description: Learn about the MNIST dataset, a large database of handwritten digits commonly used for training various image processing systems and machine learning models.
---
---
# 🚧 Page Under Construction ⚒
# MNIST Dataset
This page is currently under construction!️ 👷Please check back later for updates. 😃🔜
The [MNIST](http://yann.lecun.com/exdb/mnist/) (Modified National Institute of Standards and Technology) dataset is a large database of handwritten digits that is commonly used for training various image processing systems and machine learning models. It was created by "re-mixing" the samples from NIST's original datasets and has become a benchmark for evaluating the performance of image classification algorithms.
## Key Features
- MNIST contains 60,000 training images and 10,000 testing images of handwritten digits.
- The dataset comprises grayscale images of size 28x28 pixels.
- The images are normalized to fit into a 28x28 pixel bounding box and anti-aliased, introducing grayscale levels.
- MNIST is widely used for training and testing in the field of machine learning, especially for image classification tasks.
## Dataset Structure
The MNIST dataset is split into two subsets:
1. **Training Set**: This subset contains 60,000 images of handwritten digits used for training machine learning models.
2. **Testing Set**: This subset consists of 10,000 images used for testing and benchmarking the trained models.
## Extended MNIST (EMNIST)
Extended MNIST (EMNIST) is a newer dataset developed and released by NIST to be the successor to MNIST. While MNIST included images only of handwritten digits, EMNIST includes all the images from NIST Special Database 19, which is a large database of handwritten uppercase and lowercase letters as well as digits. The images in EMNIST were converted into the same 28x28 pixel format, by the same process, as were the MNIST images. Accordingly, tools that work with the older, smaller MNIST dataset will likely work unmodified with EMNIST.
## Applications
The MNIST dataset is widely used for training and evaluating deep learning models in image classification tasks, such as Convolutional Neural Networks (CNNs), Support Vector Machines (SVMs), and various other machine learning algorithms. The dataset's simple and well-structured format makes it an essential resource for researchers and practitioners in the field of machine learning and computer vision.
## Usage
To train a CNN model on the MNIST dataset for 100 epochs with an image size of 32x32, you can use the following code snippets. For a comprehensive list of available arguments, refer to the model [Training](../../modes/train.md) page.
!!! example "Train Example"
=== "Python"
```python
from ultralytics import YOLO
# Load a model
model = YOLO('yolov8n-cls.pt') # load a pretrained model (recommended for training)
The MNIST dataset contains grayscale images of handwritten digits, providing a well-structured dataset for image classification tasks. Here are some examples of images from the dataset:
The example showcases the variety and complexity of the handwritten digits in the MNIST dataset, highlighting the importance of a diverse dataset for training robust image classification models.
## Citations and Acknowledgments
If you use the MNIST dataset in your
research or development work, please cite the following paper:
```bibtex
@article{lecun2010mnist,
title={MNIST handwritten digit database},
author={LeCun, Yann and Cortes, Corinna and Burges, CJ},
We would like to acknowledge Yann LeCun, Corinna Cortes, and Christopher J.C. Burges for creating and maintaining the MNIST dataset as a valuable resource for the machine learning and computer vision research community. For more information about the MNIST dataset and its creators, visit the [MNIST dataset website](http://yann.lecun.com/exdb/mnist/).
Real-Time Detection Transformer (RT-DETR) is an end-to-end object detector that provides real-time performance while maintaining high accuracy. It efficiently processes multi-scale features by decoupling intra-scale interaction and cross-scale fusion, and supports flexible adjustment of inference speed using different decoder layers without retraining. RT-DETR outperforms many real-time object detectors on accelerated backends like CUDA with TensorRT.
Real-Time Detection Transformer (RT-DETR) is an end-to-end object detector that provides real-time performance while maintaining high accuracy. It efficiently processes multi-scale features by decoupling intra-scale interaction and cross-scale fusion, and supports flexible adjustment of inference speed using different decoder layers without retraining. RT-DETR outperforms many real-time object detectors on accelerated backends like CUDA with TensorRT.
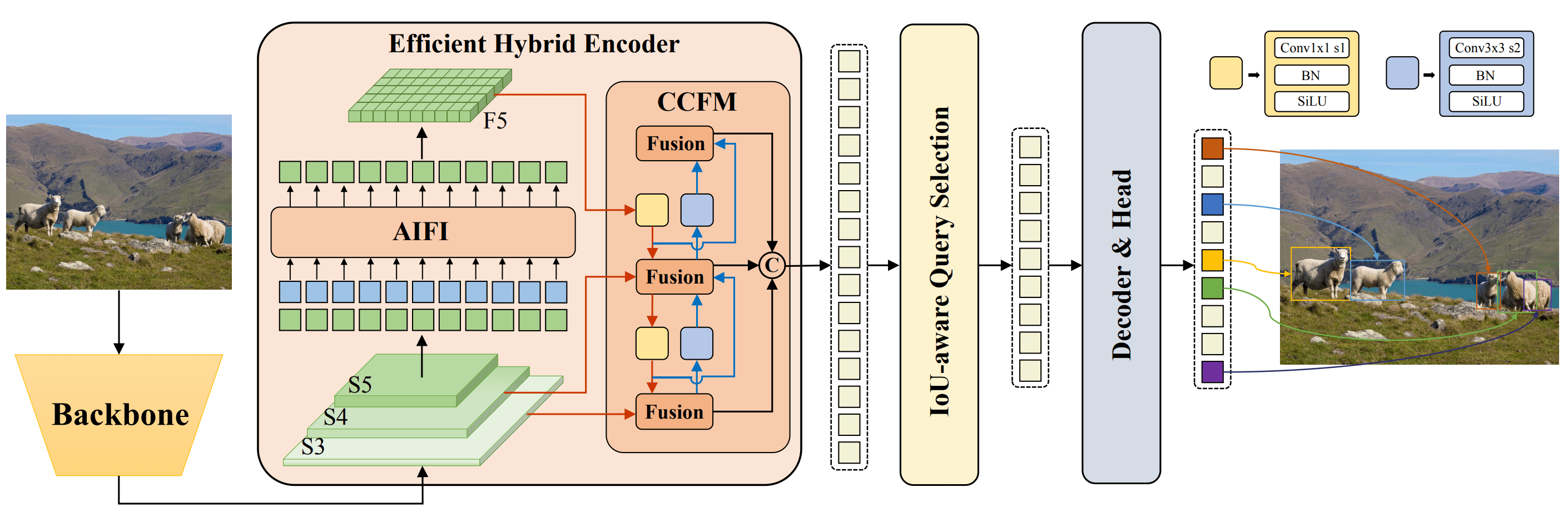
**Overview of RT-DETR.** Model architecture diagram showing the last three stages of the backbone {S3, S4, S5} as the input
to the encoder. The efficient hybrid encoder transforms multiscale features into a sequence of image features through intrascale feature interaction (AIFI) and cross-scale feature-fusion module (CCFM). The IoU-aware query selection is employed
to select a fixed number of image features to serve as initial object queries for the decoder. Finally, the decoder with auxiliary
prediction heads iteratively optimizes object queries to generate boxes and confidence scores ([source](https://arxiv.org/pdf/2304.08069.pdf)).
### Key Features
### Key Features
- **Efficient Hybrid Encoder:** RT-DETR uses an efficient hybrid encoder that processes multi-scale features by decoupling intra-scale interaction and cross-scale fusion. This design reduces computational costs and allows for real-time object detection.
- **Efficient Hybrid Encoder:** RT-DETR uses an efficient hybrid encoder that processes multi-scale features by decoupling intra-scale interaction and cross-scale fusion. This design reduces computational costs and allows for real-time object detection.