ultralytics 8.0.100
add Mosaic9() augmentation (#2605)
Co-authored-by: Ayush Chaurasia <ayush.chaurarsia@gmail.com> Co-authored-by: Tommy in Tongji <36354458+TommyZihao@users.noreply.github.com> Co-authored-by: pre-commit-ci[bot] <66853113+pre-commit-ci[bot]@users.noreply.github.com> Co-authored-by: BIGBOSS-FOX <47949596+BIGBOSS-FOX@users.noreply.github.com> Co-authored-by: xbkaishui <xxkaishui@gmail.com>
This commit is contained in:
4
.github/workflows/links.yml
vendored
4
.github/workflows/links.yml
vendored
@ -28,7 +28,7 @@ jobs:
|
||||
timeout_minutes: 5
|
||||
retry_wait_seconds: 60
|
||||
max_attempts: 3
|
||||
command: lychee --accept 429,999 --exclude-loopback --exclude twitter.com --exclude-path '**/ci.yaml' --exclude-mail --github-token ${{ secrets.GITHUB_TOKEN }} './**/*.md' './**/*.html'
|
||||
command: lychee --accept 429,999 --exclude-loopback --exclude 'https?://(www\.)?(twitter\.com|instagram\.com)' --exclude-path '**/ci.yaml' --exclude-mail --github-token ${{ secrets.GITHUB_TOKEN }} './**/*.md' './**/*.html'
|
||||
|
||||
- name: Test Markdown, HTML, YAML, Python and Notebook links with retry
|
||||
if: github.event_name == 'workflow_dispatch'
|
||||
@ -37,4 +37,4 @@ jobs:
|
||||
timeout_minutes: 5
|
||||
retry_wait_seconds: 60
|
||||
max_attempts: 3
|
||||
command: lychee --accept 429,999 --exclude-loopback --exclude twitter.com,url.com --exclude-path '**/ci.yaml' --exclude-mail --github-token ${{ secrets.GITHUB_TOKEN }} './**/*.md' './**/*.html' './**/*.yml' './**/*.yaml' './**/*.py' './**/*.ipynb'
|
||||
command: lychee --accept 429,999 --exclude-loopback --exclude 'https?://(www\.)?(twitter\.com|instagram\.com|url\.com)' --exclude-path '**/ci.yaml' --exclude-mail --github-token ${{ secrets.GITHUB_TOKEN }} './**/*.md' './**/*.html' './**/*.yml' './**/*.yaml' './**/*.py' './**/*.ipynb'
|
||||
|
1
.gitignore
vendored
1
.gitignore
vendored
@ -107,6 +107,7 @@ celerybeat.pid
|
||||
# Environments
|
||||
.env
|
||||
.venv
|
||||
.idea
|
||||
env/
|
||||
venv/
|
||||
ENV/
|
||||
|
@ -1,7 +1,88 @@
|
||||
---
|
||||
comments: true
|
||||
description: Learn about the Argoverse dataset, a rich dataset designed to support research in autonomous driving tasks such as 3D tracking, motion forecasting, and stereo depth estimation.
|
||||
---
|
||||
|
||||
# 🚧 Page Under Construction ⚒
|
||||
# Argoverse Dataset
|
||||
|
||||
This page is currently under construction!️ 👷Please check back later for updates. 😃🔜
|
||||
The [Argoverse](https://www.argoverse.org/) dataset is a collection of data designed to support research in autonomous driving tasks, such as 3D tracking, motion forecasting, and stereo depth estimation. Developed by Argo AI, the dataset provides a wide range of high-quality sensor data, including high-resolution images, LiDAR point clouds, and map data.
|
||||
|
||||
## Key Features
|
||||
|
||||
- Argoverse contains over 290K labeled 3D object tracks and 5 million object instances across 1,263 distinct scenes.
|
||||
- The dataset includes high-resolution camera images, LiDAR point clouds, and richly annotated HD maps.
|
||||
- Annotations include 3D bounding boxes for objects, object tracks, and trajectory information.
|
||||
- Argoverse provides multiple subsets for different tasks, such as 3D tracking, motion forecasting, and stereo depth estimation.
|
||||
|
||||
## Dataset Structure
|
||||
|
||||
The Argoverse dataset is organized into three main subsets:
|
||||
|
||||
1. **Argoverse 3D Tracking**: This subset contains 113 scenes with over 290K labeled 3D object tracks, focusing on 3D object tracking tasks. It includes LiDAR point clouds, camera images, and sensor calibration information.
|
||||
2. **Argoverse Motion Forecasting**: This subset consists of 324K vehicle trajectories collected from 60 hours of driving data, suitable for motion forecasting tasks.
|
||||
3. **Argoverse Stereo Depth Estimation**: This subset is designed for stereo depth estimation tasks and includes over 10K stereo image pairs with corresponding LiDAR point clouds for ground truth depth estimation.
|
||||
|
||||
## Applications
|
||||
|
||||
The Argoverse dataset is widely used for training and evaluating deep learning models in autonomous driving tasks such as 3D object tracking, motion forecasting, and stereo depth estimation. The dataset's diverse set of sensor data, object annotations, and map information make it a valuable resource for researchers and practitioners in the field of autonomous driving.
|
||||
|
||||
## Dataset YAML
|
||||
|
||||
A YAML (Yet Another Markup Language) file is used to define the dataset configuration. It contains information about the dataset's paths, classes, and other relevant information. For the case of the Argoverse dataset, the `Argoverse.yaml` file is maintained at [https://github.com/ultralytics/ultralytics/blob/main/ultralytics/datasets/Argoverse.yaml](https://github.com/ultralytics/ultralytics/blob/main/ultralytics/datasets/Argoverse.yaml).
|
||||
|
||||
!!! example "ultralytics/datasets/Argoverse.yaml"
|
||||
|
||||
```yaml
|
||||
--8<-- "ultralytics/datasets/Argoverse.yaml"
|
||||
```
|
||||
|
||||
## Usage
|
||||
|
||||
To train a YOLOv8n model on the Argoverse dataset for 100 epochs with an image size of 640, you can use the following code snippets. For a comprehensive list of available arguments, refer to the model [Training](../../modes/train.md) page.
|
||||
|
||||
!!! example "Train Example"
|
||||
|
||||
=== "Python"
|
||||
|
||||
```python
|
||||
from ultralytics import YOLO
|
||||
|
||||
# Load a model
|
||||
model = YOLO('yolov8n.pt') # load a pretrained model (recommended for training)
|
||||
|
||||
# Train the model
|
||||
model.train(data='Argoverse.yaml', epochs=100, imgsz=640)
|
||||
```
|
||||
|
||||
=== "CLI"
|
||||
|
||||
```bash
|
||||
# Start training from a pretrained *.pt model
|
||||
yolo detect train data=Argoverse.yaml model=yolov8n.pt epochs=100 imgsz=640
|
||||
```
|
||||
|
||||
## Sample Data and Annotations
|
||||
|
||||
The Argoverse dataset contains a diverse set of sensor data, including camera images, LiDAR point clouds, and HD map information, providing rich context for autonomous driving tasks. Here are some examples of data from the dataset, along with their corresponding annotations:
|
||||
|
||||
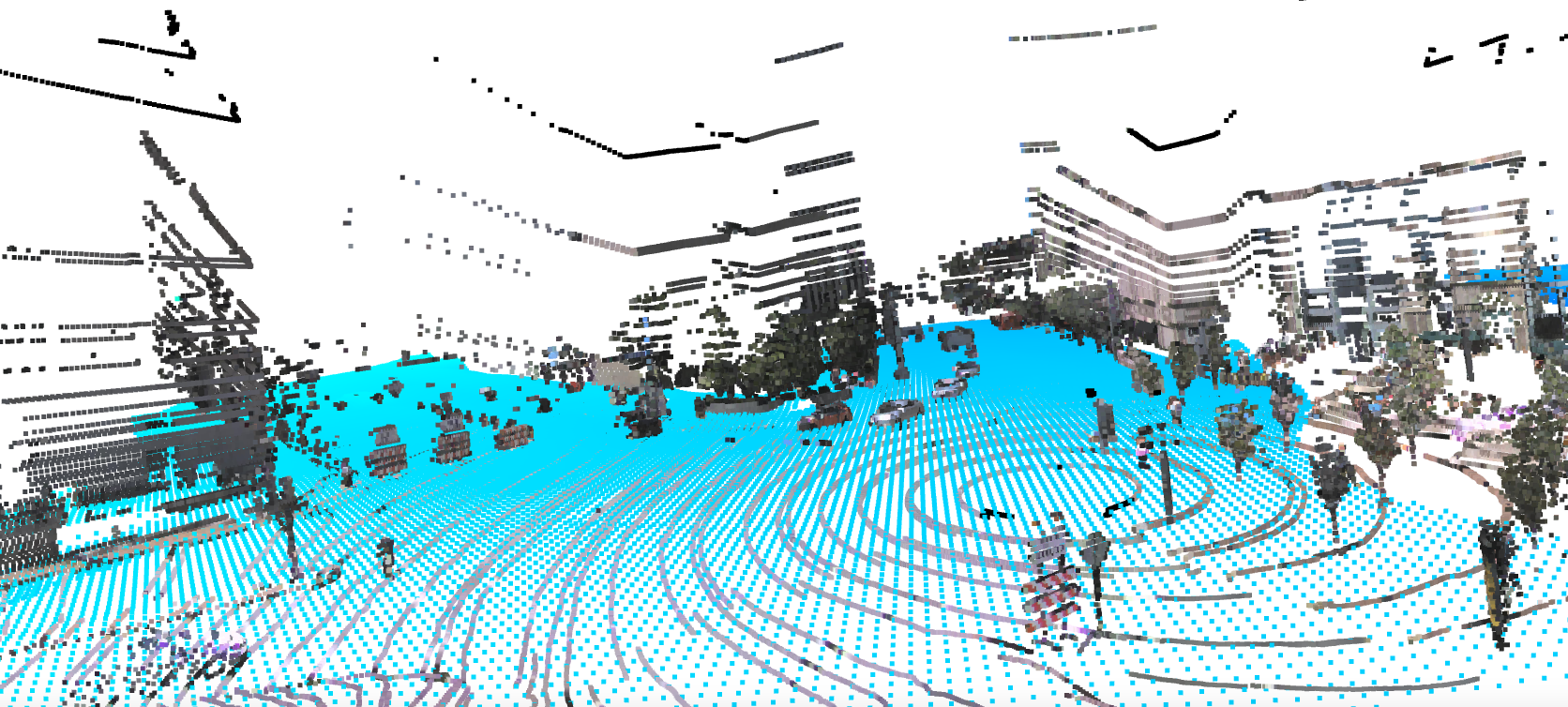
|
||||
|
||||
- **Argoverse 3D Tracking**: This image demonstrates an example of 3D object tracking, where objects are annotated with 3D bounding boxes. The dataset provides LiDAR point clouds and camera images to facilitate the development of models for this task.
|
||||
|
||||
The example showcases the variety and complexity of the data in the Argoverse dataset and highlights the importance of high-quality sensor data for autonomous driving tasks.
|
||||
|
||||
## Citations and Acknowledgments
|
||||
|
||||
If you use the Argoverse dataset in your research or development work, please cite the following paper:
|
||||
|
||||
```bibtex
|
||||
@inproceedings{chang2019argoverse,
|
||||
title={Argoverse: 3D Tracking and Forecasting with Rich Maps},
|
||||
author={Chang, Ming-Fang and Lambert, John and Sangkloy, Patsorn and Singh, Jagjeet and Bak, Slawomir and Hartnett, Andrew and Wang, Dequan and Carr, Peter and Lucey, Simon and Ramanan, Deva and others},
|
||||
booktitle={Proceedings of the IEEE/CVF Conference on Computer Vision and Pattern Recognition},
|
||||
pages={8748--8757},
|
||||
year={2019}
|
||||
}
|
||||
```
|
||||
|
||||
We would like to acknowledge Argo AI for creating and maintaining the Argoverse dataset as a valuable resource for the autonomous driving research community. For more information about the Argoverse dataset and its creators, visit the [Argoverse dataset website](https://www.argoverse.org/).
|
@ -1,7 +1,86 @@
|
||||
---
|
||||
comments: true
|
||||
description: Learn about the Global Wheat Head Dataset, aimed at supporting the development of accurate wheat head models for applications in wheat phenotyping and crop management.
|
||||
---
|
||||
|
||||
# 🚧 Page Under Construction ⚒
|
||||
# Global Wheat Head Dataset
|
||||
|
||||
This page is currently under construction!️ 👷Please check back later for updates. 😃🔜
|
||||
The [Global Wheat Head Dataset](http://www.global-wheat.com/) is a collection of images designed to support the development of accurate wheat head detection models for applications in wheat phenotyping and crop management. Wheat heads, also known as spikes, are the grain-bearing parts of the wheat plant. Accurate estimation of wheat head density and size is essential for assessing crop health, maturity, and yield potential. The dataset, created by a collaboration of nine research institutes from seven countries, covers multiple growing regions to ensure models generalize well across different environments.
|
||||
|
||||
## Key Features
|
||||
|
||||
- The dataset contains over 3,000 training images from Europe (France, UK, Switzerland) and North America (Canada).
|
||||
- It includes approximately 1,000 test images from Australia, Japan, and China.
|
||||
- Images are outdoor field images, capturing the natural variability in wheat head appearances.
|
||||
- Annotations include wheat head bounding boxes to support object detection tasks.
|
||||
|
||||
## Dataset Structure
|
||||
|
||||
The Global Wheat Head Dataset is organized into two main subsets:
|
||||
|
||||
1. **Training Set**: This subset contains over 3,000 images from Europe and North America. The images are labeled with wheat head bounding boxes, providing ground truth for training object detection models.
|
||||
2. **Test Set**: This subset consists of approximately 1,000 images from Australia, Japan, and China. These images are used for evaluating the performance of trained models on unseen genotypes, environments, and observational conditions.
|
||||
|
||||
## Applications
|
||||
|
||||
The Global Wheat Head Dataset is widely used for training and evaluating deep learning models in wheat head detection tasks. The dataset's diverse set of images, capturing a wide range of appearances, environments, and conditions, make it a valuable resource for researchers and practitioners in the field of plant phenotyping and crop management.
|
||||
|
||||
## Dataset YAML
|
||||
|
||||
A YAML (Yet Another Markup Language) file is used to define the dataset configuration. It contains information about the dataset's paths, classes, and other relevant information. For the case of the Global Wheat Head Dataset, the `GlobalWheat2020.yaml` file is maintained at [https://github.com/ultralytics/ultralytics/blob/main/ultralytics/datasets/GlobalWheat2020.yaml](https://github.com/ultralytics/ultralytics/blob/main/ultralytics/datasets/GlobalWheat2020.yaml).
|
||||
|
||||
!!! example "ultralytics/datasets/GlobalWheat2020.yaml"
|
||||
|
||||
```yaml
|
||||
--8<-- "ultralytics/datasets/GlobalWheat2020.yaml"
|
||||
```
|
||||
|
||||
## Usage
|
||||
|
||||
To train a YOLOv8n model on the Global Wheat Head Dataset for 100 epochs with an image size of 640, you can use the following code snippets. For a comprehensive list of available arguments, refer to the model [Training](../../modes/train.md) page.
|
||||
|
||||
!!! example "Train Example"
|
||||
|
||||
=== "Python"
|
||||
|
||||
```python
|
||||
from ultralytics import YOLO
|
||||
|
||||
# Load a model
|
||||
model = YOLO('yolov8n.pt') # load a pretrained model (recommended for training)
|
||||
|
||||
# Train the model
|
||||
model.train(data='GlobalWheat2020.yaml', epochs=100, imgsz=640)
|
||||
```
|
||||
|
||||
=== "CLI"
|
||||
|
||||
```bash
|
||||
# Start training from a pretrained *.pt model
|
||||
yolo detect train data=GlobalWheat2020.yaml model=yolov8n.pt epochs=100 imgsz=640
|
||||
```
|
||||
|
||||
## Sample Data and Annotations
|
||||
|
||||
The Global Wheat Head Dataset contains a diverse set of outdoor field images, capturing the natural variability in wheat head appearances, environments, and conditions. Here are some examples of data from the dataset, along with their corresponding annotations:
|
||||
|
||||
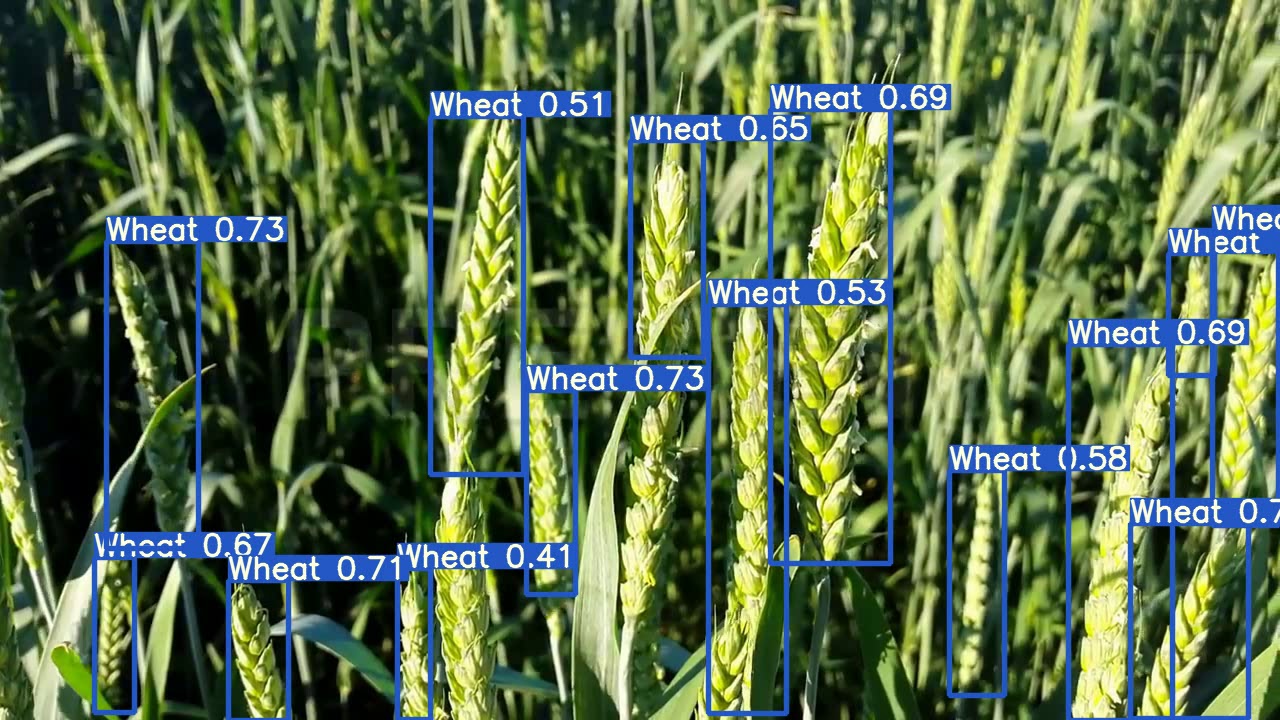
|
||||
|
||||
- **Wheat Head Detection**: This image demonstrates an example of wheat head detection, where wheat heads are annotated with bounding boxes. The dataset provides a variety of images to facilitate the development of models for this task.
|
||||
|
||||
The example showcases the variety and complexity of the data in the Global Wheat Head Dataset and highlights the importance of accurate wheat head detection for applications in wheat phenotyping and crop management.
|
||||
|
||||
## Citations and Acknowledgments
|
||||
|
||||
If you use the Global Wheat Head Dataset in your research or development work, please cite the following paper:
|
||||
|
||||
```bibtex
|
||||
@article{david2020global,
|
||||
title={Global Wheat Head Detection (GWHD) Dataset: A Large and Diverse Dataset of High-Resolution RGB-Labelled Images to Develop and Benchmark Wheat Head Detection Methods},
|
||||
author={David, Etienne and Madec, Simon and Sadeghi-Tehran, Pouria and Aasen, Helge and Zheng, Bangyou and Liu, Shouyang and Kirchgessner, Norbert and Ishikawa, Goro and Nagasawa, Koichi and Badhon, Minhajul and others},
|
||||
journal={arXiv preprint arXiv:2005.02162},
|
||||
year={2020}
|
||||
}
|
||||
```
|
||||
|
||||
We would like to acknowledge the researchers and institutions that contributed to the creation and maintenance of the Global Wheat Head Dataset as a valuable resource for the plant phenotyping and crop management research community. For more information about the dataset and its creators, visit the [Global Wheat Head Dataset website](http://www.global-wheat.com/).
|
@ -45,7 +45,7 @@ train: <path-to-training-images>
|
||||
val: <path-to-validation-images>
|
||||
|
||||
nc: <number-of-classes>
|
||||
names: [<class-1>, <class-2>, ..., <class-n>]
|
||||
names: [ <class-1>, <class-2>, ..., <class-n> ]
|
||||
|
||||
```
|
||||
|
||||
@ -72,7 +72,7 @@ train: data/train/
|
||||
val: data/val/
|
||||
|
||||
nc: 2
|
||||
names: ['person', 'car']
|
||||
names: [ 'person', 'car' ]
|
||||
```
|
||||
|
||||
## Usage
|
||||
@ -107,4 +107,30 @@ names: ['person', 'car']
|
||||
from ultralytics.yolo.data.converter import convert_coco
|
||||
|
||||
convert_coco(labels_dir='../coco/annotations/', use_segments=True)
|
||||
```
|
||||
```
|
||||
|
||||
## Auto-Annotation
|
||||
|
||||
Auto-annotation is an essential feature that allows you to generate a segmentation dataset using a pre-trained detection model. It enables you to quickly and accurately annotate a large number of images without the need for manual labeling, saving time and effort.
|
||||
|
||||
### Generate Segmentation Dataset Using a Detection Model
|
||||
|
||||
To auto-annotate your dataset using the Ultralytics framework, you can use the `auto_annotate` function as shown below:
|
||||
|
||||
```python
|
||||
from ultralytics.yolo.data import auto_annotate
|
||||
|
||||
auto_annotate(data="path/to/images", det_model="yolov8x.pt", sam_model='sam_b.pt')
|
||||
```
|
||||
|
||||
| Argument | Type | Description | Default |
|
||||
|------------|---------------------|---------------------------------------------------------------------------------------------------------|--------------|
|
||||
| data | str | Path to a folder containing images to be annotated. | |
|
||||
| det_model | str, optional | Pre-trained YOLO detection model. Defaults to 'yolov8x.pt'. | 'yolov8x.pt' |
|
||||
| sam_model | str, optional | Pre-trained SAM segmentation model. Defaults to 'sam_b.pt'. | 'sam_b.pt' |
|
||||
| device | str, optional | Device to run the models on. Defaults to an empty string (CPU or GPU, if available). | |
|
||||
| output_dir | str, None, optional | Directory to save the annotated results. Defaults to a 'labels' folder in the same directory as 'data'. | None |
|
||||
|
||||
The `auto_annotate` function takes the path to your images, along with optional arguments for specifying the pre-trained detection and [SAM segmentation models](https://docs.ultralytics.com/models/sam), the device to run the models on, and the output directory for saving the annotated results.
|
||||
|
||||
By leveraging the power of pre-trained models, auto-annotation can significantly reduce the time and effort required for creating high-quality segmentation datasets. This feature is particularly useful for researchers and developers working with large image collections, as it allows them to focus on model development and evaluation rather than manual annotation.
|
@ -49,4 +49,19 @@ model.predict("path/to/image.jpg") # predict
|
||||
| Validation | :heavy_check_mark: |
|
||||
| Training | :x: (Coming soon) |
|
||||
|
||||
For more information about the RT-DETR model, please refer to the [original paper](https://arxiv.org/abs/2304.08069) and the [PaddleDetection repository](https://github.com/PaddlePaddle/PaddleDetection).
|
||||
# Citations and Acknowledgements
|
||||
|
||||
If you use RT-DETR in your research or development work, please cite the [original paper](https://arxiv.org/abs/2304.08069):
|
||||
|
||||
```bibtex
|
||||
@misc{lv2023detrs,
|
||||
title={DETRs Beat YOLOs on Real-time Object Detection},
|
||||
author={Wenyu Lv and Shangliang Xu and Yian Zhao and Guanzhong Wang and Jinman Wei and Cheng Cui and Yuning Du and Qingqing Dang and Yi Liu},
|
||||
year={2023},
|
||||
eprint={2304.08069},
|
||||
archivePrefix={arXiv},
|
||||
primaryClass={cs.CV}
|
||||
}
|
||||
```
|
||||
|
||||
We would like to acknowledge Baidu's [PaddlePaddle]((https://github.com/PaddlePaddle/PaddleDetection)) team for creating and maintaining this valuable resource for the computer vision community.
|
||||
|
@ -9,7 +9,8 @@ description: Learn about the Segment Anything Model (SAM) and how it provides pr
|
||||
|
||||
The Segment Anything Model (SAM) is a groundbreaking image segmentation model that enables promptable segmentation with real-time performance. It forms the foundation for the Segment Anything project, which introduces a new task, model, and dataset for image segmentation. SAM is designed to be promptable, allowing it to transfer zero-shot to new image distributions and tasks. The model is trained on the [SA-1B dataset](https://ai.facebook.com/datasets/segment-anything/), which contains over 1 billion masks on 11 million licensed and privacy-respecting images. SAM has demonstrated impressive zero-shot performance, often surpassing prior fully supervised results.
|
||||
|
||||

|
||||
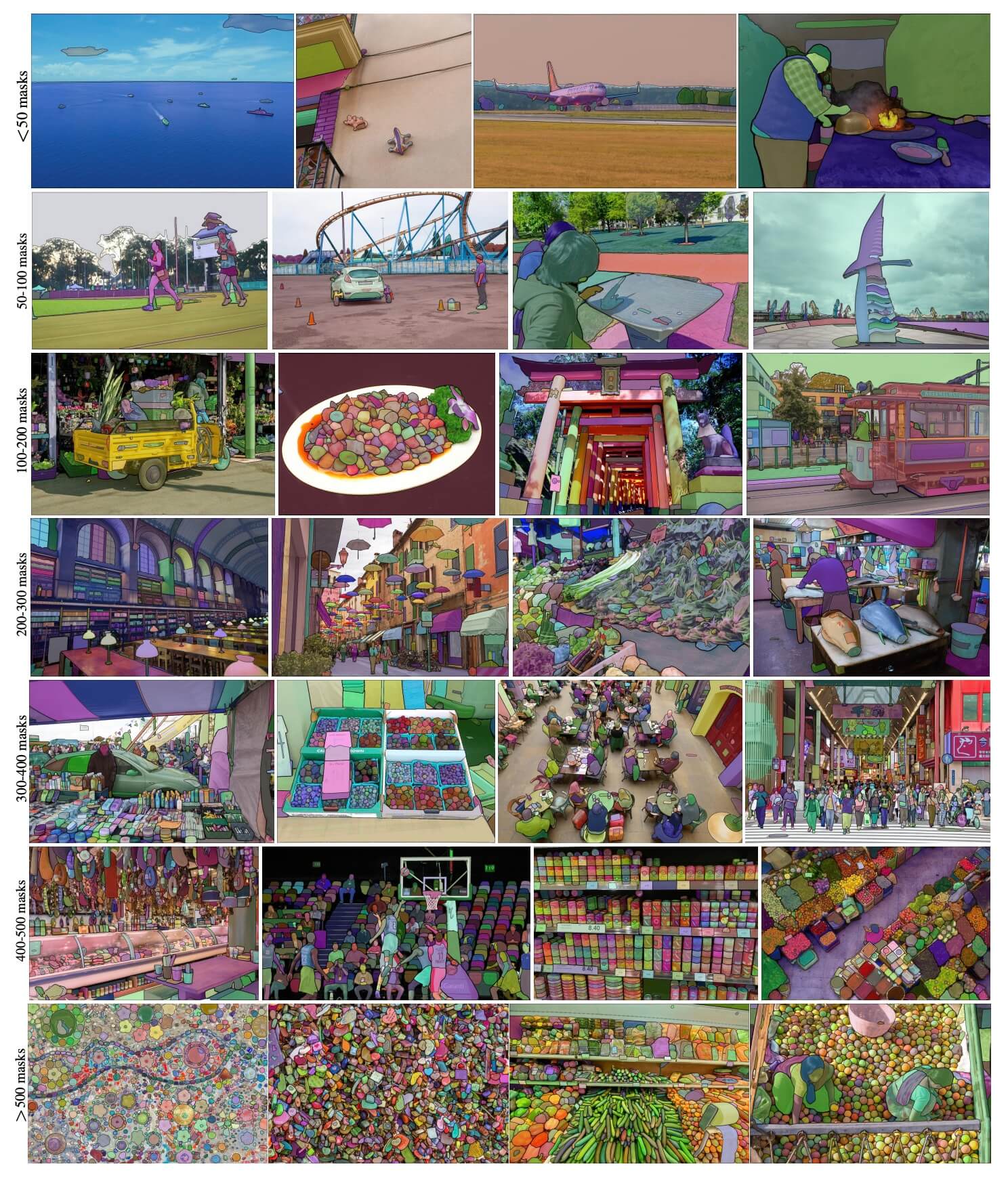
|
||||
Example images with overlaid masks from our newly introduced dataset, SA-1B. SA-1B contains 11M diverse, high-resolution, licensed, and privacy protecting images and 1.1B high-quality segmentation masks. These masks were annotated fully automatically by SAM, and as verified by human ratings and numerous experiments, are of high quality and diversity. Images are grouped by number of masks per image for visualization (there are ∼100 masks per image on average).
|
||||
|
||||
## Key Features
|
||||
|
||||
@ -47,7 +48,33 @@ model.predict('path/to/image.jpg') # predict
|
||||
| Validation | :x: |
|
||||
| Training | :x: |
|
||||
|
||||
# Citations and Acknowledgements
|
||||
## Auto-Annotation
|
||||
|
||||
Auto-annotation is an essential feature that allows you to generate a [segmentation dataset](https://docs.ultralytics.com/datasets/segment) using a pre-trained detection model. It enables you to quickly and accurately annotate a large number of images without the need for manual labeling, saving time and effort.
|
||||
|
||||
### Generate Segmentation Dataset Using a Detection Model
|
||||
|
||||
To auto-annotate your dataset using the Ultralytics framework, you can use the `auto_annotate` function as shown below:
|
||||
|
||||
```python
|
||||
from ultralytics.yolo.data import auto_annotate
|
||||
|
||||
auto_annotate(data="path/to/images", det_model="yolov8x.pt", sam_model='sam_b.pt')
|
||||
```
|
||||
|
||||
| Argument | Type | Description | Default |
|
||||
|------------|---------------------|---------------------------------------------------------------------------------------------------------|--------------|
|
||||
| data | str | Path to a folder containing images to be annotated. | |
|
||||
| det_model | str, optional | Pre-trained YOLO detection model. Defaults to 'yolov8x.pt'. | 'yolov8x.pt' |
|
||||
| sam_model | str, optional | Pre-trained SAM segmentation model. Defaults to 'sam_b.pt'. | 'sam_b.pt' |
|
||||
| device | str, optional | Device to run the models on. Defaults to an empty string (CPU or GPU, if available). | |
|
||||
| output_dir | str, None, optional | Directory to save the annotated results. Defaults to a 'labels' folder in the same directory as 'data'. | None |
|
||||
|
||||
The `auto_annotate` function takes the path to your images, along with optional arguments for specifying the pre-trained detection and SAM segmentation models, the device to run the models on, and the output directory for saving the annotated results.
|
||||
|
||||
By leveraging the power of pre-trained models, auto-annotation can significantly reduce the time and effort required for creating high-quality segmentation datasets. This feature is particularly useful for researchers and developers working with large image collections, as it allows them to focus on model development and evaluation rather than manual annotation.
|
||||
|
||||
## Citations and Acknowledgements
|
||||
|
||||
If you use SAM in your research or development work, please cite the following paper:
|
||||
|
||||
|
@ -49,7 +49,7 @@ whether each source can be used in streaming mode with `stream=True` ✅ and an
|
||||
| URL | `'https://ultralytics.com/images/bus.jpg'` | `str` | |
|
||||
| screenshot | `'screen'` | `str` | |
|
||||
| PIL | `Image.open('im.jpg')` | `PIL.Image` | HWC, RGB |
|
||||
| OpenCV | `cv2.imread('im.jpg')[:,:,::-1]` | `np.ndarray` | HWC, BGR to RGB |
|
||||
| OpenCV | `cv2.imread('im.jpg')` | `np.ndarray` | HWC, BGR |
|
||||
| numpy | `np.zeros((640,1280,3))` | `np.ndarray` | HWC |
|
||||
| torch | `torch.zeros(16,3,320,640)` | `torch.Tensor` | BCHW, RGB |
|
||||
| CSV | `'sources.csv'` | `str`, `Path` | RTSP, RTMP, HTTP |
|
||||
@ -279,4 +279,4 @@ Here's a Python script using OpenCV (cv2) and YOLOv8 to run inference on video f
|
||||
# Release the video capture object and close the display window
|
||||
cap.release()
|
||||
cv2.destroyAllWindows()
|
||||
```
|
||||
```
|
||||
|
@ -13,7 +13,7 @@ torchvision>=0.8.1
|
||||
tqdm>=4.64.0
|
||||
|
||||
# Logging -------------------------------------
|
||||
# tensorboard>=2.4.1
|
||||
# tensorboard>=2.13.0
|
||||
# clearml
|
||||
# comet
|
||||
|
||||
|
@ -1,6 +1,6 @@
|
||||
# Ultralytics YOLO 🚀, AGPL-3.0 license
|
||||
|
||||
__version__ = '8.0.99'
|
||||
__version__ = '8.0.100'
|
||||
|
||||
from ultralytics.hub import start
|
||||
from ultralytics.vit.rtdetr import RTDETR
|
||||
|
@ -177,13 +177,13 @@ class C2f(nn.Module):
|
||||
self.m = nn.ModuleList(Bottleneck(self.c, self.c, shortcut, g, k=((3, 3), (3, 3)), e=1.0) for _ in range(n))
|
||||
|
||||
def forward(self, x):
|
||||
"""Forward pass of a YOLOv5 CSPDarknet backbone layer."""
|
||||
"""Forward pass through C2f layer."""
|
||||
y = list(self.cv1(x).chunk(2, 1))
|
||||
y.extend(m(y[-1]) for m in self.m)
|
||||
return self.cv2(torch.cat(y, 1))
|
||||
|
||||
def forward_split(self, x):
|
||||
"""Applies spatial attention to module's input."""
|
||||
"""Forward pass using split() instead of chunk()."""
|
||||
y = list(self.cv1(x).split((self.c, self.c), 1))
|
||||
y.extend(m(y[-1]) for m in self.m)
|
||||
return self.cv2(torch.cat(y, 1))
|
||||
|
@ -126,7 +126,7 @@ class BaseModel(nn.Module):
|
||||
bn = tuple(v for k, v in nn.__dict__.items() if 'Norm' in k) # normalization layers, i.e. BatchNorm2d()
|
||||
return sum(isinstance(v, bn) for v in self.modules()) < thresh # True if < 'thresh' BatchNorm layers in model
|
||||
|
||||
def info(self, verbose=True, imgsz=640):
|
||||
def info(self, detailed=False, verbose=True, imgsz=640):
|
||||
"""
|
||||
Prints model information
|
||||
|
||||
@ -134,7 +134,7 @@ class BaseModel(nn.Module):
|
||||
verbose (bool): if True, prints out the model information. Defaults to False
|
||||
imgsz (int): the size of the image that the model will be trained on. Defaults to 640
|
||||
"""
|
||||
model_info(self, verbose=verbose, imgsz=imgsz)
|
||||
return model_info(self, detailed=detailed, verbose=verbose, imgsz=imgsz)
|
||||
|
||||
def _apply(self, fn):
|
||||
"""
|
||||
|
@ -181,7 +181,7 @@ class BYTETracker:
|
||||
def update(self, results, img=None):
|
||||
"""Updates object tracker with new detections and returns tracked object bounding boxes."""
|
||||
self.frame_id += 1
|
||||
activated_starcks = []
|
||||
activated_stracks = []
|
||||
refind_stracks = []
|
||||
lost_stracks = []
|
||||
removed_stracks = []
|
||||
@ -230,7 +230,7 @@ class BYTETracker:
|
||||
det = detections[idet]
|
||||
if track.state == TrackState.Tracked:
|
||||
track.update(det, self.frame_id)
|
||||
activated_starcks.append(track)
|
||||
activated_stracks.append(track)
|
||||
else:
|
||||
track.re_activate(det, self.frame_id, new_id=False)
|
||||
refind_stracks.append(track)
|
||||
@ -246,7 +246,7 @@ class BYTETracker:
|
||||
det = detections_second[idet]
|
||||
if track.state == TrackState.Tracked:
|
||||
track.update(det, self.frame_id)
|
||||
activated_starcks.append(track)
|
||||
activated_stracks.append(track)
|
||||
else:
|
||||
track.re_activate(det, self.frame_id, new_id=False)
|
||||
refind_stracks.append(track)
|
||||
@ -262,7 +262,7 @@ class BYTETracker:
|
||||
matches, u_unconfirmed, u_detection = matching.linear_assignment(dists, thresh=0.7)
|
||||
for itracked, idet in matches:
|
||||
unconfirmed[itracked].update(detections[idet], self.frame_id)
|
||||
activated_starcks.append(unconfirmed[itracked])
|
||||
activated_stracks.append(unconfirmed[itracked])
|
||||
for it in u_unconfirmed:
|
||||
track = unconfirmed[it]
|
||||
track.mark_removed()
|
||||
@ -273,7 +273,7 @@ class BYTETracker:
|
||||
if track.score < self.args.new_track_thresh:
|
||||
continue
|
||||
track.activate(self.kalman_filter, self.frame_id)
|
||||
activated_starcks.append(track)
|
||||
activated_stracks.append(track)
|
||||
# Step 5: Update state
|
||||
for track in self.lost_stracks:
|
||||
if self.frame_id - track.end_frame > self.max_time_lost:
|
||||
@ -281,7 +281,7 @@ class BYTETracker:
|
||||
removed_stracks.append(track)
|
||||
|
||||
self.tracked_stracks = [t for t in self.tracked_stracks if t.state == TrackState.Tracked]
|
||||
self.tracked_stracks = self.joint_stracks(self.tracked_stracks, activated_starcks)
|
||||
self.tracked_stracks = self.joint_stracks(self.tracked_stracks, activated_stracks)
|
||||
self.tracked_stracks = self.joint_stracks(self.tracked_stracks, refind_stracks)
|
||||
self.lost_stracks = self.sub_stracks(self.lost_stracks, self.tracked_stracks)
|
||||
self.lost_stracks.extend(lost_stracks)
|
||||
|
@ -8,7 +8,7 @@ from pathlib import Path
|
||||
from ultralytics.nn.tasks import DetectionModel, attempt_load_one_weight, yaml_model_load
|
||||
from ultralytics.yolo.cfg import get_cfg
|
||||
from ultralytics.yolo.engine.exporter import Exporter
|
||||
from ultralytics.yolo.utils import DEFAULT_CFG, DEFAULT_CFG_DICT
|
||||
from ultralytics.yolo.utils import DEFAULT_CFG, DEFAULT_CFG_DICT, LOGGER, ROOT, is_git_dir
|
||||
from ultralytics.yolo.utils.checks import check_imgsz
|
||||
from ultralytics.yolo.utils.torch_utils import model_info
|
||||
|
||||
@ -47,7 +47,7 @@ class RTDETR:
|
||||
self.task = self.model.args['task']
|
||||
|
||||
@smart_inference_mode()
|
||||
def predict(self, source, stream=False, **kwargs):
|
||||
def predict(self, source=None, stream=False, **kwargs):
|
||||
"""
|
||||
Perform prediction using the YOLO model.
|
||||
|
||||
@ -61,6 +61,9 @@ class RTDETR:
|
||||
Returns:
|
||||
(List[ultralytics.yolo.engine.results.Results]): The prediction results.
|
||||
"""
|
||||
if source is None:
|
||||
source = ROOT / 'assets' if is_git_dir() else 'https://ultralytics.com/images/bus.jpg'
|
||||
LOGGER.warning(f"WARNING ⚠️ 'source' is missing. Using 'source={source}'.")
|
||||
overrides = dict(conf=0.25, task='detect', mode='predict')
|
||||
overrides.update(kwargs) # prefer kwargs
|
||||
if not self.predictor:
|
||||
|
@ -114,7 +114,11 @@ sam_model_map = {
|
||||
|
||||
def build_sam(ckpt='sam_b.pt'):
|
||||
"""Build a SAM model specified by ckpt."""
|
||||
model_builder = sam_model_map.get(ckpt)
|
||||
model_builder = None
|
||||
for k in sam_model_map.keys():
|
||||
if ckpt.endswith(k):
|
||||
model_builder = sam_model_map.get(k)
|
||||
|
||||
if not model_builder:
|
||||
raise FileNotFoundError(f'{ckpt} is not a supported sam model. Available models are: \n {sam_model_map.keys()}')
|
||||
|
||||
|
@ -9,7 +9,7 @@ from .predict import Predictor
|
||||
class SAM:
|
||||
|
||||
def __init__(self, model='sam_b.pt') -> None:
|
||||
if model and not (model.endswith('.pt') or model.endswith('.pth')):
|
||||
if model and not model.endswith('.pt') and not model.endswith('.pth'):
|
||||
# Should raise AssertionError instead?
|
||||
raise NotImplementedError('Segment anything prediction requires pre-trained checkpoint')
|
||||
self.model = build_sam(model)
|
||||
|
@ -115,30 +115,42 @@ class BaseMixTransform:
|
||||
|
||||
|
||||
class Mosaic(BaseMixTransform):
|
||||
"""Mosaic augmentation.
|
||||
Args:
|
||||
imgsz (Sequence[int]): Image size after mosaic pipeline of single
|
||||
image. The shape order should be (height, width).
|
||||
Default to (640, 640).
|
||||
"""
|
||||
Mosaic augmentation.
|
||||
|
||||
This class performs mosaic augmentation by combining multiple (4 or 9) images into a single mosaic image.
|
||||
The augmentation is applied to a dataset with a given probability.
|
||||
|
||||
Attributes:
|
||||
dataset: The dataset on which the mosaic augmentation is applied.
|
||||
imgsz (int, optional): Image size (height and width) after mosaic pipeline of a single image. Default to 640.
|
||||
p (float, optional): Probability of applying the mosaic augmentation. Must be in the range 0-1. Default to 1.0.
|
||||
n (int, optional): The grid size, either 4 (for 2x2) or 9 (for 3x3).
|
||||
"""
|
||||
|
||||
def __init__(self, dataset, imgsz=640, p=1.0, border=(0, 0)):
|
||||
def __init__(self, dataset, imgsz=640, p=1.0, n=9):
|
||||
"""Initializes the object with a dataset, image size, probability, and border."""
|
||||
assert 0 <= p <= 1.0, 'The probability should be in range [0, 1]. ' f'got {p}.'
|
||||
assert 0 <= p <= 1.0, f'The probability should be in range [0, 1], but got {p}.'
|
||||
assert n in (4, 9), 'grid must be equal to 4 or 9.'
|
||||
super().__init__(dataset=dataset, p=p)
|
||||
self.dataset = dataset
|
||||
self.imgsz = imgsz
|
||||
self.border = border
|
||||
self.border = [-imgsz // 2, -imgsz // 2] if n == 4 else [-imgsz, -imgsz]
|
||||
self.n = n
|
||||
|
||||
def get_indexes(self):
|
||||
"""Return a list of 3 random indexes from the dataset."""
|
||||
return [random.randint(0, len(self.dataset) - 1) for _ in range(3)]
|
||||
"""Return a list of random indexes from the dataset."""
|
||||
return [random.randint(0, len(self.dataset) - 1) for _ in range(self.n - 1)]
|
||||
|
||||
def _mix_transform(self, labels):
|
||||
"""Apply mixup transformation to the input image and labels."""
|
||||
assert labels.get('rect_shape', None) is None, 'rect and mosaic are mutually exclusive.'
|
||||
assert len(labels.get('mix_labels', [])), 'There are no other images for mosaic augment.'
|
||||
return self._mosaic4(labels) if self.n == 4 else self._mosaic9(labels)
|
||||
|
||||
def _mosaic4(self, labels):
|
||||
"""Create a 2x2 image mosaic."""
|
||||
mosaic_labels = []
|
||||
assert labels.get('rect_shape', None) is None, 'rect and mosaic is exclusive.'
|
||||
assert len(labels.get('mix_labels', [])) > 0, 'There are no other images for mosaic augment.'
|
||||
s = self.imgsz
|
||||
yc, xc = (int(random.uniform(-x, 2 * s + x)) for x in self.border) # mosaic center x, y
|
||||
for i in range(4):
|
||||
@ -172,7 +184,54 @@ class Mosaic(BaseMixTransform):
|
||||
final_labels['img'] = img4
|
||||
return final_labels
|
||||
|
||||
def _update_labels(self, labels, padw, padh):
|
||||
def _mosaic9(self, labels):
|
||||
"""Create a 3x3 image mosaic."""
|
||||
mosaic_labels = []
|
||||
s = self.imgsz
|
||||
hp, wp = -1, -1 # height, width previous
|
||||
for i in range(9):
|
||||
labels_patch = labels if i == 0 else labels['mix_labels'][i - 1]
|
||||
# Load image
|
||||
img = labels_patch['img']
|
||||
h, w = labels_patch.pop('resized_shape')
|
||||
|
||||
# Place img in img9
|
||||
if i == 0: # center
|
||||
img9 = np.full((s * 3, s * 3, img.shape[2]), 114, dtype=np.uint8) # base image with 4 tiles
|
||||
h0, w0 = h, w
|
||||
c = s, s, s + w, s + h # xmin, ymin, xmax, ymax (base) coordinates
|
||||
elif i == 1: # top
|
||||
c = s, s - h, s + w, s
|
||||
elif i == 2: # top right
|
||||
c = s + wp, s - h, s + wp + w, s
|
||||
elif i == 3: # right
|
||||
c = s + w0, s, s + w0 + w, s + h
|
||||
elif i == 4: # bottom right
|
||||
c = s + w0, s + hp, s + w0 + w, s + hp + h
|
||||
elif i == 5: # bottom
|
||||
c = s + w0 - w, s + h0, s + w0, s + h0 + h
|
||||
elif i == 6: # bottom left
|
||||
c = s + w0 - wp - w, s + h0, s + w0 - wp, s + h0 + h
|
||||
elif i == 7: # left
|
||||
c = s - w, s + h0 - h, s, s + h0
|
||||
elif i == 8: # top left
|
||||
c = s - w, s + h0 - hp - h, s, s + h0 - hp
|
||||
|
||||
padw, padh = c[:2]
|
||||
x1, y1, x2, y2 = (max(x, 0) for x in c) # allocate coords
|
||||
|
||||
# Image
|
||||
img9[y1:y2, x1:x2] = img[y1 - padh:, x1 - padw:] # img9[ymin:ymax, xmin:xmax]
|
||||
hp, wp = h, w # height, width previous for next iteration
|
||||
|
||||
labels_patch = self._update_labels(labels_patch, padw, padh)
|
||||
mosaic_labels.append(labels_patch)
|
||||
final_labels = self._cat_labels(mosaic_labels)
|
||||
final_labels['img'] = img9
|
||||
return final_labels
|
||||
|
||||
@staticmethod
|
||||
def _update_labels(labels, padw, padh):
|
||||
"""Update labels."""
|
||||
nh, nw = labels['img'].shape[:2]
|
||||
labels['instances'].convert_bbox(format='xyxy')
|
||||
@ -195,8 +254,9 @@ class Mosaic(BaseMixTransform):
|
||||
'resized_shape': (self.imgsz * 2, self.imgsz * 2),
|
||||
'cls': np.concatenate(cls, 0),
|
||||
'instances': Instances.concatenate(instances, axis=0),
|
||||
'mosaic_border': self.border}
|
||||
final_labels['instances'].clip(self.imgsz * 2, self.imgsz * 2)
|
||||
'mosaic_border': self.border} # final_labels
|
||||
clip_size = self.imgsz * (2 if self.n == 4 else 3)
|
||||
final_labels['instances'].clip(clip_size, clip_size)
|
||||
return final_labels
|
||||
|
||||
|
||||
@ -695,7 +755,7 @@ class Format:
|
||||
def v8_transforms(dataset, imgsz, hyp):
|
||||
"""Convert images to a size suitable for YOLOv8 training."""
|
||||
pre_transform = Compose([
|
||||
Mosaic(dataset, imgsz=imgsz, p=hyp.mosaic, border=[-imgsz // 2, -imgsz // 2]),
|
||||
Mosaic(dataset, imgsz=imgsz, p=hyp.mosaic),
|
||||
CopyPaste(p=hyp.copy_paste),
|
||||
RandomPerspective(
|
||||
degrees=hyp.degrees,
|
||||
|
@ -201,15 +201,16 @@ class YOLO:
|
||||
self.model.load(weights)
|
||||
return self
|
||||
|
||||
def info(self, verbose=True):
|
||||
def info(self, detailed=False, verbose=True):
|
||||
"""
|
||||
Logs model info.
|
||||
|
||||
Args:
|
||||
detailed (bool): Show detailed information about model.
|
||||
verbose (bool): Controls verbosity.
|
||||
"""
|
||||
self._check_is_pytorch_model()
|
||||
self.model.info(verbose=verbose)
|
||||
return self.model.info(detailed=detailed, verbose=verbose)
|
||||
|
||||
def fuse(self):
|
||||
"""Fuse PyTorch Conv2d and BatchNorm2d layers."""
|
||||
|
@ -190,17 +190,6 @@ class BaseTrainer:
|
||||
else:
|
||||
self._do_train(world_size)
|
||||
|
||||
def _pre_caching_dataset(self):
|
||||
"""
|
||||
Caching dataset before training to avoid NCCL timeout.
|
||||
Must be done before DDP initialization.
|
||||
See https://github.com/ultralytics/ultralytics/pull/2549 for details.
|
||||
"""
|
||||
if RANK in (-1, 0):
|
||||
LOGGER.info('Pre-caching dataset to avoid NCCL timeout')
|
||||
self.get_dataloader(self.trainset, batch_size=1, rank=RANK, mode='train')
|
||||
self.get_dataloader(self.testset, batch_size=1, rank=-1, mode='val')
|
||||
|
||||
def _setup_ddp(self, world_size):
|
||||
"""Initializes and sets the DistributedDataParallel parameters for training."""
|
||||
torch.cuda.set_device(RANK)
|
||||
@ -274,7 +263,6 @@ class BaseTrainer:
|
||||
def _do_train(self, world_size=1):
|
||||
"""Train completed, evaluate and plot if specified by arguments."""
|
||||
if world_size > 1:
|
||||
self._pre_caching_dataset()
|
||||
self._setup_ddp(world_size)
|
||||
|
||||
self._setup_train(world_size)
|
||||
|
@ -233,7 +233,7 @@ def check_requirements(requirements=ROOT.parent / 'requirements.txt', exclude=()
|
||||
n += 1
|
||||
|
||||
if s and install and AUTOINSTALL: # check environment variable
|
||||
LOGGER.info(f"{prefix} YOLOv8 requirement{'s' * (n > 1)} {s}not found, attempting AutoUpdate...")
|
||||
LOGGER.info(f"{prefix} Ultralytics requirement{'s' * (n > 1)} {s}not found, attempting AutoUpdate...")
|
||||
try:
|
||||
assert is_online(), 'AutoUpdate skipped (offline)'
|
||||
LOGGER.info(subprocess.check_output(f'pip install --no-cache {s} {cmds}', shell=True).decode())
|
||||
|
@ -34,7 +34,7 @@ class BboxLoss(nn.Module):
|
||||
|
||||
def forward(self, pred_dist, pred_bboxes, anchor_points, target_bboxes, target_scores, target_scores_sum, fg_mask):
|
||||
"""IoU loss."""
|
||||
weight = torch.masked_select(target_scores.sum(-1), fg_mask).unsqueeze(-1)
|
||||
weight = target_scores.sum(-1)[fg_mask]
|
||||
iou = bbox_iou(pred_bboxes[fg_mask], target_bboxes[fg_mask], xywh=False, CIoU=True)
|
||||
loss_iou = ((1.0 - iou) * weight).sum() / target_scores_sum
|
||||
|
||||
|
@ -189,6 +189,7 @@ class TaskAlignedAssigner(nn.Module):
|
||||
for k in range(self.topk):
|
||||
# Expand topk_idxs for each value of k and add 1 at the specified positions
|
||||
count_tensor.scatter_add_(-1, topk_idxs[:, :, k:k + 1], ones)
|
||||
# count_tensor.scatter_add_(-1, topk_idxs, torch.ones_like(topk_idxs, dtype=torch.int8, device=topk_idxs.device))
|
||||
# filter invalid bboxes
|
||||
count_tensor.masked_fill_(count_tensor > 1, 0)
|
||||
|
||||
|
@ -165,14 +165,15 @@ def model_info(model, detailed=False, verbose=True, imgsz=640):
|
||||
f"{'layer':>5} {'name':>40} {'gradient':>9} {'parameters':>12} {'shape':>20} {'mu':>10} {'sigma':>10}")
|
||||
for i, (name, p) in enumerate(model.named_parameters()):
|
||||
name = name.replace('module_list.', '')
|
||||
LOGGER.info('%5g %40s %9s %12g %20s %10.3g %10.3g' %
|
||||
(i, name, p.requires_grad, p.numel(), list(p.shape), p.mean(), p.std()))
|
||||
LOGGER.info('%5g %40s %9s %12g %20s %10.3g %10.3g %10s' %
|
||||
(i, name, p.requires_grad, p.numel(), list(p.shape), p.mean(), p.std(), p.dtype))
|
||||
|
||||
flops = get_flops(model, imgsz)
|
||||
fused = ' (fused)' if model.is_fused() else ''
|
||||
fs = f', {flops:.1f} GFLOPs' if flops else ''
|
||||
m = Path(getattr(model, 'yaml_file', '') or model.yaml.get('yaml_file', '')).stem.replace('yolo', 'YOLO') or 'Model'
|
||||
LOGGER.info(f'{m} summary{fused}: {len(list(model.modules()))} layers, {n_p} parameters, {n_g} gradients{fs}')
|
||||
return n_p, flops
|
||||
|
||||
|
||||
def get_num_params(model):
|
||||
|
Reference in New Issue
Block a user