diff --git a/docs/datasets/classify/caltech101.md b/docs/datasets/classify/caltech101.md index 765c100..bc7e911 100644 --- a/docs/datasets/classify/caltech101.md +++ b/docs/datasets/classify/caltech101.md @@ -39,7 +39,7 @@ To train a YOLO model on the Caltech-101 dataset for 100 epochs, you can use the model = YOLO('yolov8n-cls.pt') # load a pretrained model (recommended for training) # Train the model - model.train(data='caltech101', epochs=100, imgsz=416) + results = model.train(data='caltech101', epochs=100, imgsz=416) ``` === "CLI" @@ -61,17 +61,21 @@ The example showcases the variety and complexity of the objects in the Caltech-1 If you use the Caltech-101 dataset in your research or development work, please cite the following paper: -```bibtex -@article{fei2007learning, - title={Learning generative visual models from few training examples: An incremental Bayesian approach tested on 101 object categories}, - author={Fei-Fei, Li and Fergus, Rob and Perona, Pietro}, - journal={Computer vision and Image understanding}, - volume={106}, - number={1}, - pages={59--70}, - year={2007}, - publisher={Elsevier} -} -``` +!!! note "" + + === "BibTeX" + + ```bibtex + @article{fei2007learning, + title={Learning generative visual models from few training examples: An incremental Bayesian approach tested on 101 object categories}, + author={Fei-Fei, Li and Fergus, Rob and Perona, Pietro}, + journal={Computer vision and Image understanding}, + volume={106}, + number={1}, + pages={59--70}, + year={2007}, + publisher={Elsevier} + } + ``` We would like to acknowledge Li Fei-Fei, Rob Fergus, and Pietro Perona for creating and maintaining the Caltech-101 dataset as a valuable resource for the machine learning and computer vision research community. For more information about the Caltech-101 dataset and its creators, visit the [Caltech-101 dataset website](https://data.caltech.edu/records/mzrjq-6wc02). diff --git a/docs/datasets/classify/caltech256.md b/docs/datasets/classify/caltech256.md index 5830e0b..664167d 100644 --- a/docs/datasets/classify/caltech256.md +++ b/docs/datasets/classify/caltech256.md @@ -39,7 +39,7 @@ To train a YOLO model on the Caltech-256 dataset for 100 epochs, you can use the model = YOLO('yolov8n-cls.pt') # load a pretrained model (recommended for training) # Train the model - model.train(data='caltech256', epochs=100, imgsz=416) + results = model.train(data='caltech256', epochs=100, imgsz=416) ``` === "CLI" @@ -61,13 +61,17 @@ The example showcases the diversity and complexity of the objects in the Caltech If you use the Caltech-256 dataset in your research or development work, please cite the following paper: -```bibtex -@article{griffin2007caltech, - title={Caltech-256 object category dataset}, - author={Griffin, Gregory and Holub, Alex and Perona, Pietro}, - year={2007} -} -``` +!!! note "" + + === "BibTeX" + + ```bibtex + @article{griffin2007caltech, + title={Caltech-256 object category dataset}, + author={Griffin, Gregory and Holub, Alex and Perona, Pietro}, + year={2007} + } + ``` We would like to acknowledge Gregory Griffin, Alex Holub, and Pietro Perona for creating and maintaining the Caltech-256 dataset as a valuable resource for the machine learning and computer vision research community. For more information about the diff --git a/docs/datasets/classify/cifar10.md b/docs/datasets/classify/cifar10.md index 4292564..100c238 100644 --- a/docs/datasets/classify/cifar10.md +++ b/docs/datasets/classify/cifar10.md @@ -42,7 +42,7 @@ To train a YOLO model on the CIFAR-10 dataset for 100 epochs with an image size model = YOLO('yolov8n-cls.pt') # load a pretrained model (recommended for training) # Train the model - model.train(data='cifar10', epochs=100, imgsz=32) + results = model.train(data='cifar10', epochs=100, imgsz=32) ``` === "CLI" @@ -64,13 +64,17 @@ The example showcases the variety and complexity of the objects in the CIFAR-10 If you use the CIFAR-10 dataset in your research or development work, please cite the following paper: -```bibtex -@TECHREPORT{Krizhevsky09learningmultiple, - author={Alex Krizhevsky}, - title={Learning multiple layers of features from tiny images}, - institution={}, - year={2009} -} -``` +!!! note "" + + === "BibTeX" + + ```bibtex + @TECHREPORT{Krizhevsky09learningmultiple, + author={Alex Krizhevsky}, + title={Learning multiple layers of features from tiny images}, + institution={}, + year={2009} + } + ``` We would like to acknowledge Alex Krizhevsky for creating and maintaining the CIFAR-10 dataset as a valuable resource for the machine learning and computer vision research community. For more information about the CIFAR-10 dataset and its creator, visit the [CIFAR-10 dataset website](https://www.cs.toronto.edu/~kriz/cifar.html). diff --git a/docs/datasets/classify/cifar100.md b/docs/datasets/classify/cifar100.md index c5c9b7d..9f7f407 100644 --- a/docs/datasets/classify/cifar100.md +++ b/docs/datasets/classify/cifar100.md @@ -42,7 +42,7 @@ To train a YOLO model on the CIFAR-100 dataset for 100 epochs with an image size model = YOLO('yolov8n-cls.pt') # load a pretrained model (recommended for training) # Train the model - model.train(data='cifar100', epochs=100, imgsz=32) + results = model.train(data='cifar100', epochs=100, imgsz=32) ``` === "CLI" @@ -64,13 +64,17 @@ The example showcases the variety and complexity of the objects in the CIFAR-100 If you use the CIFAR-100 dataset in your research or development work, please cite the following paper: -```bibtex -@TECHREPORT{Krizhevsky09learningmultiple, - author={Alex Krizhevsky}, - title={Learning multiple layers of features from tiny images}, - institution={}, - year={2009} -} -``` +!!! note "" + + === "BibTeX" + + ```bibtex + @TECHREPORT{Krizhevsky09learningmultiple, + author={Alex Krizhevsky}, + title={Learning multiple layers of features from tiny images}, + institution={}, + year={2009} + } + ``` We would like to acknowledge Alex Krizhevsky for creating and maintaining the CIFAR-100 dataset as a valuable resource for the machine learning and computer vision research community. For more information about the CIFAR-100 dataset and its creator, visit the [CIFAR-100 dataset website](https://www.cs.toronto.edu/~kriz/cifar.html). diff --git a/docs/datasets/classify/fashion-mnist.md b/docs/datasets/classify/fashion-mnist.md index 876bed2..319b221 100644 --- a/docs/datasets/classify/fashion-mnist.md +++ b/docs/datasets/classify/fashion-mnist.md @@ -56,7 +56,7 @@ To train a CNN model on the Fashion-MNIST dataset for 100 epochs with an image s model = YOLO('yolov8n-cls.pt') # load a pretrained model (recommended for training) # Train the model - model.train(data='fashion-mnist', epochs=100, imgsz=28) + results = model.train(data='fashion-mnist', epochs=100, imgsz=28) ``` === "CLI" diff --git a/docs/datasets/classify/imagenet.md b/docs/datasets/classify/imagenet.md index ba7da29..008a3ff 100644 --- a/docs/datasets/classify/imagenet.md +++ b/docs/datasets/classify/imagenet.md @@ -42,7 +42,7 @@ To train a deep learning model on the ImageNet dataset for 100 epochs with an im model = YOLO('yolov8n-cls.pt') # load a pretrained model (recommended for training) # Train the model - model.train(data='imagenet', epochs=100, imgsz=224) + results = model.train(data='imagenet', epochs=100, imgsz=224) ``` === "CLI" @@ -64,16 +64,20 @@ The example showcases the variety and complexity of the images in the ImageNet d If you use the ImageNet dataset in your research or development work, please cite the following paper: -```bibtex -@article{ILSVRC15, - author = {Olga Russakovsky and Jia Deng and Hao Su and Jonathan Krause and Sanjeev Satheesh and Sean Ma and Zhiheng Huang and Andrej Karpathy and Aditya Khosla and Michael Bernstein and Alexander C. Berg and Li Fei-Fei}, - title={ImageNet Large Scale Visual Recognition Challenge}, - year={2015}, - journal={International Journal of Computer Vision (IJCV)}, - volume={115}, - number={3}, - pages={211-252} -} -``` +!!! note "" + + === "BibTeX" + + ```bibtex + @article{ILSVRC15, + author = {Olga Russakovsky and Jia Deng and Hao Su and Jonathan Krause and Sanjeev Satheesh and Sean Ma and Zhiheng Huang and Andrej Karpathy and Aditya Khosla and Michael Bernstein and Alexander C. Berg and Li Fei-Fei}, + title={ImageNet Large Scale Visual Recognition Challenge}, + year={2015}, + journal={International Journal of Computer Vision (IJCV)}, + volume={115}, + number={3}, + pages={211-252} + } + ``` We would like to acknowledge the ImageNet team, led by Olga Russakovsky, Jia Deng, and Li Fei-Fei, for creating and maintaining the ImageNet dataset as a valuable resource for the machine learning and computer vision research community. For more information about the ImageNet dataset and its creators, visit the [ImageNet website](https://www.image-net.org/). diff --git a/docs/datasets/classify/imagenet10.md b/docs/datasets/classify/imagenet10.md index 3520f4c..886d5af 100644 --- a/docs/datasets/classify/imagenet10.md +++ b/docs/datasets/classify/imagenet10.md @@ -38,7 +38,7 @@ To test a deep learning model on the ImageNet10 dataset with an image size of 22 model = YOLO('yolov8n-cls.pt') # load a pretrained model (recommended for training) # Train the model - model.train(data='imagenet10', epochs=5, imgsz=224) + results = model.train(data='imagenet10', epochs=5, imgsz=224) ``` === "CLI" @@ -59,16 +59,20 @@ The example showcases the variety and complexity of the images in the ImageNet10 If you use the ImageNet10 dataset in your research or development work, please cite the original ImageNet paper: -```bibtex -@article{ILSVRC15, - author = {Olga Russakovsky and Jia Deng and Hao Su and Jonathan Krause and Sanjeev Satheesh and Sean Ma and Zhiheng Huang and Andrej Karpathy and Aditya Khosla and Michael Bernstein and Alexander C. Berg and Li Fei-Fei}, - title={ImageNet Large Scale Visual Recognition Challenge}, - year={2015}, - journal={International Journal of Computer Vision (IJCV)}, - volume={115}, - number={3}, - pages={211-252} -} -``` +!!! note "" + + === "BibTeX" + + ```bibtex + @article{ILSVRC15, + author = {Olga Russakovsky and Jia Deng and Hao Su and Jonathan Krause and Sanjeev Satheesh and Sean Ma and Zhiheng Huang and Andrej Karpathy and Aditya Khosla and Michael Bernstein and Alexander C. Berg and Li Fei-Fei}, + title={ImageNet Large Scale Visual Recognition Challenge}, + year={2015}, + journal={International Journal of Computer Vision (IJCV)}, + volume={115}, + number={3}, + pages={211-252} + } + ``` We would like to acknowledge the ImageNet team, led by Olga Russakovsky, Jia Deng, and Li Fei-Fei, for creating and maintaining the ImageNet dataset. The ImageNet10 dataset, while a compact subset, is a valuable resource for quick testing and debugging in the machine learning and computer vision research community. For more information about the ImageNet dataset and its creators, visit the [ImageNet website](https://www.image-net.org/). diff --git a/docs/datasets/classify/imagenette.md b/docs/datasets/classify/imagenette.md index 968e279..cc5cb27 100644 --- a/docs/datasets/classify/imagenette.md +++ b/docs/datasets/classify/imagenette.md @@ -40,7 +40,7 @@ To train a model on the ImageNette dataset for 100 epochs with a standard image model = YOLO('yolov8n-cls.pt') # load a pretrained model (recommended for training) # Train the model - model.train(data='imagenette', epochs=100, imgsz=224) + results = model.train(data='imagenette', epochs=100, imgsz=224) ``` === "CLI" @@ -75,7 +75,7 @@ To use these datasets, simply replace 'imagenette' with 'imagenette160' or 'imag model = YOLO('yolov8n-cls.pt') # load a pretrained model (recommended for training) # Train the model with ImageNette160 - model.train(data='imagenette160', epochs=100, imgsz=160) + results = model.train(data='imagenette160', epochs=100, imgsz=160) ``` === "CLI" @@ -96,7 +96,7 @@ To use these datasets, simply replace 'imagenette' with 'imagenette160' or 'imag model = YOLO('yolov8n-cls.pt') # load a pretrained model (recommended for training) # Train the model with ImageNette320 - model.train(data='imagenette320', epochs=100, imgsz=320) + results = model.train(data='imagenette320', epochs=100, imgsz=320) ``` === "CLI" diff --git a/docs/datasets/classify/imagewoof.md b/docs/datasets/classify/imagewoof.md index 86e51f7..2c1271f 100644 --- a/docs/datasets/classify/imagewoof.md +++ b/docs/datasets/classify/imagewoof.md @@ -37,7 +37,7 @@ To train a CNN model on the ImageWoof dataset for 100 epochs with an image size model = YOLO('yolov8n-cls.pt') # load a pretrained model (recommended for training) # Train the model - model.train(data='imagewoof', epochs=100, imgsz=224) + results = model.train(data='imagewoof', epochs=100, imgsz=224) ``` === "CLI" @@ -79,6 +79,6 @@ The example showcases the subtle differences and similarities among the differen ## Citations and Acknowledgments -If you use the ImageWoof dataset in your research or development work, please make sure to acknowledge the creators of the dataset by linking to the [official dataset repository](https://github.com/fastai/imagenette). As of my knowledge cutoff in September 2021, there is no official publication specifically about ImageWoof for citation. +If you use the ImageWoof dataset in your research or development work, please make sure to acknowledge the creators of the dataset by linking to the [official dataset repository](https://github.com/fastai/imagenette). We would like to acknowledge the FastAI team for creating and maintaining the ImageWoof dataset as a valuable resource for the machine learning and computer vision research community. For more information about the ImageWoof dataset, visit the [ImageWoof dataset repository](https://github.com/fastai/imagenette). diff --git a/docs/datasets/classify/index.md b/docs/datasets/classify/index.md index 220d3aa..748701e 100644 --- a/docs/datasets/classify/index.md +++ b/docs/datasets/classify/index.md @@ -91,7 +91,7 @@ In this example, the `train` directory contains subdirectories for each class in model = YOLO('yolov8n-cls.pt') # load a pretrained model (recommended for training) # Train the model - model.train(data='path/to/dataset', epochs=100, imgsz=640) + results = model.train(data='path/to/dataset', epochs=100, imgsz=640) ``` === "CLI" diff --git a/docs/datasets/classify/mnist.md b/docs/datasets/classify/mnist.md index 9dac46b..40f6a77 100644 --- a/docs/datasets/classify/mnist.md +++ b/docs/datasets/classify/mnist.md @@ -45,7 +45,7 @@ To train a CNN model on the MNIST dataset for 100 epochs with an image size of 3 model = YOLO('yolov8n-cls.pt') # load a pretrained model (recommended for training) # Train the model - model.train(data='mnist', epochs=100, imgsz=32) + results = model.train(data='mnist', epochs=100, imgsz=32) ``` === "CLI" @@ -69,14 +69,18 @@ If you use the MNIST dataset in your research or development work, please cite the following paper: -```bibtex -@article{lecun2010mnist, - title={MNIST handwritten digit database}, - author={LeCun, Yann and Cortes, Corinna and Burges, CJ}, - journal={ATT Labs [Online]. Available: http://yann.lecun.com/exdb/mnist}, - volume={2}, - year={2010} -} -``` +!!! note "" + + === "BibTeX" + + ```bibtex + @article{lecun2010mnist, + title={MNIST handwritten digit database}, + author={LeCun, Yann and Cortes, Corinna and Burges, CJ}, + journal={ATT Labs [Online]. Available: http://yann.lecun.com/exdb/mnist}, + volume={2}, + year={2010} + } + ``` We would like to acknowledge Yann LeCun, Corinna Cortes, and Christopher J.C. Burges for creating and maintaining the MNIST dataset as a valuable resource for the machine learning and computer vision research community. For more information about the MNIST dataset and its creators, visit the [MNIST dataset website](http://yann.lecun.com/exdb/mnist/). diff --git a/docs/datasets/detect/argoverse.md b/docs/datasets/detect/argoverse.md index 0836fa4..cbc2fd2 100644 --- a/docs/datasets/detect/argoverse.md +++ b/docs/datasets/detect/argoverse.md @@ -12,7 +12,6 @@ The [Argoverse](https://www.argoverse.org/) dataset is a collection of data desi The Argoverse dataset *.zip file required for training was removed from Amazon S3 after the shutdown of Argo AI by Ford, but we have made it available for manual download on [Google Drive](https://drive.google.com/file/d/1st9qW3BeIwQsnR0t8mRpvbsSWIo16ACi/view?usp=drive_link). - ## Key Features - Argoverse contains over 290K labeled 3D object tracks and 5 million object instances across 1,263 distinct scenes. @@ -57,7 +56,7 @@ To train a YOLOv8n model on the Argoverse dataset for 100 epochs with an image s model = YOLO('yolov8n.pt') # load a pretrained model (recommended for training) # Train the model - model.train(data='Argoverse.yaml', epochs=100, imgsz=640) + results = model.train(data='Argoverse.yaml', epochs=100, imgsz=640) ``` === "CLI" @@ -81,14 +80,18 @@ The example showcases the variety and complexity of the data in the Argoverse da If you use the Argoverse dataset in your research or development work, please cite the following paper: -```bibtex -@inproceedings{chang2019argoverse, - title={Argoverse: 3D Tracking and Forecasting with Rich Maps}, - author={Chang, Ming-Fang and Lambert, John and Sangkloy, Patsorn and Singh, Jagjeet and Bak, Slawomir and Hartnett, Andrew and Wang, Dequan and Carr, Peter and Lucey, Simon and Ramanan, Deva and others}, - booktitle={Proceedings of the IEEE/CVF Conference on Computer Vision and Pattern Recognition}, - pages={8748--8757}, - year={2019} -} -``` +!!! note "" + + === "BibTeX" + + ```bibtex + @inproceedings{chang2019argoverse, + title={Argoverse: 3D Tracking and Forecasting with Rich Maps}, + author={Chang, Ming-Fang and Lambert, John and Sangkloy, Patsorn and Singh, Jagjeet and Bak, Slawomir and Hartnett, Andrew and Wang, Dequan and Carr, Peter and Lucey, Simon and Ramanan, Deva and others}, + booktitle={Proceedings of the IEEE/CVF Conference on Computer Vision and Pattern Recognition}, + pages={8748--8757}, + year={2019} + } + ``` We would like to acknowledge Argo AI for creating and maintaining the Argoverse dataset as a valuable resource for the autonomous driving research community. For more information about the Argoverse dataset and its creators, visit the [Argoverse dataset website](https://www.argoverse.org/). diff --git a/docs/datasets/detect/coco.md b/docs/datasets/detect/coco.md index b8d1934..9030370 100644 --- a/docs/datasets/detect/coco.md +++ b/docs/datasets/detect/coco.md @@ -52,7 +52,7 @@ To train a YOLOv8n model on the COCO dataset for 100 epochs with an image size o model = YOLO('yolov8n.pt') # load a pretrained model (recommended for training) # Train the model - model.train(data='coco.yaml', epochs=100, imgsz=640) + results = model.train(data='coco.yaml', epochs=100, imgsz=640) ``` === "CLI" @@ -76,15 +76,19 @@ The example showcases the variety and complexity of the images in the COCO datas If you use the COCO dataset in your research or development work, please cite the following paper: -```bibtex -@misc{lin2015microsoft, - title={Microsoft COCO: Common Objects in Context}, - author={Tsung-Yi Lin and Michael Maire and Serge Belongie and Lubomir Bourdev and Ross Girshick and James Hays and Pietro Perona and Deva Ramanan and C. Lawrence Zitnick and Piotr Dollár}, - year={2015}, - eprint={1405.0312}, - archivePrefix={arXiv}, - primaryClass={cs.CV} -} -``` +!!! note "" + + === "BibTeX" + + ```bibtex + @misc{lin2015microsoft, + title={Microsoft COCO: Common Objects in Context}, + author={Tsung-Yi Lin and Michael Maire and Serge Belongie and Lubomir Bourdev and Ross Girshick and James Hays and Pietro Perona and Deva Ramanan and C. Lawrence Zitnick and Piotr Dollár}, + year={2015}, + eprint={1405.0312}, + archivePrefix={arXiv}, + primaryClass={cs.CV} + } + ``` We would like to acknowledge the COCO Consortium for creating and maintaining this valuable resource for the computer vision community. For more information about the COCO dataset and its creators, visit the [COCO dataset website](https://cocodataset.org/#home). diff --git a/docs/datasets/detect/coco8.md b/docs/datasets/detect/coco8.md index 7fc71fb..c365b3b 100644 --- a/docs/datasets/detect/coco8.md +++ b/docs/datasets/detect/coco8.md @@ -42,7 +42,7 @@ To train a YOLOv8n model on the COCO8 dataset for 100 epochs with an image size model = YOLO('yolov8n.pt') # load a pretrained model (recommended for training) # Train the model - model.train(data='coco8.yaml', epochs=100, imgsz=640) + results = model.train(data='coco8.yaml', epochs=100, imgsz=640) ``` === "CLI" @@ -66,15 +66,19 @@ The example showcases the variety and complexity of the images in the COCO8 data If you use the COCO dataset in your research or development work, please cite the following paper: -```bibtex -@misc{lin2015microsoft, - title={Microsoft COCO: Common Objects in Context}, - author={Tsung-Yi Lin and Michael Maire and Serge Belongie and Lubomir Bourdev and Ross Girshick and James Hays and Pietro Perona and Deva Ramanan and C. Lawrence Zitnick and Piotr Dollár}, - year={2015}, - eprint={1405.0312}, - archivePrefix={arXiv}, - primaryClass={cs.CV} -} -``` +!!! note "" + + === "BibTeX" + + ```bibtex + @misc{lin2015microsoft, + title={Microsoft COCO: Common Objects in Context}, + author={Tsung-Yi Lin and Michael Maire and Serge Belongie and Lubomir Bourdev and Ross Girshick and James Hays and Pietro Perona and Deva Ramanan and C. Lawrence Zitnick and Piotr Dollár}, + year={2015}, + eprint={1405.0312}, + archivePrefix={arXiv}, + primaryClass={cs.CV} + } + ``` We would like to acknowledge the COCO Consortium for creating and maintaining this valuable resource for the computer vision community. For more information about the COCO dataset and its creators, visit the [COCO dataset website](https://cocodataset.org/#home). diff --git a/docs/datasets/detect/globalwheat2020.md b/docs/datasets/detect/globalwheat2020.md index 3a60aa0..0f0d7c0 100644 --- a/docs/datasets/detect/globalwheat2020.md +++ b/docs/datasets/detect/globalwheat2020.md @@ -51,7 +51,7 @@ To train a YOLOv8n model on the Global Wheat Head Dataset for 100 epochs with an model = YOLO('yolov8n.pt') # load a pretrained model (recommended for training) # Train the model - model.train(data='GlobalWheat2020.yaml', epochs=100, imgsz=640) + results = model.train(data='GlobalWheat2020.yaml', epochs=100, imgsz=640) ``` === "CLI" @@ -75,13 +75,17 @@ The example showcases the variety and complexity of the data in the Global Wheat If you use the Global Wheat Head Dataset in your research or development work, please cite the following paper: -```bibtex -@article{david2020global, - title={Global Wheat Head Detection (GWHD) Dataset: A Large and Diverse Dataset of High-Resolution RGB-Labelled Images to Develop and Benchmark Wheat Head Detection Methods}, - author={David, Etienne and Madec, Simon and Sadeghi-Tehran, Pouria and Aasen, Helge and Zheng, Bangyou and Liu, Shouyang and Kirchgessner, Norbert and Ishikawa, Goro and Nagasawa, Koichi and Badhon, Minhajul and others}, - journal={arXiv preprint arXiv:2005.02162}, - year={2020} -} -``` +!!! note "" + + === "BibTeX" + + ```bibtex + @article{david2020global, + title={Global Wheat Head Detection (GWHD) Dataset: A Large and Diverse Dataset of High-Resolution RGB-Labelled Images to Develop and Benchmark Wheat Head Detection Methods}, + author={David, Etienne and Madec, Simon and Sadeghi-Tehran, Pouria and Aasen, Helge and Zheng, Bangyou and Liu, Shouyang and Kirchgessner, Norbert and Ishikawa, Goro and Nagasawa, Koichi and Badhon, Minhajul and others}, + journal={arXiv preprint arXiv:2005.02162}, + year={2020} + } + ``` We would like to acknowledge the researchers and institutions that contributed to the creation and maintenance of the Global Wheat Head Dataset as a valuable resource for the plant phenotyping and crop management research community. For more information about the dataset and its creators, visit the [Global Wheat Head Dataset website](http://www.global-wheat.com/). diff --git a/docs/datasets/detect/index.md b/docs/datasets/detect/index.md index b6dd1e1..f93e6b0 100644 --- a/docs/datasets/detect/index.md +++ b/docs/datasets/detect/index.md @@ -59,7 +59,7 @@ Here's how you can use these formats to train your model: model = YOLO('yolov8n.pt') # load a pretrained model (recommended for training) # Train the model - model.train(data='coco8.yaml', epochs=100, imgsz=640) + results = model.train(data='coco8.yaml', epochs=100, imgsz=640) ``` === "CLI" diff --git a/docs/datasets/detect/objects365.md b/docs/datasets/detect/objects365.md index 0254b47..06301b1 100644 --- a/docs/datasets/detect/objects365.md +++ b/docs/datasets/detect/objects365.md @@ -51,7 +51,7 @@ To train a YOLOv8n model on the Objects365 dataset for 100 epochs with an image model = YOLO('yolov8n.pt') # load a pretrained model (recommended for training) # Train the model - model.train(data='Objects365.yaml', epochs=100, imgsz=640) + results = model.train(data='Objects365.yaml', epochs=100, imgsz=640) ``` === "CLI" @@ -75,14 +75,18 @@ The example showcases the variety and complexity of the data in the Objects365 d If you use the Objects365 dataset in your research or development work, please cite the following paper: -```bibtex -@inproceedings{shao2019objects365, - title={Objects365: A Large-scale, High-quality Dataset for Object Detection}, - author={Shao, Shuai and Li, Zeming and Zhang, Tianyuan and Peng, Chao and Yu, Gang and Li, Jing and Zhang, Xiangyu and Sun, Jian}, - booktitle={Proceedings of the IEEE/CVF International Conference on Computer Vision}, - pages={8425--8434}, - year={2019} -} -``` +!!! note "" + + === "BibTeX" + + ```bibtex + @inproceedings{shao2019objects365, + title={Objects365: A Large-scale, High-quality Dataset for Object Detection}, + author={Shao, Shuai and Li, Zeming and Zhang, Tianyuan and Peng, Chao and Yu, Gang and Li, Jing and Zhang, Xiangyu and Sun, Jian}, + booktitle={Proceedings of the IEEE/CVF International Conference on Computer Vision}, + pages={8425--8434}, + year={2019} + } + ``` We would like to acknowledge the team of researchers who created and maintain the Objects365 dataset as a valuable resource for the computer vision research community. For more information about the Objects365 dataset and its creators, visit the [Objects365 dataset website](https://www.objects365.org/). diff --git a/docs/datasets/detect/open-images-v7.md b/docs/datasets/detect/open-images-v7.md index 5e8dd17..1d97a8d 100644 --- a/docs/datasets/detect/open-images-v7.md +++ b/docs/datasets/detect/open-images-v7.md @@ -70,7 +70,7 @@ To train a YOLOv8n model on the Open Images V7 dataset for 100 epochs with an im model = YOLO('yolov8n.pt') # Train the model on the Open Images V7 dataset - model.train(data='open-images-v7.yaml', epochs=100, imgsz=640) + results = model.train(data='open-images-v7.yaml', epochs=100, imgsz=640) ``` === "CLI" @@ -94,13 +94,17 @@ Researchers can gain invaluable insights into the array of computer vision chall For those employing Open Images V7 in their work, it's prudent to cite the relevant papers and acknowledge the creators: -```bibtex -@article{OpenImages, - author = {Alina Kuznetsova and Hassan Rom and Neil Alldrin and Jasper Uijlings and Ivan Krasin and Jordi Pont-Tuset and Shahab Kamali and Stefan Popov and Matteo Malloci and Alexander Kolesnikov and Tom Duerig and Vittorio Ferrari}, - title = {The Open Images Dataset V4: Unified image classification, object detection, and visual relationship detection at scale}, - year = {2020}, - journal = {IJCV} -} -``` +!!! note "" + + === "BibTeX" + + ```bibtex + @article{OpenImages, + author = {Alina Kuznetsova and Hassan Rom and Neil Alldrin and Jasper Uijlings and Ivan Krasin and Jordi Pont-Tuset and Shahab Kamali and Stefan Popov and Matteo Malloci and Alexander Kolesnikov and Tom Duerig and Vittorio Ferrari}, + title = {The Open Images Dataset V4: Unified image classification, object detection, and visual relationship detection at scale}, + year = {2020}, + journal = {IJCV} + } + ``` A heartfelt acknowledgment goes out to the Google AI team for creating and maintaining the Open Images V7 dataset. For a deep dive into the dataset and its offerings, navigate to the [official Open Images V7 website](https://storage.googleapis.com/openimages/web/index.html). diff --git a/docs/datasets/detect/sku-110k.md b/docs/datasets/detect/sku-110k.md index ac56f2b..07c3579 100644 --- a/docs/datasets/detect/sku-110k.md +++ b/docs/datasets/detect/sku-110k.md @@ -53,7 +53,7 @@ To train a YOLOv8n model on the SKU-110K dataset for 100 epochs with an image si model = YOLO('yolov8n.pt') # load a pretrained model (recommended for training) # Train the model - model.train(data='SKU-110K.yaml', epochs=100, imgsz=640) + results = model.train(data='SKU-110K.yaml', epochs=100, imgsz=640) ``` === "CLI" @@ -77,13 +77,17 @@ The example showcases the variety and complexity of the data in the SKU-110k dat If you use the SKU-110k dataset in your research or development work, please cite the following paper: -```bibtex -@inproceedings{goldman2019dense, - author = {Eran Goldman and Roei Herzig and Aviv Eisenschtat and Jacob Goldberger and Tal Hassner}, - title = {Precise Detection in Densely Packed Scenes}, - booktitle = {Proc. Conf. Comput. Vision Pattern Recognition (CVPR)}, - year = {2019} -} -``` +!!! note "" + + === "BibTeX" + + ```bibtex + @inproceedings{goldman2019dense, + author = {Eran Goldman and Roei Herzig and Aviv Eisenschtat and Jacob Goldberger and Tal Hassner}, + title = {Precise Detection in Densely Packed Scenes}, + booktitle = {Proc. Conf. Comput. Vision Pattern Recognition (CVPR)}, + year = {2019} + } + ``` We would like to acknowledge Eran Goldman et al. for creating and maintaining the SKU-110k dataset as a valuable resource for the computer vision research community. For more information about the SKU-110k dataset and its creators, visit the [SKU-110k dataset GitHub repository](https://github.com/eg4000/SKU110K_CVPR19). diff --git a/docs/datasets/detect/visdrone.md b/docs/datasets/detect/visdrone.md index 5468d57..be0c956 100644 --- a/docs/datasets/detect/visdrone.md +++ b/docs/datasets/detect/visdrone.md @@ -49,7 +49,7 @@ To train a YOLOv8n model on the VisDrone dataset for 100 epochs with an image si model = YOLO('yolov8n.pt') # load a pretrained model (recommended for training) # Train the model - model.train(data='VisDrone.yaml', epochs=100, imgsz=640) + results = model.train(data='VisDrone.yaml', epochs=100, imgsz=640) ``` === "CLI" @@ -73,16 +73,20 @@ The example showcases the variety and complexity of the data in the VisDrone dat If you use the VisDrone dataset in your research or development work, please cite the following paper: -```bibtex -@ARTICLE{9573394, - author={Zhu, Pengfei and Wen, Longyin and Du, Dawei and Bian, Xiao and Fan, Heng and Hu, Qinghua and Ling, Haibin}, - journal={IEEE Transactions on Pattern Analysis and Machine Intelligence}, - title={Detection and Tracking Meet Drones Challenge}, - year={2021}, - volume={}, - number={}, - pages={1-1}, - doi={10.1109/TPAMI.2021.3119563}} -``` +!!! note "" + + === "BibTeX" + + ```bibtex + @ARTICLE{9573394, + author={Zhu, Pengfei and Wen, Longyin and Du, Dawei and Bian, Xiao and Fan, Heng and Hu, Qinghua and Ling, Haibin}, + journal={IEEE Transactions on Pattern Analysis and Machine Intelligence}, + title={Detection and Tracking Meet Drones Challenge}, + year={2021}, + volume={}, + number={}, + pages={1-1}, + doi={10.1109/TPAMI.2021.3119563}} + ``` We would like to acknowledge the AISKYEYE team at the Lab of Machine Learning and Data Mining, Tianjin University, China, for creating and maintaining the VisDrone dataset as a valuable resource for the drone-based computer vision research community. For more information about the VisDrone dataset and its creators, visit the [VisDrone Dataset GitHub repository](https://github.com/VisDrone/VisDrone-Dataset). diff --git a/docs/datasets/detect/voc.md b/docs/datasets/detect/voc.md index d29da7d..ec18af7 100644 --- a/docs/datasets/detect/voc.md +++ b/docs/datasets/detect/voc.md @@ -52,7 +52,7 @@ To train a YOLOv8n model on the VOC dataset for 100 epochs with an image size of model = YOLO('yolov8n.pt') # load a pretrained model (recommended for training) # Train the model - model.train(data='VOC.yaml', epochs=100, imgsz=640) + results = model.train(data='VOC.yaml', epochs=100, imgsz=640) ``` === "CLI" @@ -77,15 +77,19 @@ The example showcases the variety and complexity of the images in the VOC datase If you use the VOC dataset in your research or development work, please cite the following paper: -```bibtex -@misc{everingham2010pascal, - title={The PASCAL Visual Object Classes (VOC) Challenge}, - author={Mark Everingham and Luc Van Gool and Christopher K. I. Williams and John Winn and Andrew Zisserman}, - year={2010}, - eprint={0909.5206}, - archivePrefix={arXiv}, - primaryClass={cs.CV} -} -``` +!!! note "" + + === "BibTeX" + + ```bibtex + @misc{everingham2010pascal, + title={The PASCAL Visual Object Classes (VOC) Challenge}, + author={Mark Everingham and Luc Van Gool and Christopher K. I. Williams and John Winn and Andrew Zisserman}, + year={2010}, + eprint={0909.5206}, + archivePrefix={arXiv}, + primaryClass={cs.CV} + } + ``` We would like to acknowledge the PASCAL VOC Consortium for creating and maintaining this valuable resource for the computer vision community. For more information about the VOC dataset and its creators, visit the [PASCAL VOC dataset website](http://host.robots.ox.ac.uk/pascal/VOC/). diff --git a/docs/datasets/detect/xview.md b/docs/datasets/detect/xview.md index 9da4ca8..e47268d 100644 --- a/docs/datasets/detect/xview.md +++ b/docs/datasets/detect/xview.md @@ -55,7 +55,7 @@ To train a model on the xView dataset for 100 epochs with an image size of 640, model = YOLO('yolov8n.pt') # load a pretrained model (recommended for training) # Train the model - model.train(data='xView.yaml', epochs=100, imgsz=640) + results = model.train(data='xView.yaml', epochs=100, imgsz=640) ``` === "CLI" @@ -79,15 +79,19 @@ The example showcases the variety and complexity of the data in the xView datase If you use the xView dataset in your research or development work, please cite the following paper: -```bibtex -@misc{lam2018xview, - title={xView: Objects in Context in Overhead Imagery}, - author={Darius Lam and Richard Kuzma and Kevin McGee and Samuel Dooley and Michael Laielli and Matthew Klaric and Yaroslav Bulatov and Brendan McCord}, - year={2018}, - eprint={1802.07856}, - archivePrefix={arXiv}, - primaryClass={cs.CV} -} -``` +!!! note "" + + === "BibTeX" + + ```bibtex + @misc{lam2018xview, + title={xView: Objects in Context in Overhead Imagery}, + author={Darius Lam and Richard Kuzma and Kevin McGee and Samuel Dooley and Michael Laielli and Matthew Klaric and Yaroslav Bulatov and Brendan McCord}, + year={2018}, + eprint={1802.07856}, + archivePrefix={arXiv}, + primaryClass={cs.CV} + } + ``` We would like to acknowledge the [Defense Innovation Unit](https://www.diu.mil/) (DIU) and the creators of the xView dataset for their valuable contribution to the computer vision research community. For more information about the xView dataset and its creators, visit the [xView dataset website](http://xviewdataset.org/). diff --git a/docs/datasets/obb/dota-v2.md b/docs/datasets/obb/dota-v2.md new file mode 100644 index 0000000..d50e014 --- /dev/null +++ b/docs/datasets/obb/dota-v2.md @@ -0,0 +1,129 @@ +--- +comments: true +description: Delve into DOTA v2, an Oriented Bounding Box (OBB) aerial imagery dataset with 1.7 million instances and 11,268 images. +keywords: DOTA v2, object detection, aerial images, computer vision, deep learning, annotations, oriented bounding boxes, OBB +--- + +# DOTA v2 Dataset with OBB + +[DOTA v2](https://captain-whu.github.io/DOTA/index.html) stands as a specialized dataset, emphasizing object detection in aerial images. Originating from the DOTA series of datasets, it offers annotated images capturing a diverse array of aerial scenes with Oriented Bounding Boxes (OBB). + +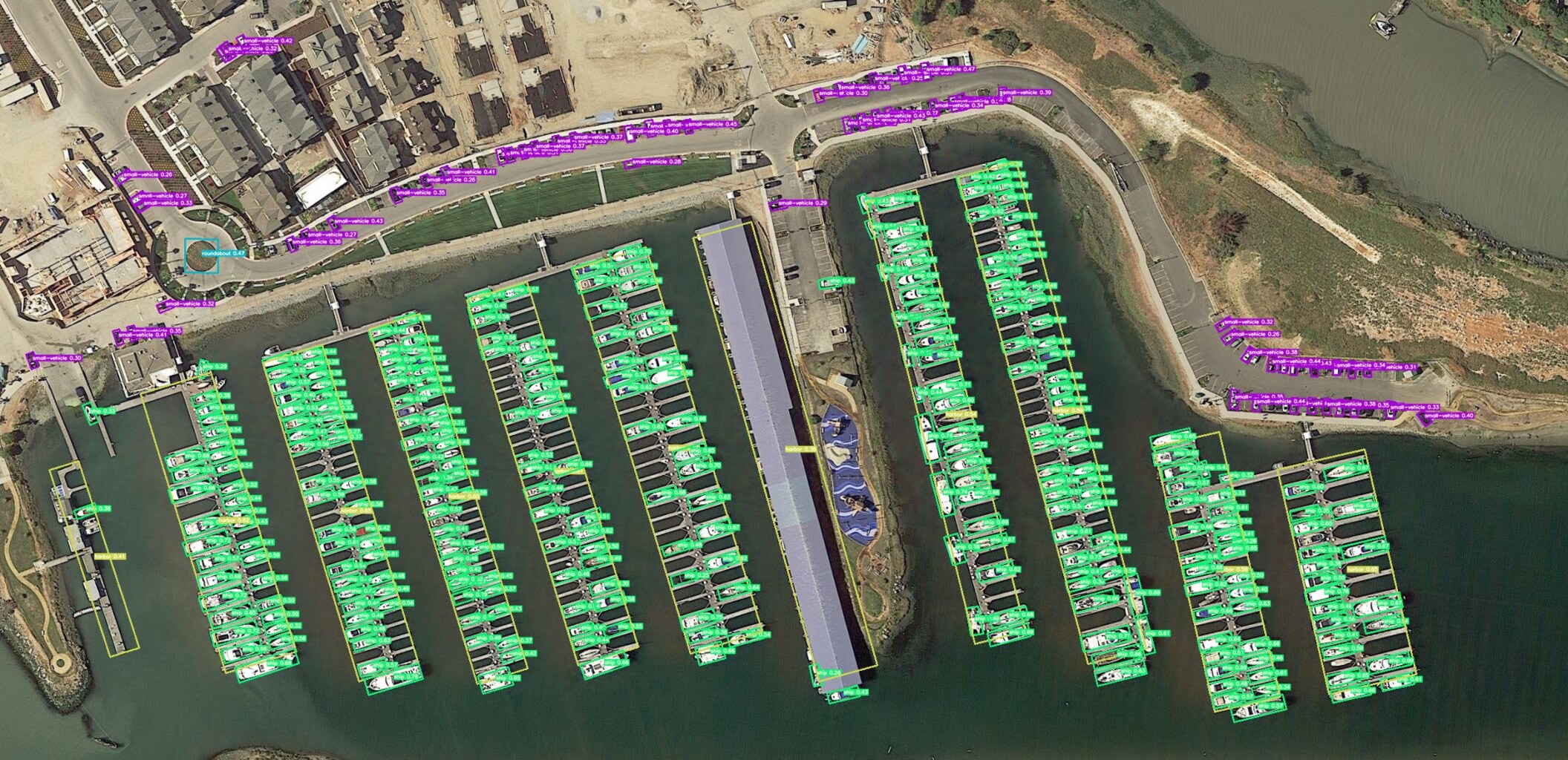 + +## Key Features + +- Collection from various sensors and platforms, with image sizes ranging from 800 × 800 to 20,000 × 20,000 pixels. +- Features more than 1.7M Oriented Bounding Boxes across 18 categories. +- Encompasses multiscale object detection. +- Instances are annotated by experts using arbitrary (8 d.o.f.) quadrilateral, capturing objects of different scales, orientations, and shapes. + +## Dataset Versions + +### DOTA-v1.0 + +- Contains 15 common categories. +- Comprises 2,806 images with 188,282 instances. +- Split ratios: 1/2 for training, 1/6 for validation, and 1/3 for testing. + +### DOTA-v1.5 + +- Incorporates the same images as DOTA-v1.0. +- Very small instances (less than 10 pixels) are also annotated. +- Addition of a new category: "container crane". +- A total of 403,318 instances. +- Released for the DOAI Challenge 2019 on Object Detection in Aerial Images. + +### DOTA-v2.0 + +- Collections from Google Earth, GF-2 Satellite, and other aerial images. +- Contains 18 common categories. +- Comprises 11,268 images with a whopping 1,793,658 instances. +- New categories introduced: "airport" and "helipad". +- Image splits: + - Training: 1,830 images with 268,627 instances. + - Validation: 593 images with 81,048 instances. + - Test-dev: 2,792 images with 353,346 instances. + - Test-challenge: 6,053 images with 1,090,637 instances. + +## Dataset Structure + +DOTA v2 exhibits a structured layout tailored for OBB object detection challenges: + +- **Images**: A vast collection of high-resolution aerial images capturing diverse terrains and structures. +- **Oriented Bounding Boxes**: Annotations in the form of rotated rectangles encapsulating objects irrespective of their orientation, ideal for capturing objects like airplanes, ships, and buildings. + +## Applications + +DOTA v2 serves as a benchmark for training and evaluating models specifically tailored for aerial image analysis. With the inclusion of OBB annotations, it provides a unique challenge, enabling the development of specialized object detection models that cater to aerial imagery's nuances. + +## Dataset YAML + +Typically, datasets incorporate a YAML (Yet Another Markup Language) file detailing the dataset's configuration. For DOTA v2, a hypothetical `DOTAv2.yaml` could be used. For accurate paths and configurations, it's vital to consult the dataset's official repository or documentation. + +!!! example "DOTAv2.yaml" + + ```yaml + --8<-- "ultralytics/cfg/datasets/DOTAv2.yaml" + ``` + +## Usage + +To train a model on the DOTA v2 dataset, you can utilize the following code snippets. Always refer to your model's documentation for a thorough list of available arguments. + +!!! warning + + Please note that all images and associated annotations in the DOTAv2 dataset can be used for academic purposes, but commercial use is prohibited. Your understanding and respect for the dataset creators' wishes are greatly appreciated! + +!!! example "Train Example" + + === "Python" + + ```python + from ultralytics import YOLO + + # Create a new YOLOv8n-OBB model from scratch + model = YOLO('yolov8n-obb.yaml') + + # Train the model on the DOTAv2 dataset + results = model.train(data='DOTAv2.yaml', epochs=100, imgsz=640) + ``` + + === "CLI" + + ```bash + # Train a new YOLOv8n-OBB model on the DOTAv2 dataset + yolo detect train data=DOTAv2.yaml model=yolov8n.pt epochs=100 imgsz=640 + ``` + +## Sample Data and Annotations + +Having a glance at the dataset illustrates its depth: + +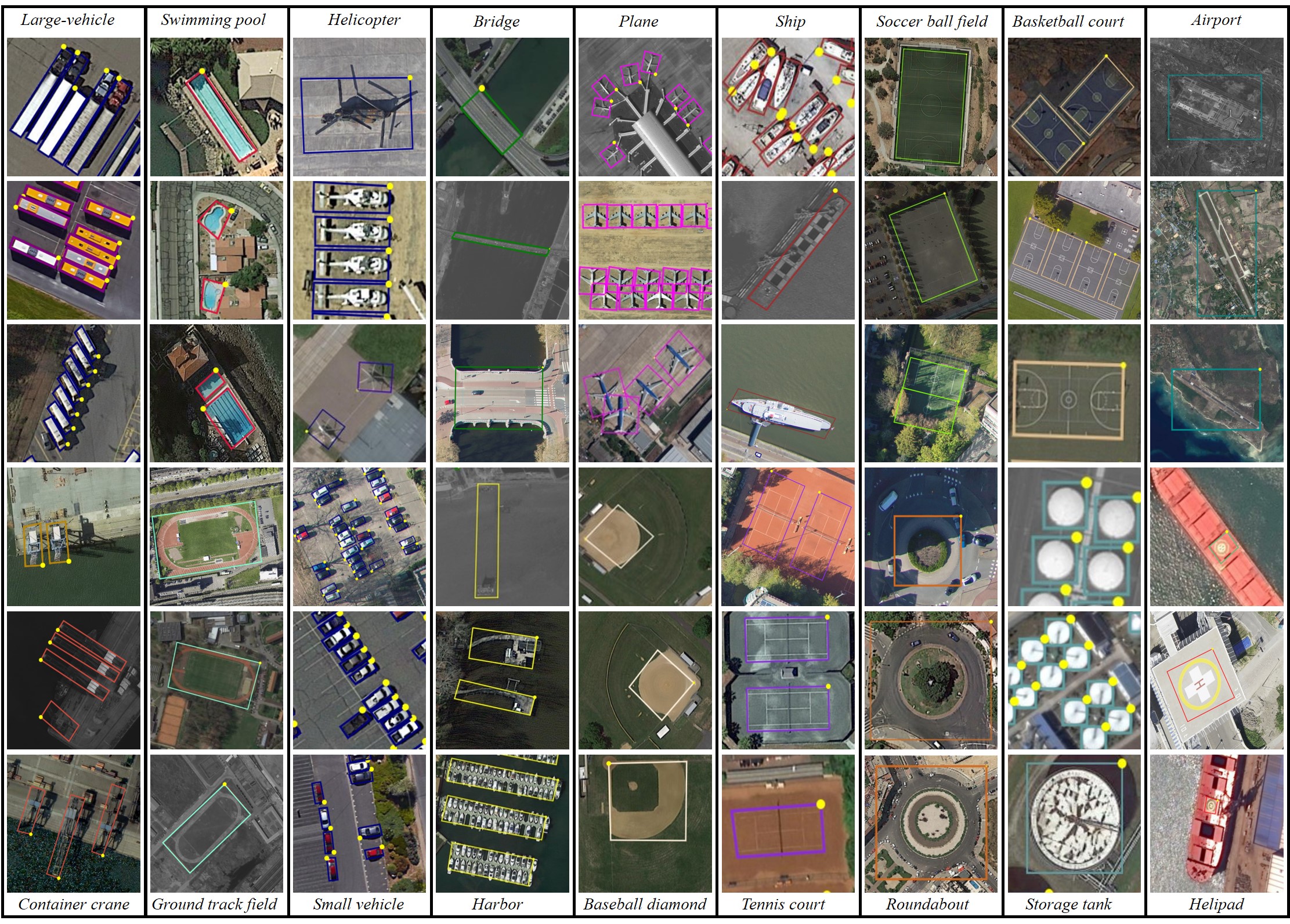 + +- **DOTA v2**: This snapshot underlines the complexity of aerial scenes and the significance of Oriented Bounding Box annotations, capturing objects in their natural orientation. + +The dataset's richness offers invaluable insights into object detection challenges exclusive to aerial imagery. + +## Citations and Acknowledgments + +For those leveraging DOTA v2 in their endeavors, it's pertinent to cite the relevant research papers: + +!!! note "" + + === "BibTeX" + + ```bibtex + @article{9560031, + author={Ding, Jian and Xue, Nan and Xia, Gui-Song and Bai, Xiang and Yang, Wen and Yang, Michael and Belongie, Serge and Luo, Jiebo and Datcu, Mihai and Pelillo, Marcello and Zhang, Liangpei}, + journal={IEEE Transactions on Pattern Analysis and Machine Intelligence}, + title={Object Detection in Aerial Images: A Large-Scale Benchmark and Challenges}, + year={2021}, + volume={}, + number={}, + pages={1-1}, + doi={10.1109/TPAMI.2021.3117983} + } + ``` + +A special note of gratitude to the team behind DOTA v2 for their commendable effort in curating this dataset. For an exhaustive understanding of the dataset and its nuances, please visit the [official DOTA v2 website](https://captain-whu.github.io/DOTA/index.html). \ No newline at end of file diff --git a/docs/datasets/obb/index.md b/docs/datasets/obb/index.md new file mode 100644 index 0000000..1e1f544 --- /dev/null +++ b/docs/datasets/obb/index.md @@ -0,0 +1,80 @@ +--- +comments: true +description: Dive deep into various oriented bounding box (OBB) dataset formats compatible with the Ultralytics YOLO model. Grasp the nuances of using and converting datasets to this format. +keywords: Ultralytics, YOLO, oriented bounding boxes, OBB, dataset formats, label formats, DOTA v2, data conversion +--- + +# Oriented Bounding Box Datasets Overview + +Training a precise object detection model with oriented bounding boxes (OBB) requires a thorough dataset. This guide elucidates the various OBB dataset formats compatible with the Ultralytics YOLO model, offering insights into their structure, application, and methods for format conversions. + +## Supported OBB Dataset Formats + +### YOLO OBB Format + +The YOLO OBB format designates bounding boxes by their four corner points with coordinates normalized between 0 and 1. It follows this format: + +```bash +class_index, x1, y1, x2, y2, x3, y3, x4, y4 +``` + +Internally, YOLO processes losses and outputs in the `xywhr` format, which represents the bounding box's center point (xy), width, height, and rotation. + +