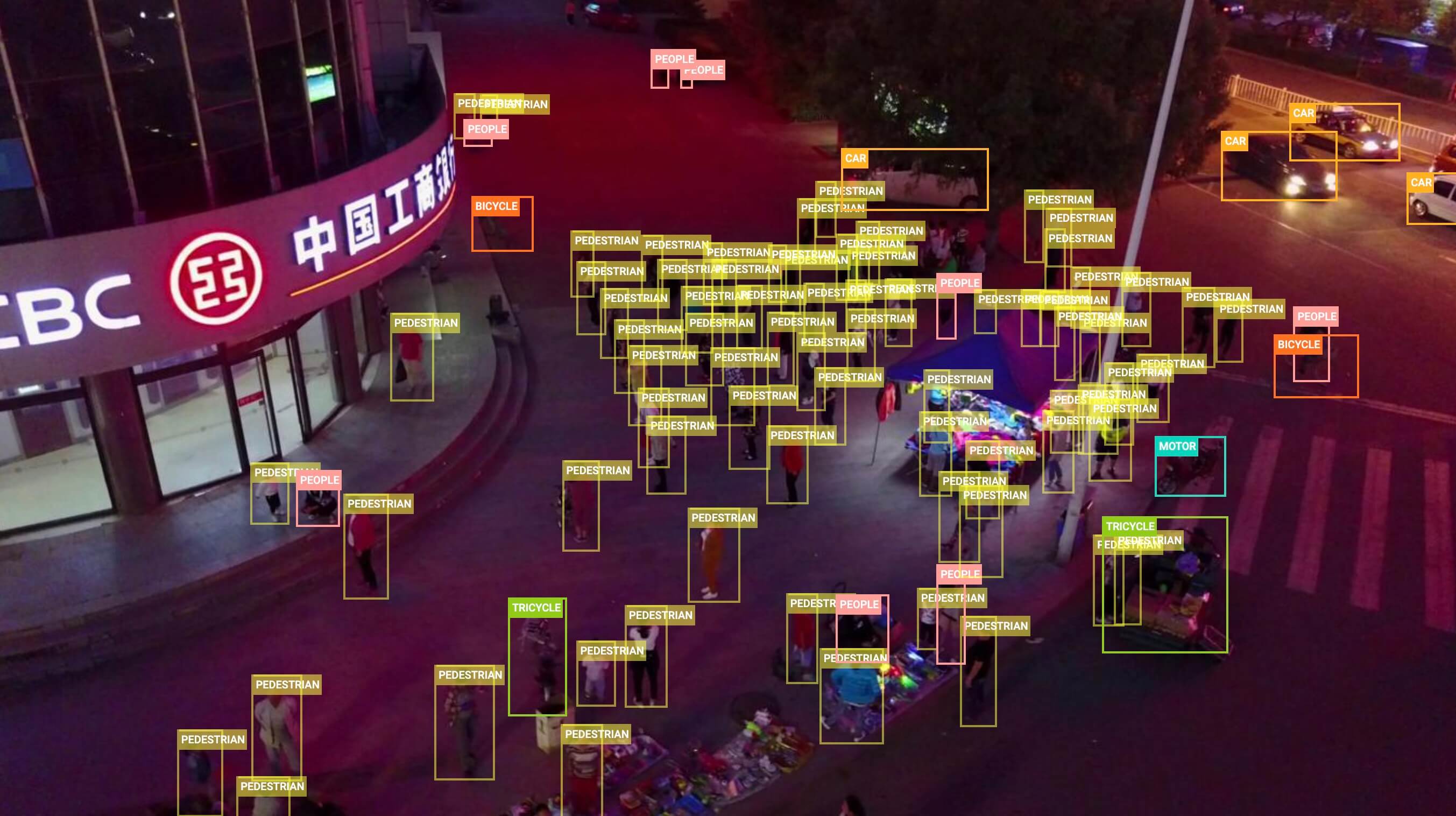
|
||||
|
||||
- **Task 1**: Object detection in images - This image demonstrates an example of object detection in images, where objects are annotated with bounding boxes. The dataset provides a wide variety of images taken from different locations, environments, and densities to facilitate the development of models for this task.
|
||||
|
||||
The example showcases the variety and complexity of the data in the VisDrone dataset and highlights the importance of high-quality sensor data for drone-based computer vision tasks.
|
||||
|
||||
## Citations and Acknowledgments
|
||||
|
||||
If you use the VisDrone dataset in your research or development work, please cite the following paper:
|
||||
|
||||
```bibtex
|
||||
@ARTICLE{9573394,
|
||||
author={Zhu, Pengfei and Wen, Longyin and Du, Dawei and Bian, Xiao and Fan, Heng and Hu, Qinghua and Ling, Haibin},
|
||||
journal={IEEE Transactions on Pattern Analysis and Machine Intelligence},
|
||||
title={Detection and Tracking Meet Drones Challenge},
|
||||
year={2021},
|
||||
volume={},
|
||||
number={},
|
||||
pages={1-1},
|
||||
doi={10.1109/TPAMI.2021.3119563}}
|
||||
```
|
||||
|
||||
We would like to acknowledge the AISKYEYE team at the Lab of Machine Learning and Data Mining, Tianjin University, China, for creating and maintaining the VisDrone dataset as a valuable resource for the drone-based computer vision research community. For more information about the VisDrone dataset and its creators, visit the [VisDrone Dataset GitHub repository](https://github.com/VisDrone/VisDrone-Dataset).
|
@ -64,4 +64,4 @@ If you use RT-DETR in your research or development work, please cite the [origin
|
||||
}
|
||||
```
|
||||
|
||||
We would like to acknowledge Baidu's [PaddlePaddle]((https://github.com/PaddlePaddle/PaddleDetection)) team for creating and maintaining this valuable resource for the computer vision community.
|
||||
We would like to acknowledge Baidu's [PaddlePaddle](https://github.com/PaddlePaddle/PaddleDetection) team for creating and maintaining this valuable resource for the computer vision community.
|
||||
|
@ -1,6 +1,6 @@
|
||||
# Ultralytics YOLO 🚀, AGPL-3.0 license
|
||||
|
||||
__version__ = '8.0.100'
|
||||
__version__ = '8.0.101'
|
||||
|
||||
from ultralytics.hub import start
|
||||
from ultralytics.vit.rtdetr import RTDETR
|
||||
|
@ -145,7 +145,8 @@ class BaseDataset(Dataset):
|
||||
r = self.imgsz / max(h0, w0) # ratio
|
||||
if r != 1: # if sizes are not equal
|
||||
interp = cv2.INTER_LINEAR if (self.augment or r > 1) else cv2.INTER_AREA
|
||||
im = cv2.resize(im, (math.ceil(w0 * r), math.ceil(h0 * r)), interpolation=interp)
|
||||
im = cv2.resize(im, (min(math.ceil(w0 * r), self.imgsz), min(math.ceil(h0 * r), self.imgsz)),
|
||||
interpolation=interp)
|
||||
return im, (h0, w0), im.shape[:2] # im, hw_original, hw_resized
|
||||
return self.ims[i], self.im_hw0[i], self.im_hw[i] # im, hw_original, hw_resized
|
||||
|
||||
|
@ -181,6 +181,8 @@ class BaseTrainer:
|
||||
# Command
|
||||
cmd, file = generate_ddp_command(world_size, self)
|
||||
try:
|
||||
LOGGER.info('Pre-caching dataset to avoid NCCL timeout before running DDP command')
|
||||
deepcopy(self)._setup_train(world_size=0)
|
||||
LOGGER.info(f'Running DDP command {cmd}')
|
||||
subprocess.run(cmd, check=True)
|
||||
except Exception as e:
|
||||
|
Reference in New Issue
Block a user