Add Dockerfiles and update Docs README (#124)
Co-authored-by: Ayush Chaurasia <ayush.chaurarsia@gmail.com> Co-authored-by: pre-commit-ci[bot] <66853113+pre-commit-ci[bot]@users.noreply.github.com>single_channel
parent
df4fc14c10
commit
a9b9fe7618
@ -0,0 +1,57 @@
|
|||||||
|
# YOLOv5 🚀 by Ultralytics, GPL-3.0 license
|
||||||
|
# Builds ultralytics/ultralytics:latest images on DockerHub https://hub.docker.com/r/ultralytics
|
||||||
|
|
||||||
|
name: Publish Docker Images
|
||||||
|
|
||||||
|
on:
|
||||||
|
push:
|
||||||
|
branches: [ none ] # TODO: replace with main
|
||||||
|
|
||||||
|
jobs:
|
||||||
|
docker:
|
||||||
|
if: github.repository == 'ultralytics/ultralytics'
|
||||||
|
name: Push Docker image to Docker Hub
|
||||||
|
runs-on: ubuntu-latest
|
||||||
|
steps:
|
||||||
|
- name: Checkout repo
|
||||||
|
uses: actions/checkout@v3
|
||||||
|
|
||||||
|
- name: Set up QEMU
|
||||||
|
uses: docker/setup-qemu-action@v2
|
||||||
|
|
||||||
|
- name: Set up Docker Buildx
|
||||||
|
uses: docker/setup-buildx-action@v2
|
||||||
|
|
||||||
|
- name: Login to Docker Hub
|
||||||
|
uses: docker/login-action@v2
|
||||||
|
with:
|
||||||
|
username: ${{ secrets.DOCKERHUB_USERNAME }}
|
||||||
|
password: ${{ secrets.DOCKERHUB_TOKEN }}
|
||||||
|
|
||||||
|
- name: Build and push arm64 image
|
||||||
|
uses: docker/build-push-action@v3
|
||||||
|
continue-on-error: true
|
||||||
|
with:
|
||||||
|
context: .
|
||||||
|
platforms: linux/arm64
|
||||||
|
file: docker/Dockerfile-arm64
|
||||||
|
push: true
|
||||||
|
tags: ultralytics/ultralytics:latest-arm64
|
||||||
|
|
||||||
|
- name: Build and push CPU image
|
||||||
|
uses: docker/build-push-action@v3
|
||||||
|
continue-on-error: true
|
||||||
|
with:
|
||||||
|
context: .
|
||||||
|
file: docker/Dockerfile-cpu
|
||||||
|
push: true
|
||||||
|
tags: ultralytics/ultralytics:latest-cpu
|
||||||
|
|
||||||
|
- name: Build and push GPU image
|
||||||
|
uses: docker/build-push-action@v3
|
||||||
|
continue-on-error: true
|
||||||
|
with:
|
||||||
|
context: .
|
||||||
|
file: docker/Dockerfile
|
||||||
|
push: true
|
||||||
|
tags: ultralytics/ultralytics:latest
|
@ -0,0 +1,64 @@
|
|||||||
|
# YOLOv5 🚀 by Ultralytics, GPL-3.0 license
|
||||||
|
# Builds ultralytics/ultralytics:latest image on DockerHub https://hub.docker.com/r/ultralytics/ultralytics
|
||||||
|
# Image is CUDA-optimized for YOLOv5 single/multi-GPU training and inference
|
||||||
|
|
||||||
|
# Start FROM NVIDIA PyTorch image https://ngc.nvidia.com/catalog/containers/nvidia:pytorch
|
||||||
|
FROM nvcr.io/nvidia/pytorch:22.12-py3
|
||||||
|
RUN rm -rf /opt/pytorch # remove 1.2GB dir
|
||||||
|
|
||||||
|
# Downloads to user config dir
|
||||||
|
ADD https://ultralytics.com/assets/Arial.ttf https://ultralytics.com/assets/Arial.Unicode.ttf /root/.config/Ultralytics/
|
||||||
|
|
||||||
|
# Install linux packages
|
||||||
|
RUN apt update && apt install --no-install-recommends -y zip htop screen libgl1-mesa-glx
|
||||||
|
|
||||||
|
# Create working directory
|
||||||
|
RUN mkdir -p /usr/src/ultralytics
|
||||||
|
WORKDIR /usr/src/ultralytics
|
||||||
|
|
||||||
|
# Copy contents
|
||||||
|
# COPY . /usr/src/app (issues as not a .git directory)
|
||||||
|
RUN git clone https://github.com/ultralytics/ultralytics /usr/src/ultralytics
|
||||||
|
|
||||||
|
# Install pip packages
|
||||||
|
RUN python -m pip install --upgrade pip wheel
|
||||||
|
RUN pip uninstall -y Pillow torchtext # torch torchvision
|
||||||
|
RUN pip install --no-cache ultralytics albumentations comet gsutil notebook Pillow>=9.1.0 \
|
||||||
|
'opencv-python<4.6.0.66' \
|
||||||
|
--extra-index-url https://download.pytorch.org/whl/cu113
|
||||||
|
|
||||||
|
# Set environment variables
|
||||||
|
ENV OMP_NUM_THREADS=1
|
||||||
|
|
||||||
|
|
||||||
|
# Usage Examples -------------------------------------------------------------------------------------------------------
|
||||||
|
|
||||||
|
# Build and Push
|
||||||
|
# t=ultralytics/ultralytics:latest && sudo docker build -f utils/docker/Dockerfile -t $t . && sudo docker push $t
|
||||||
|
|
||||||
|
# Pull and Run
|
||||||
|
# t=ultralytics/ultralytics:latest && sudo docker pull $t && sudo docker run -it --ipc=host --gpus all $t
|
||||||
|
|
||||||
|
# Pull and Run with local directory access
|
||||||
|
# t=ultralytics/ultralytics:latest && sudo docker pull $t && sudo docker run -it --ipc=host --gpus all -v "$(pwd)"/datasets:/usr/src/datasets $t
|
||||||
|
|
||||||
|
# Kill all
|
||||||
|
# sudo docker kill $(sudo docker ps -q)
|
||||||
|
|
||||||
|
# Kill all image-based
|
||||||
|
# sudo docker kill $(sudo docker ps -qa --filter ancestor=ultralytics/ultralytics:latest)
|
||||||
|
|
||||||
|
# DockerHub tag update
|
||||||
|
# t=ultralytics/ultralytics:latest tnew=ultralytics/ultralytics:v6.2 && sudo docker pull $t && sudo docker tag $t $tnew && sudo docker push $tnew
|
||||||
|
|
||||||
|
# Clean up
|
||||||
|
# docker system prune -a --volumes
|
||||||
|
|
||||||
|
# Update Ubuntu drivers
|
||||||
|
# https://www.maketecheasier.com/install-nvidia-drivers-ubuntu/
|
||||||
|
|
||||||
|
# DDP test
|
||||||
|
# python -m torch.distributed.run --nproc_per_node 2 --master_port 1 train.py --epochs 3
|
||||||
|
|
||||||
|
# GCP VM from Image
|
||||||
|
# docker.io/ultralytics/ultralytics:latest
|
@ -0,0 +1,45 @@
|
|||||||
|
# YOLOv5 🚀 by Ultralytics, GPL-3.0 license
|
||||||
|
# Builds ultralytics/ultralytics:latest-arm64 image on DockerHub https://hub.docker.com/r/ultralytics/ultralytics
|
||||||
|
# Image is aarch64-compatible for Apple M1 and other ARM architectures i.e. Jetson Nano and Raspberry Pi
|
||||||
|
|
||||||
|
# Start FROM Ubuntu image https://hub.docker.com/_/ubuntu
|
||||||
|
FROM arm64v8/ubuntu:20.04
|
||||||
|
|
||||||
|
# Downloads to user config dir
|
||||||
|
ADD https://ultralytics.com/assets/Arial.ttf https://ultralytics.com/assets/Arial.Unicode.ttf /root/.config/Ultralytics/
|
||||||
|
|
||||||
|
# Install linux packages
|
||||||
|
ENV DEBIAN_FRONTEND noninteractive
|
||||||
|
RUN apt update
|
||||||
|
RUN TZ=Etc/UTC apt install -y tzdata
|
||||||
|
RUN apt install --no-install-recommends -y python3-pip git zip curl htop gcc libgl1-mesa-glx libglib2.0-0 libpython3-dev
|
||||||
|
# RUN alias python=python3
|
||||||
|
|
||||||
|
# Create working directory
|
||||||
|
RUN mkdir -p /usr/src/ultralytics
|
||||||
|
WORKDIR /usr/src/ultralytics
|
||||||
|
|
||||||
|
# Copy contents
|
||||||
|
# COPY . /usr/src/app (issues as not a .git directory)
|
||||||
|
RUN git clone https://github.com/ultralytics/ultralytics /usr/src/ultralytics
|
||||||
|
|
||||||
|
# Install pip packages
|
||||||
|
COPY requirements.txt .
|
||||||
|
RUN python3 -m pip install --upgrade pip wheel
|
||||||
|
RUN pip install --no-cache ultralytics gsutil notebook \
|
||||||
|
tensorflow-aarch64
|
||||||
|
# tensorflowjs \
|
||||||
|
# onnx onnx-simplifier onnxruntime \
|
||||||
|
# coremltools openvino-dev \
|
||||||
|
|
||||||
|
# Cleanup
|
||||||
|
ENV DEBIAN_FRONTEND teletype
|
||||||
|
|
||||||
|
|
||||||
|
# Usage Examples -------------------------------------------------------------------------------------------------------
|
||||||
|
|
||||||
|
# Build and Push
|
||||||
|
# t=ultralytics/ultralytics:latest-arm64 && sudo docker build --platform linux/arm64 -f utils/docker/Dockerfile-arm64 -t $t . && sudo docker push $t
|
||||||
|
|
||||||
|
# Pull and Run
|
||||||
|
# t=ultralytics/ultralytics:latest-arm64 && sudo docker pull $t && sudo docker run -it --ipc=host -v "$(pwd)"/datasets:/usr/src/datasets $t
|
@ -0,0 +1,44 @@
|
|||||||
|
# YOLOv5 🚀 by Ultralytics, GPL-3.0 license
|
||||||
|
# Builds ultralytics/ultralytics:latest-cpu image on DockerHub https://hub.docker.com/r/ultralytics/ultralytics
|
||||||
|
# Image is CPU-optimized for ONNX, OpenVINO and PyTorch YOLOv5 deployments
|
||||||
|
|
||||||
|
# Start FROM Ubuntu image https://hub.docker.com/_/ubuntu
|
||||||
|
FROM ubuntu:20.04
|
||||||
|
|
||||||
|
# Downloads to user config dir
|
||||||
|
ADD https://ultralytics.com/assets/Arial.ttf https://ultralytics.com/assets/Arial.Unicode.ttf /root/.config/Ultralytics/
|
||||||
|
|
||||||
|
# Install linux packages
|
||||||
|
ENV DEBIAN_FRONTEND noninteractive
|
||||||
|
RUN apt update
|
||||||
|
RUN TZ=Etc/UTC apt install -y tzdata
|
||||||
|
RUN apt install --no-install-recommends -y python3-pip git zip curl htop libgl1-mesa-glx libglib2.0-0 libpython3-dev gnupg
|
||||||
|
# RUN alias python=python3
|
||||||
|
|
||||||
|
# Create working directory
|
||||||
|
RUN mkdir -p /usr/src/ultralytics
|
||||||
|
WORKDIR /usr/src/ultralytics
|
||||||
|
|
||||||
|
# Copy contents
|
||||||
|
# COPY . /usr/src/app (issues as not a .git directory)
|
||||||
|
RUN git clone https://github.com/ultralytics/ultralytics /usr/src/ultralytics
|
||||||
|
|
||||||
|
# Install pip packages
|
||||||
|
COPY requirements.txt .
|
||||||
|
RUN python3 -m pip install --upgrade pip wheel
|
||||||
|
RUN pip install --no-cache ultralytics albumentations gsutil notebook \
|
||||||
|
coremltools onnx onnx-simplifier onnxruntime tensorflow-cpu tensorflowjs \
|
||||||
|
# openvino-dev \
|
||||||
|
--extra-index-url https://download.pytorch.org/whl/cpu
|
||||||
|
|
||||||
|
# Cleanup
|
||||||
|
ENV DEBIAN_FRONTEND teletype
|
||||||
|
|
||||||
|
|
||||||
|
# Usage Examples -------------------------------------------------------------------------------------------------------
|
||||||
|
|
||||||
|
# Build and Push
|
||||||
|
# t=ultralytics/ultralytics:latest-cpu && sudo docker build -f utils/docker/Dockerfile-cpu -t $t . && sudo docker push $t
|
||||||
|
|
||||||
|
# Pull and Run
|
||||||
|
# t=ultralytics/ultralytics:latest-cpu && sudo docker pull $t && sudo docker run -it --ipc=host -v "$(pwd)"/datasets:/usr/src/datasets $t
|
@ -1,7 +1,85 @@
|
|||||||
## To serve docs
|
# Ultralytics Docs
|
||||||
* Install ultralytics repo in Dev mode:
|
|
||||||
|
Deployed to https://docs.ultralytics.com
|
||||||
|
|
||||||
|
### Install Ultralytics package
|
||||||
|
|
||||||
|
To install the ultralytics package in developer mode, you will need to have Git and Python 3 installed on your system.
|
||||||
|
Then, follow these steps:
|
||||||
|
|
||||||
|
1. Clone the ultralytics repository to your local machine using Git:
|
||||||
|
|
||||||
|
```bash
|
||||||
|
git clone https://github.com/ultralytics/ultralytics.git
|
||||||
```
|
```
|
||||||
|
|
||||||
|
2. Navigate to the root directory of the repository:
|
||||||
|
|
||||||
|
```bash
|
||||||
cd ultralytics
|
cd ultralytics
|
||||||
|
```
|
||||||
|
|
||||||
|
3. Install the package in developer mode using pip:
|
||||||
|
|
||||||
|
```bash
|
||||||
pip install -e '.[dev]'
|
pip install -e '.[dev]'
|
||||||
```
|
```
|
||||||
* Run `mkdocs serve`
|
|
||||||
|
This will install the ultralytics package and its dependencies in developer mode, allowing you to make changes to the
|
||||||
|
package code and have them reflected immediately in your Python environment.
|
||||||
|
|
||||||
|
Note that you may need to use the pip3 command instead of pip if you have multiple versions of Python installed on your
|
||||||
|
system.
|
||||||
|
|
||||||
|
### Building and Serving Locally
|
||||||
|
|
||||||
|
The `mkdocs serve` command is used to build and serve a local version of the MkDocs documentation site. It is typically
|
||||||
|
used during the development and testing phase of a documentation project.
|
||||||
|
|
||||||
|
```bash
|
||||||
|
mkdocs serve
|
||||||
|
```
|
||||||
|
|
||||||
|
Here is a breakdown of what this command does:
|
||||||
|
|
||||||
|
- `mkdocs`: This is the command-line interface (CLI) for the MkDocs static site generator. It is used to build and serve
|
||||||
|
MkDocs sites.
|
||||||
|
- `serve`: This is a subcommand of the `mkdocs` CLI that tells it to build and serve the documentation site locally.
|
||||||
|
- `-a`: This flag specifies the hostname and port number to bind the server to. The default value is `localhost:8000`.
|
||||||
|
- `-t`: This flag specifies the theme to use for the documentation site. The default value is `mkdocs`.
|
||||||
|
- `-s`: This flag tells the `serve` command to serve the site in silent mode, which means it will not display any log
|
||||||
|
messages or progress updates.
|
||||||
|
When you run the `mkdocs serve` command, it will build the documentation site using the files in the `docs/` directory
|
||||||
|
and serve it at the specified hostname and port number. You can then view the site by going to the URL in your web
|
||||||
|
browser.
|
||||||
|
|
||||||
|
While the site is being served, you can make changes to the documentation files and see them reflected in the live site
|
||||||
|
immediately. This is useful for testing and debugging your documentation before deploying it to a live server.
|
||||||
|
|
||||||
|
To stop the serve command and terminate the local server, you can use the `CTRL+C` keyboard shortcut.
|
||||||
|
|
||||||
|
### Deploying Your Documentation Site
|
||||||
|
|
||||||
|
To deploy your MkDocs documentation site, you will need to choose a hosting provider and a deployment method. Some
|
||||||
|
popular options include GitHub Pages, GitLab Pages, and Amazon S3.
|
||||||
|
|
||||||
|
Before you can deploy your site, you will need to configure your `mkdocs.yml` file to specify the remote host and any
|
||||||
|
other necessary deployment settings.
|
||||||
|
|
||||||
|
Once you have configured your `mkdocs.yml` file, you can use the `mkdocs deploy` command to build and deploy your site.
|
||||||
|
This command will build the documentation site using the files in the `docs/` directory and the specified configuration
|
||||||
|
file and theme, and then deploy the site to the specified remote host.
|
||||||
|
|
||||||
|
For example, to deploy your site to GitHub Pages using the gh-deploy plugin, you can use the following command:
|
||||||
|
|
||||||
|
```bash
|
||||||
|
mkdocs gh-deploy
|
||||||
|
```
|
||||||
|
|
||||||
|
If you are using GitHub Pages, you can set a custom domain for your documentation site by going to the "Settings" page
|
||||||
|
for your repository and updating the "Custom domain" field in the "GitHub Pages" section.
|
||||||
|
|
||||||
|
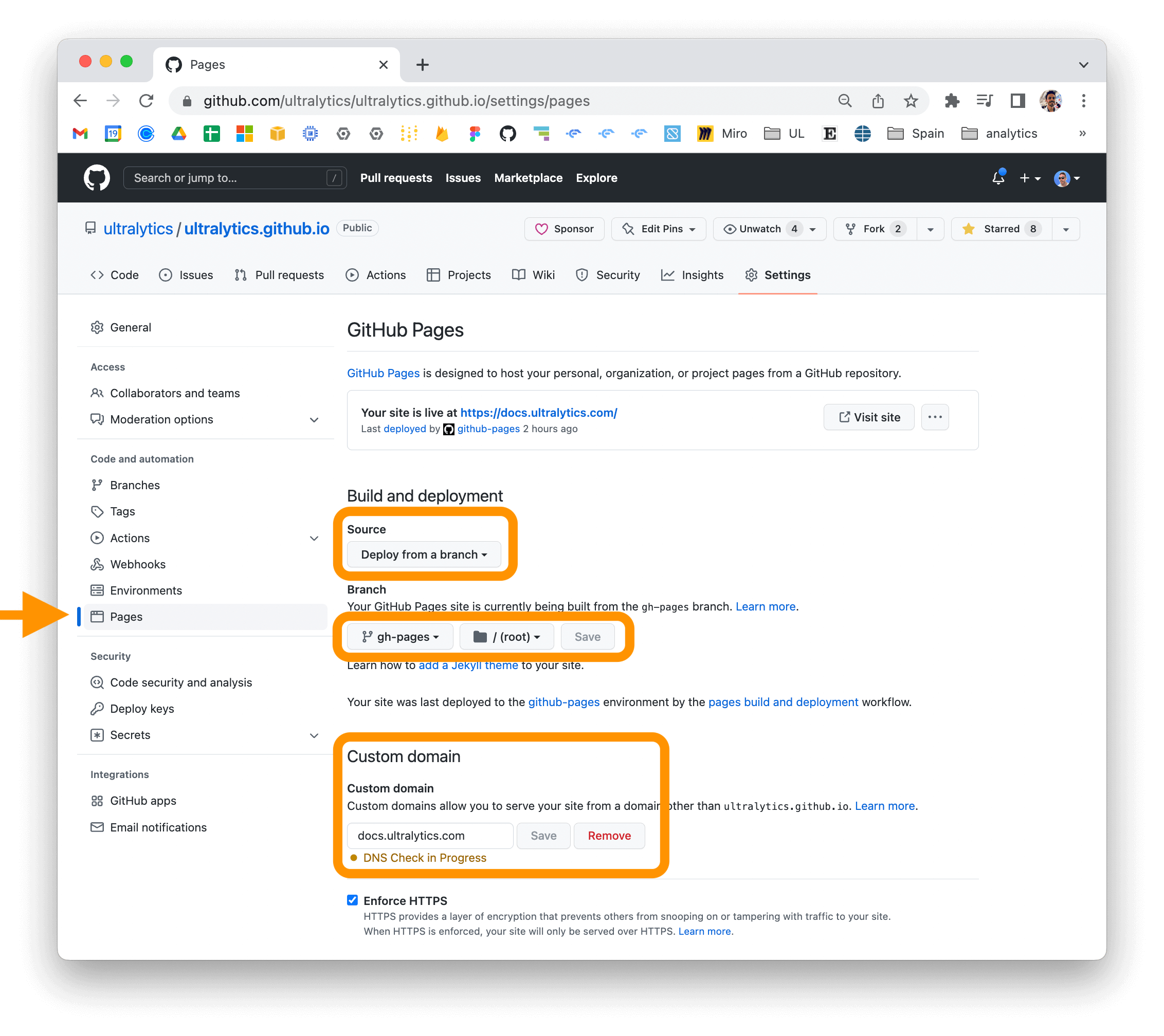
|
||||||
|
|
||||||
|
For more information on deploying your MkDocs documentation site, see
|
||||||
|
the [MkDocs documentation](https://www.mkdocs.org/user-guide/deploying-your-docs/).
|
||||||
|
@ -1,109 +0,0 @@
|
|||||||
## Ultralytics YOLO
|
|
||||||
|
|
||||||
Default training settings and hyperparameters for medium-augmentation COCO training
|
|
||||||
|
|
||||||
### Setting the operation type
|
|
||||||
???+ note "Operation"
|
|
||||||
|
|
||||||
| Key | Value | Description |
|
|
||||||
|--------|----------|---------------------------------------------------------------------------------------------------------------------------------------------------------------------------------------------|
|
|
||||||
| task | `detect` | Set the task via CLI. See Tasks for all supported tasks like - `detect`, `segment`, `classify`.<br> - `init` is a special case that creates a copy of default.yaml configs to the current working dir |
|
|
||||||
| mode | `train` | Set the mode via CLI. It can be `train`, `val`, `predict` |
|
|
||||||
| resume | `False` | Resume last given task when set to `True`. <br> Resume from a given checkpoint is `model.pt` is passed |
|
|
||||||
| model | null | Set the model. Format can differ for task type. Supports `model_name`, `model.yaml` & `model.pt` |
|
|
||||||
| data | null | Set the data. Format can differ for task type. Supports `data.yaml`, `data_folder`, `dataset_name`|
|
|
||||||
|
|
||||||
### Training settings
|
|
||||||
??? note "Train"
|
|
||||||
| Key | Value | Description |
|
|
||||||
|------------------|--------|---------------------------------------------------------------------------------|
|
|
||||||
| device | '' | cuda device, i.e. 0 or 0,1,2,3 or cpu. `''` selects available cuda 0 device |
|
|
||||||
| epochs | 100 | Number of epochs to train |
|
|
||||||
| workers | 8 | Number of cpu workers used per process. Scales automatically with DDP |
|
|
||||||
| batch_size | 16 | Batch size of the dataloader |
|
|
||||||
| imgsz | 640 | Image size of data in dataloader |
|
|
||||||
| optimizer | SGD | Optimizer used. Supported optimizer are: `Adam`, `SGD`, `RMSProp` |
|
|
||||||
| single_cls | False | Train on multi-class data as single-class |
|
|
||||||
| image_weights | False | Use weighted image selection for training |
|
|
||||||
| rect | False | Enable rectangular training |
|
|
||||||
| cos_lr | False | Use cosine LR scheduler |
|
|
||||||
| lr0 | 0.01 | Initial learning rate |
|
|
||||||
| lrf | 0.01 | Final OneCycleLR learning rate |
|
|
||||||
| momentum | 0.937 | Use as `momentum` for SGD and `beta1` for Adam |
|
|
||||||
| weight_decay | 0.0005 | Optimizer weight decay |
|
|
||||||
| warmup_epochs | 3.0 | Warmup epochs. Fractions are ok. |
|
|
||||||
| warmup_momentum | 0.8 | Warmup initial momentum |
|
|
||||||
| warmup_bias_lr | 0.1 | Warmup initial bias lr |
|
|
||||||
| box | 0.05 | Box loss gain |
|
|
||||||
| cls | 0.5 | cls loss gain |
|
|
||||||
| cls_pw | 1.0 | cls BCELoss positive_weight |
|
|
||||||
| obj | 1.0 | bj loss gain (scale with pixels) |
|
|
||||||
| obj_pw | 1.0 | obj BCELoss positive_weight |
|
|
||||||
| iou_t | 0.20 | IOU training threshold |
|
|
||||||
| anchor_t | 4.0 | anchor-multiple threshold |
|
|
||||||
| fl_gamma | 0.0 | focal loss gamma |
|
|
||||||
| label_smoothing | 0.0 | |
|
|
||||||
| nbs | 64 | nominal batch size |
|
|
||||||
| overlap_mask | `True` | **Segmentation**: Use mask overlapping during training |
|
|
||||||
| mask_ratio | 4 | **Segmentation**: Set mask downsampling |
|
|
||||||
| dropout | `False`| **Classification**: Use dropout while training |
|
|
||||||
### Prediction Settings
|
|
||||||
??? note "Prediction"
|
|
||||||
| Key | Value | Description |
|
|
||||||
|----------------|----------------------|----------------------------------------------------|
|
|
||||||
| source | `ultralytics/assets` | Input source. Accepts image, folder, video, url |
|
|
||||||
| view_img | `False` | View the prediction images |
|
|
||||||
| save_txt | `False` | Save the results in a txt file |
|
|
||||||
| save_conf | `False` | Save the condidence scores |
|
|
||||||
| save_crop | `Fasle` | |
|
|
||||||
| hide_labels | `False` | Hide the labels |
|
|
||||||
| hide_conf | `False` | Hide the confidence scores |
|
|
||||||
| vid_stride | `False` | Input video frame-rate stride |
|
|
||||||
| line_thickness | `3` | Bounding-box thickness (pixels) |
|
|
||||||
| visualize | `False` | Visualize model features |
|
|
||||||
| augment | `False` | Augmented inference |
|
|
||||||
| agnostic_nms | `False` | Class-agnostic NMS |
|
|
||||||
| retina_masks | `False` | **Segmentation:** High resolution masks |
|
|
||||||
|
|
||||||
|
|
||||||
### Validation settings
|
|
||||||
??? note "Validation"
|
|
||||||
| Key | Value | Description |
|
|
||||||
|-------------|---------|-----------------------------------|
|
|
||||||
| noval | `False` | ??? |
|
|
||||||
| save_json | `False` | |
|
|
||||||
| save_hybrid | `False` | |
|
|
||||||
| conf_thres | `0.001` | Confidence threshold |
|
|
||||||
| iou_thres | `0.6` | IoU threshold |
|
|
||||||
| max_det | `300` | Maximum number of detections |
|
|
||||||
| half | `True` | Use .half() mode. |
|
|
||||||
| dnn | `False` | Use OpenCV DNN for ONNX inference |
|
|
||||||
| plots | `False` | |
|
|
||||||
|
|
||||||
### Augmentation settings
|
|
||||||
??? note "Augmentation"
|
|
||||||
|
|
||||||
| hsv_h | 0.015 | Image HSV-Hue augmentation (fraction) |
|
|
||||||
|-------------|-------|-------------------------------------------------|
|
|
||||||
| hsv_s | 0.7 | Image HSV-Saturation augmentation (fraction) |
|
|
||||||
| hsv_v | 0.4 | Image HSV-Value augmentation (fraction) |
|
|
||||||
| degrees | 0.0 | Image rotation (+/- deg) |
|
|
||||||
| translate | 0.1 | Image translation (+/- fraction) |
|
|
||||||
| scale | 0.5 | Image scale (+/- gain) |
|
|
||||||
| shear | 0.0 | Image shear (+/- deg) |
|
|
||||||
| perspective | 0.0 | Image perspective (+/- fraction), range 0-0.001 |
|
|
||||||
| flipud | 0.0 | Image flip up-down (probability) |
|
|
||||||
| fliplr | 0.5 | Image flip left-right (probability) |
|
|
||||||
| mosaic | 1.0 | Image mosaic (probability) |
|
|
||||||
| mixup | 0.0 | Image mixup (probability) |
|
|
||||||
| copy_paste | 0.0 | Segment copy-paste (probability) |
|
|
||||||
|
|
||||||
### Logging, checkpoints, plotting and file management
|
|
||||||
??? note "files"
|
|
||||||
| Key | Value | Description |
|
|
||||||
|-----------|---------|---------------------------------------------------------------------------------------------|
|
|
||||||
| project: | 'runs' | The project name |
|
|
||||||
| name: | 'exp' | The run name. `exp` gets automatically incremented if not specified, i.e, `exp`, `exp2` ... |
|
|
||||||
| exist_ok: | `False` | ??? |
|
|
||||||
| plots | `False` | **Validation**: Save plots while validation |
|
|
||||||
| nosave | `False` | Don't save any plots, models or files |
|
|
@ -0,0 +1,202 @@
|
|||||||
|
YOLO settings and hyperparameters play a critical role in the model's performance, speed, and accuracy. These settings
|
||||||
|
and hyperparameters can affect the model's behavior at various stages of the model development process, including
|
||||||
|
training, validation, and prediction.
|
||||||
|
|
||||||
|
Properly setting and tuning these parameters can have a significant impact on the model's ability to learn effectively
|
||||||
|
from the training data and generalize to new data. For example, choosing an appropriate learning rate, batch size, and
|
||||||
|
optimization algorithm can greatly affect the model's convergence speed and accuracy. Similarly, setting the correct
|
||||||
|
confidence threshold and non-maximum suppression (NMS) threshold can affect the model's performance on detection tasks.
|
||||||
|
|
||||||
|
It is important to carefully consider and experiment with these settings and hyperparameters to achieve the best
|
||||||
|
possible performance for a given task. This can involve trial and error, as well as using techniques such as
|
||||||
|
hyperparameter optimization to search for the optimal set of parameters.
|
||||||
|
|
||||||
|
In summary, YOLO settings and hyperparameters are a key factor in the success of a YOLO model, and it is important to
|
||||||
|
pay careful attention to them to achieve the desired results.
|
||||||
|
|
||||||
|
### Setting the operation type
|
||||||
|
|
||||||
|
YOLO models can be used for a variety of tasks, including detection, segmentation, and classification. These tasks
|
||||||
|
differ in the type of output they produce and the specific problem they are designed to solve.
|
||||||
|
|
||||||
|
- Detection: Detection tasks involve identifying and localizing objects or regions of interest in an image or video.
|
||||||
|
YOLO models can be used for object detection tasks by predicting the bounding boxes and class labels of objects in an
|
||||||
|
image.
|
||||||
|
- Segmentation: Segmentation tasks involve dividing an image or video into regions or pixels that correspond to
|
||||||
|
different objects or classes. YOLO models can be used for image segmentation tasks by predicting a mask or label for
|
||||||
|
each pixel in an image.
|
||||||
|
- Classification: Classification tasks involve assigning a class label to an input, such as an image or text. YOLO
|
||||||
|
models can be used for image classification tasks by predicting the class label of an input image.
|
||||||
|
|
||||||
|
YOLO models can be used in different modes depending on the specific problem you are trying to solve. These modes
|
||||||
|
include train, val, and predict.
|
||||||
|
|
||||||
|
- Train: The train mode is used to train the model on a dataset. This mode is typically used during the development and
|
||||||
|
testing phase of a model.
|
||||||
|
- Val: The val mode is used to evaluate the model's performance on a validation dataset. This mode is typically used to
|
||||||
|
tune the model's hyperparameters and detect overfitting.
|
||||||
|
- Predict: The predict mode is used to make predictions with the model on new data. This mode is typically used in
|
||||||
|
production or when deploying the model to users.
|
||||||
|
|
||||||
|
| Key | Value | Description |
|
||||||
|
|--------|----------|-------------------------------------------------------------------------------------------------------------------------------------------------------------------------------------------------------|
|
||||||
|
| task | `detect` | Set the task via CLI. See Tasks for all supported tasks like - `detect`, `segment`, `classify`.<br> - `init` is a special case that creates a copy of default.yaml configs to the current working dir |
|
||||||
|
| mode | `train` | Set the mode via CLI. It can be `train`, `val`, `predict` |
|
||||||
|
| resume | `False` | Resume last given task when set to `True`. <br> Resume from a given checkpoint is `model.pt` is passed |
|
||||||
|
| model | null | Set the model. Format can differ for task type. Supports `model_name`, `model.yaml` & `model.pt` |
|
||||||
|
| data | null | Set the data. Format can differ for task type. Supports `data.yaml`, `data_folder`, `dataset_name` |
|
||||||
|
|
||||||
|
### Training settings
|
||||||
|
|
||||||
|
Training settings for YOLO models refer to the various hyperparameters and configurations used to train the model on a
|
||||||
|
dataset. These settings can affect the model's performance, speed, and accuracy. Some common YOLO training settings
|
||||||
|
include the batch size, learning rate, momentum, and weight decay. Other factors that may affect the training process
|
||||||
|
include the choice of optimizer, the choice of loss function, and the size and composition of the training dataset. It
|
||||||
|
is important to carefully tune and experiment with these settings to achieve the best possible performance for a given
|
||||||
|
task.
|
||||||
|
|
||||||
|
| Key | Value | Description |
|
||||||
|
|-----------------|---------|-----------------------------------------------------------------------------|
|
||||||
|
| device | '' | cuda device, i.e. 0 or 0,1,2,3 or cpu. `''` selects available cuda 0 device |
|
||||||
|
| epochs | 100 | Number of epochs to train |
|
||||||
|
| workers | 8 | Number of cpu workers used per process. Scales automatically with DDP |
|
||||||
|
| batch_size | 16 | Batch size of the dataloader |
|
||||||
|
| imgsz | 640 | Image size of data in dataloader |
|
||||||
|
| optimizer | SGD | Optimizer used. Supported optimizer are: `Adam`, `SGD`, `RMSProp` |
|
||||||
|
| single_cls | False | Train on multi-class data as single-class |
|
||||||
|
| image_weights | False | Use weighted image selection for training |
|
||||||
|
| rect | False | Enable rectangular training |
|
||||||
|
| cos_lr | False | Use cosine LR scheduler |
|
||||||
|
| lr0 | 0.01 | Initial learning rate |
|
||||||
|
| lrf | 0.01 | Final OneCycleLR learning rate |
|
||||||
|
| momentum | 0.937 | Use as `momentum` for SGD and `beta1` for Adam |
|
||||||
|
| weight_decay | 0.0005 | Optimizer weight decay |
|
||||||
|
| warmup_epochs | 3.0 | Warmup epochs. Fractions are ok. |
|
||||||
|
| warmup_momentum | 0.8 | Warmup initial momentum |
|
||||||
|
| warmup_bias_lr | 0.1 | Warmup initial bias lr |
|
||||||
|
| box | 0.05 | Box loss gain |
|
||||||
|
| cls | 0.5 | cls loss gain |
|
||||||
|
| cls_pw | 1.0 | cls BCELoss positive_weight |
|
||||||
|
| obj | 1.0 | bj loss gain (scale with pixels) |
|
||||||
|
| obj_pw | 1.0 | obj BCELoss positive_weight |
|
||||||
|
| iou_t | 0.20 | IOU training threshold |
|
||||||
|
| anchor_t | 4.0 | anchor-multiple threshold |
|
||||||
|
| fl_gamma | 0.0 | focal loss gamma |
|
||||||
|
| label_smoothing | 0.0 | |
|
||||||
|
| nbs | 64 | nominal batch size |
|
||||||
|
| overlap_mask | `True` | **Segmentation**: Use mask overlapping during training |
|
||||||
|
| mask_ratio | 4 | **Segmentation**: Set mask downsampling |
|
||||||
|
| dropout | `False` | **Classification**: Use dropout while training |
|
||||||
|
|
||||||
|
### Prediction Settings
|
||||||
|
|
||||||
|
Prediction settings for YOLO models refer to the various hyperparameters and configurations used to make predictions
|
||||||
|
with the model on new data. These settings can affect the model's performance, speed, and accuracy. Some common YOLO
|
||||||
|
prediction settings include the confidence threshold, non-maximum suppression (NMS) threshold, and the number of classes
|
||||||
|
to consider. Other factors that may affect the prediction process include the size and format of the input data, the
|
||||||
|
presence of additional features such as masks or multiple labels per box, and the specific task the model is being used
|
||||||
|
for. It is important to carefully tune and experiment with these settings to achieve the best possible performance for a
|
||||||
|
given task.
|
||||||
|
|
||||||
|
| Key | Value | Description |
|
||||||
|
|----------------|----------------------|-------------------------------------------------|
|
||||||
|
| source | `ultralytics/assets` | Input source. Accepts image, folder, video, url |
|
||||||
|
| view_img | `False` | View the prediction images |
|
||||||
|
| save_txt | `False` | Save the results in a txt file |
|
||||||
|
| save_conf | `False` | Save the condidence scores |
|
||||||
|
| save_crop | `Fasle` | |
|
||||||
|
| hide_labels | `False` | Hide the labels |
|
||||||
|
| hide_conf | `False` | Hide the confidence scores |
|
||||||
|
| vid_stride | `False` | Input video frame-rate stride |
|
||||||
|
| line_thickness | `3` | Bounding-box thickness (pixels) |
|
||||||
|
| visualize | `False` | Visualize model features |
|
||||||
|
| augment | `False` | Augmented inference |
|
||||||
|
| agnostic_nms | `False` | Class-agnostic NMS |
|
||||||
|
| retina_masks | `False` | **Segmentation:** High resolution masks |
|
||||||
|
|
||||||
|
### Validation settings
|
||||||
|
|
||||||
|
Validation settings for YOLO models refer to the various hyperparameters and configurations used to
|
||||||
|
evaluate the model's performance on a validation dataset. These settings can affect the model's performance, speed, and
|
||||||
|
accuracy. Some common YOLO validation settings include the batch size, the frequency with which validation is performed
|
||||||
|
during training, and the metrics used to evaluate the model's performance. Other factors that may affect the validation
|
||||||
|
process include the size and composition of the validation dataset and the specific task the model is being used for. It
|
||||||
|
is important to carefully tune and experiment with these settings to ensure that the model is performing well on the
|
||||||
|
validation dataset and to detect and prevent overfitting.
|
||||||
|
|
||||||
|
| Key | Value | Description |
|
||||||
|
|-------------|---------|-----------------------------------|
|
||||||
|
| noval | `False` | ??? |
|
||||||
|
| save_json | `False` | |
|
||||||
|
| save_hybrid | `False` | |
|
||||||
|
| conf_thres | `0.001` | Confidence threshold |
|
||||||
|
| iou_thres | `0.6` | IoU threshold |
|
||||||
|
| max_det | `300` | Maximum number of detections |
|
||||||
|
| half | `True` | Use .half() mode. |
|
||||||
|
| dnn | `False` | Use OpenCV DNN for ONNX inference |
|
||||||
|
| plots | `False` | |
|
||||||
|
|
||||||
|
### Export settings
|
||||||
|
|
||||||
|
Export settings for YOLO models refer to the various configurations and options used to save or
|
||||||
|
export the model for use in other environments or platforms. These settings can affect the model's performance, size,
|
||||||
|
and compatibility with different systems. Some common YOLO export settings include the format of the exported model
|
||||||
|
file (e.g. ONNX, TensorFlow SavedModel), the device on which the model will be run (e.g. CPU, GPU), and the presence of
|
||||||
|
additional features such as masks or multiple labels per box. Other factors that may affect the export process include
|
||||||
|
the specific task the model is being used for and the requirements or constraints of the target environment or platform.
|
||||||
|
It is important to carefully consider and configure these settings to ensure that the exported model is optimized for
|
||||||
|
the intended use case and can be used effectively in the target environment.
|
||||||
|
|
||||||
|
### Augmentation settings
|
||||||
|
|
||||||
|
Augmentation settings for YOLO models refer to the various transformations and modifications
|
||||||
|
applied to the training data to increase the diversity and size of the dataset. These settings can affect the model's
|
||||||
|
performance, speed, and accuracy. Some common YOLO augmentation settings include the type and intensity of the
|
||||||
|
transformations applied (e.g. random flips, rotations, cropping, color changes), the probability with which each
|
||||||
|
transformation is applied, and the presence of additional features such as masks or multiple labels per box. Other
|
||||||
|
factors that may affect the augmentation process include the size and composition of the original dataset and the
|
||||||
|
specific task the model is being used for. It is important to carefully tune and experiment with these settings to
|
||||||
|
ensure that the augmented dataset is diverse and representative enough to train a high-performing model.
|
||||||
|
|
||||||
|
| hsv_h | 0.015 | Image HSV-Hue augmentation (fraction) |
|
||||||
|
|-------------|-------|-------------------------------------------------|
|
||||||
|
| hsv_s | 0.7 | Image HSV-Saturation augmentation (fraction) |
|
||||||
|
| hsv_v | 0.4 | Image HSV-Value augmentation (fraction) |
|
||||||
|
| degrees | 0.0 | Image rotation (+/- deg) |
|
||||||
|
| translate | 0.1 | Image translation (+/- fraction) |
|
||||||
|
| scale | 0.5 | Image scale (+/- gain) |
|
||||||
|
| shear | 0.0 | Image shear (+/- deg) |
|
||||||
|
| perspective | 0.0 | Image perspective (+/- fraction), range 0-0.001 |
|
||||||
|
| flipud | 0.0 | Image flip up-down (probability) |
|
||||||
|
| fliplr | 0.5 | Image flip left-right (probability) |
|
||||||
|
| mosaic | 1.0 | Image mosaic (probability) |
|
||||||
|
| mixup | 0.0 | Image mixup (probability) |
|
||||||
|
| copy_paste | 0.0 | Segment copy-paste (probability) |
|
||||||
|
|
||||||
|
### Logging, checkpoints, plotting and file management
|
||||||
|
|
||||||
|
Logging, checkpoints, plotting, and file management are important considerations when training a YOLO model.
|
||||||
|
|
||||||
|
- Logging: It is often helpful to log various metrics and statistics during training to track the model's progress and
|
||||||
|
diagnose any issues that may arise. This can be done using a logging library such as TensorBoard or by writing log
|
||||||
|
messages to a file.
|
||||||
|
- Checkpoints: It is a good practice to save checkpoints of the model at regular intervals during training. This allows
|
||||||
|
you to resume training from a previous point if the training process is interrupted or if you want to experiment with
|
||||||
|
different training configurations.
|
||||||
|
- Plotting: Visualizing the model's performance and training progress can be helpful for understanding how the model is
|
||||||
|
behaving and identifying potential issues. This can be done using a plotting library such as matplotlib or by
|
||||||
|
generating plots using a logging library such as TensorBoard.
|
||||||
|
- File management: Managing the various files generated during the training process, such as model checkpoints, log
|
||||||
|
files, and plots, can be challenging. It is important to have a clear and organized file structure to keep track of
|
||||||
|
these files and make it easy to access and analyze them as needed.
|
||||||
|
|
||||||
|
Effective logging, checkpointing, plotting, and file management can help you keep track of the model's progress and make
|
||||||
|
it easier to debug and optimize the training process.
|
||||||
|
|
||||||
|
| Key | Value | Description |
|
||||||
|
|-----------|---------|---------------------------------------------------------------------------------------------|
|
||||||
|
| project: | 'runs' | The project name |
|
||||||
|
| name: | 'exp' | The run name. `exp` gets automatically incremented if not specified, i.e, `exp`, `exp2` ... |
|
||||||
|
| exist_ok: | `False` | ??? |
|
||||||
|
| plots | `False` | **Validation**: Save plots while validation |
|
||||||
|
| nosave | `False` | Don't save any plots, models or files |
|
@ -1,3 +1,40 @@
|
|||||||
# Welcome to Ultralytics YOLO
|
# Welcome to Ultralytics YOLO
|
||||||
|
|
||||||
TODO
|
Welcome to the Ultralytics YOLO documentation landing page! Ultralytics YOLOv8 is the latest version of the YOLO (You
|
||||||
|
Only Look Once) object detection and image segmentation model developed by Ultralytics. This page serves as the starting
|
||||||
|
point for exploring the various resources available to help you get started with YOLOv8 and understand its features and
|
||||||
|
capabilities.
|
||||||
|
|
||||||
|
The YOLOv8 model is designed to be fast, accurate, and easy to use, making it an excellent choice for a wide range of
|
||||||
|
object detection and image segmentation tasks. It can be trained on large datasets and is capable of running on a
|
||||||
|
variety of hardware platforms, from CPUs to GPUs.
|
||||||
|
|
||||||
|
Whether you are a seasoned machine learning practitioner or new to the field, we hope that the resources on this page
|
||||||
|
will help you get the most out of YOLOv8. Please feel free to browse the documentation and reach out to us with any
|
||||||
|
questions or feedback.
|
||||||
|
|
||||||
|
### A Brief History of YOLO
|
||||||
|
|
||||||
|
YOLO (You Only Look Once) is a popular object detection and image segmentation model developed by Joseph Redmon and Ali
|
||||||
|
Farhadi at the University of Washington. The first version of YOLO was released in 2015 and quickly gained popularity
|
||||||
|
due to its high speed and accuracy.
|
||||||
|
|
||||||
|
YOLOv2 was released in 2016 and improved upon the original model by incorporating batch normalization, anchor boxes, and
|
||||||
|
dimension clusters. YOLOv3 was released in 2018 and further improved the model's performance by using a more efficient
|
||||||
|
backbone network, adding a feature pyramid, and making use of focal loss.
|
||||||
|
|
||||||
|
In 2020, YOLOv4 was released which introduced a number of innovations such as the use of Mosaic data augmentation, a new
|
||||||
|
anchor-free detection head, and a new loss function.
|
||||||
|
|
||||||
|
In 2021, Ultralytics released YOLOv5, which further improved the model's performance and added new features such as
|
||||||
|
support for panoptic segmentation and object tracking.
|
||||||
|
|
||||||
|
YOLO has been widely used in a variety of applications, including autonomous vehicles, security and surveillance, and
|
||||||
|
medical imaging. It has also been used to win several competitions, such as the COCO Object Detection Challenge and the
|
||||||
|
DOTA Object Detection Challenge.
|
||||||
|
|
||||||
|
For more information about the history and development of YOLO, you can refer to the following references:
|
||||||
|
|
||||||
|
- Redmon, J., & Farhadi, A. (2015). You only look once: Unified, real-time object detection. In Proceedings of the IEEE
|
||||||
|
conference on computer vision and pattern recognition (pp. 779-788).
|
||||||
|
- Redmon, J., & Farhadi, A. (2016). YOLO9000: Better, faster, stronger. In Proceedings
|
@ -0,0 +1,5 @@
|
|||||||
|
All task Predictors are inherited from `BasePredictors` class that contains the model validation routine boilerplate. You can override any function of these Trainers to suit your needs.
|
||||||
|
|
||||||
|
---
|
||||||
|
### BasePredictor API Reference
|
||||||
|
:::ultralytics.yolo.engine.predictor.BasePredictor
|
@ -0,0 +1,5 @@
|
|||||||
|
All task Validators are inherited from `BaseValidator` class that contains the model validation routine boilerplate. You can override any function of these Trainers to suit your needs.
|
||||||
|
|
||||||
|
---
|
||||||
|
### BaseValidator API Reference
|
||||||
|
:::ultralytics.yolo.engine.validator.BaseValidator
|
@ -0,0 +1,2 @@
|
|||||||
|
### Exporter API Reference
|
||||||
|
:::ultralytics.yolo.engine.exporter.Exporter
|
Loading…
Reference in new issue