diff --git a/docker/Dockerfile-jetson b/docker/Dockerfile-jetson
index 8b4323d..08f21f2 100644
--- a/docker/Dockerfile-jetson
+++ b/docker/Dockerfile-jetson
@@ -28,6 +28,12 @@ RUN python3 -m pip install --upgrade pip wheel
RUN pip install --no-cache tqdm matplotlib pyyaml psutil thop pandas onnx "numpy==1.23"
RUN pip install --no-cache -e .
+# Resolve duplicate OpenCV installation issues in https://github.com/ultralytics/ultralytics/issues/2407
+RUN apt-get remove `dpkg -l | grep opencv | awk '{print $2}'`
+RUN pip uninstall -y opencv-python
+RUN rm /usr/local/lib/python3.8/dist-packages/cv2 # Optional
+RUN pip install "opencv-python<4.7"
+
# Set environment variables
ENV OMP_NUM_THREADS=1
diff --git a/docs/datasets/detect/coco.md b/docs/datasets/detect/coco.md
index 19480d2..e516a39 100644
--- a/docs/datasets/detect/coco.md
+++ b/docs/datasets/detect/coco.md
@@ -2,6 +2,87 @@
comments: true
---
-# 🚧 Page Under Construction ⚒
+# COCO Dataset
-This page is currently under construction!️ 👷Please check back later for updates. 😃🔜
+The [COCO](https://cocodataset.org/#home) (Common Objects in Context) dataset is a large-scale object detection, segmentation, and captioning dataset. It is designed to encourage research on a wide variety of object categories and is commonly used for benchmarking computer vision models. It is an essential dataset for researchers and developers working on object detection, segmentation, and pose estimation tasks.
+
+## Key Features
+
+- COCO contains 330K images, with 200K images having annotations for object detection, segmentation, and captioning tasks.
+- The dataset comprises 80 object categories, including common objects like cars, bicycles, and animals, as well as more specific categories such as umbrellas, handbags, and sports equipment.
+- Annotations include object bounding boxes, segmentation masks, and captions for each image.
+- COCO provides standardized evaluation metrics like mean Average Precision (mAP) for object detection, and mean Average Recall (mAR) for segmentation tasks, making it suitable for comparing model performance.
+
+## Dataset Structure
+
+The COCO dataset is split into three subsets:
+
+1. **Train2017**: This subset contains 118K images for training object detection, segmentation, and captioning models.
+2. **Val2017**: This subset has 5K images used for validation purposes during model training.
+3. **Test2017**: This subset consists of 20K images used for testing and benchmarking the trained models. Ground truth annotations for this subset are not publicly available, and the results are submitted to the [COCO evaluation server](https://competitions.codalab.org/competitions/5181) for performance evaluation.
+
+## Applications
+
+The COCO dataset is widely used for training and evaluating deep learning models in object detection (such as YOLO, Faster R-CNN, and SSD), instance segmentation (such as Mask R-CNN), and keypoint detection (such as OpenPose). The dataset's diverse set of object categories, large number of annotated images, and standardized evaluation metrics make it an essential resource for computer vision researchers and practitioners.
+
+## Dataset YAML
+
+A YAML (Yet Another Markup Language) file is used to define the dataset configuration. It contains information about the dataset's paths, classes, and other relevant information. In the case of the COCO dataset, the `coco.yaml` file is maintained at [https://github.com/ultralytics/ultralytics/blob/main/ultralytics/datasets/coco.yaml](https://github.com/ultralytics/ultralytics/blob/main/ultralytics/datasets/coco.yaml).
+
+!!! example "ultralytics/datasets/coco.yaml"
+
+ ```yaml
+ --8<-- "ultralytics/datasets/coco.yaml"
+ ```
+
+## Usage
+
+To train a YOLOv8n model on the COCO dataset for 100 epochs with an image size of 640, you can use the following code snippets. For a comprehensive list of available arguments, refer to the model [Training](../../modes/train.md) page.
+
+!!! example "Train Example"
+
+ === "Python"
+
+ ```python
+ from ultralytics import YOLO
+
+ # Load a model
+ model = YOLO('yolov8n.pt') # load a pretrained model (recommended for training)
+
+ # Train the model
+ model.train(data='coco.yaml', epochs=100, imgsz=640)
+ ```
+
+ === "CLI"
+
+ ```bash
+ # Start training from a pretrained *.pt model
+ yolo detect train data=coco.yaml model=yolov8n.pt epochs=100 imgsz=640
+ ```
+
+## Sample Images and Annotations
+
+The COCO dataset contains a diverse set of images with various object categories and complex scenes. Here are some examples of images from the dataset, along with their corresponding annotations:
+
+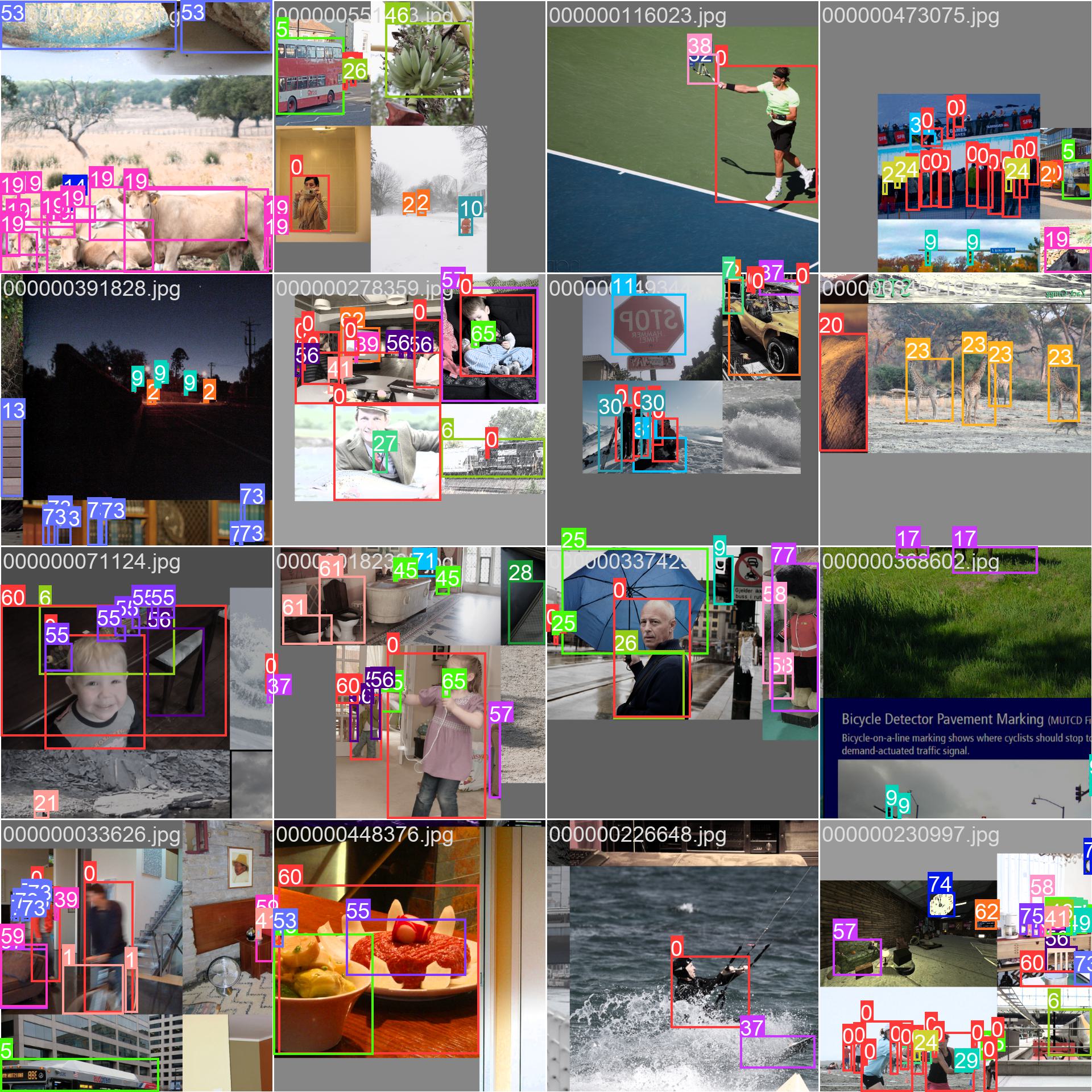
+
+- **Mosaiced Image**: This image demonstrates a training batch composed of mosaiced dataset images. Mosaicing is a technique used during training that combines multiple images into a single image to increase the variety of objects and scenes within each training batch. This helps improve the model's ability to generalize to different object sizes, aspect ratios, and contexts.
+
+The example showcases the variety and complexity of the images in the COCO dataset and the benefits of using mosaicing during the training process.
+
+## Citations and Acknowledgments
+
+If you use the COCO dataset in your research or development work, please cite the following paper:
+
+```bibtex
+@misc{lin2015microsoft,
+ title={Microsoft COCO: Common Objects in Context},
+ author={Tsung-Yi Lin and Michael Maire and Serge Belongie and Lubomir Bourdev and Ross Girshick and James Hays and Pietro Perona and Deva Ramanan and C. Lawrence Zitnick and Piotr Dollár},
+ year={2015},
+ eprint={1405.0312},
+ archivePrefix={arXiv},
+ primaryClass={cs.CV}
+}
+```
+
+We would like to acknowledge the COCO Consortium for creating and maintaining this valuable resource for the computer vision community. For more information about the COCO dataset and its creators, visit the [COCO dataset website](https://cocodataset.org/#home).
\ No newline at end of file
diff --git a/docs/modes/track.md b/docs/modes/track.md
index a0d7999..80c477f 100644
--- a/docs/modes/track.md
+++ b/docs/modes/track.md
@@ -1,5 +1,6 @@
---
comments: true
+description: Explore YOLOv8n-based object tracking with Ultralytics' BoT-SORT and ByteTrack. Learn configuration, usage, and customization tips.
---
diff --git a/docs/modes/train.md b/docs/modes/train.md
index 7a5a02d..32186cd 100644
--- a/docs/modes/train.md
+++ b/docs/modes/train.md
@@ -1,5 +1,6 @@
---
comments: true
+description: Learn how to train custom YOLOv8 models on various datasets, configure hyperparameters, and use Ultralytics' YOLO for seamless training.
---
diff --git a/docs/stylesheets/style.css b/docs/stylesheets/style.css
index 85f766c..8a13d2b 100644
--- a/docs/stylesheets/style.css
+++ b/docs/stylesheets/style.css
@@ -31,3 +31,8 @@ th, td {
background-color: hsla(var(--md-hue),25%,25%,1)
}
/* Table format like GitHub ----------------------------------------------------------------------------------------- */
+
+/* Code block vertical scroll */
+.md-typeset pre > code {
+ max-height: 20rem;
+}
\ No newline at end of file
diff --git a/docs/tasks/detect.md b/docs/tasks/detect.md
index 3365529..8ed02e0 100644
--- a/docs/tasks/detect.md
+++ b/docs/tasks/detect.md
@@ -6,10 +6,7 @@ Object detection is a task that involves identifying the location and class of o
-The output of an object detector is a set of bounding boxes that enclose the objects in the image, along with class
-labels
-and confidence scores for each box. Object detection is a good choice when you need to identify objects of interest in a
-scene, but don't need to know exactly where the object is or its exact shape.
+The output of an object detector is a set of bounding boxes that enclose the objects in the image, along with class labels and confidence scores for each box. Object detection is a good choice when you need to identify objects of interest in a scene, but don't need to know exactly where the object is or its exact shape.
!!! tip "Tip"
@@ -17,13 +14,9 @@ scene, but don't need to know exactly where the object is or its exact shape.
## [Models](https://github.com/ultralytics/ultralytics/tree/main/ultralytics/models/v8)
-YOLOv8 pretrained Detect models are shown here. Detect, Segment and Pose models are pretrained on
-the [COCO](https://github.com/ultralytics/ultralytics/blob/main/ultralytics/datasets/coco.yaml) dataset, while Classify
-models are pretrained on
-the [ImageNet](https://github.com/ultralytics/ultralytics/blob/main/ultralytics/datasets/ImageNet.yaml) dataset.
+YOLOv8 pretrained Detect models are shown here. Detect, Segment and Pose models are pretrained on the [COCO](https://github.com/ultralytics/ultralytics/blob/main/ultralytics/datasets/coco.yaml) dataset, while Classify models are pretrained on the [ImageNet](https://github.com/ultralytics/ultralytics/blob/main/ultralytics/datasets/ImageNet.yaml) dataset.
-[Models](https://github.com/ultralytics/ultralytics/tree/main/ultralytics/models) download automatically from the latest
-Ultralytics [release](https://github.com/ultralytics/assets/releases) on first use.
+[Models](https://github.com/ultralytics/ultralytics/tree/main/ultralytics/models) download automatically from the latest Ultralytics [release](https://github.com/ultralytics/assets/releases) on first use.
| Model | size
(pixels) | mAPval
50-95 | Speed
CPU ONNX
(ms) | Speed
A100 TensorRT
(ms) | params
(M) | FLOPs
(B) |
|--------------------------------------------------------------------------------------|-----------------------|----------------------|--------------------------------|-------------------------------------|--------------------|-------------------|
@@ -41,8 +34,7 @@ Ultralytics [release](https://github.com/ultralytics/assets/releases) on first u
## Train
-Train YOLOv8n on the COCO128 dataset for 100 epochs at image size 640. For a full list of available arguments see
-the [Configuration](../usage/cfg.md) page.
+Train YOLOv8n on the COCO128 dataset for 100 epochs at image size 640. For a full list of available arguments see the [Configuration](../usage/cfg.md) page.
!!! example ""
@@ -71,15 +63,14 @@ the [Configuration](../usage/cfg.md) page.
# Build a new model from YAML, transfer pretrained weights to it and start training
yolo detect train data=coco128.yaml model=yolov8n.yaml pretrained=yolov8n.pt epochs=100 imgsz=640
```
+
### Dataset format
-YOLO detection dataset format can be found in detail in the [Dataset Guide](../yolov5/tutorials/train_custom_data.md).
-To convert your existing dataset from other formats( like COCO, VOC etc.) to YOLO format, please use [json2yolo tool](https://github.com/ultralytics/JSON2YOLO) by Ultralytics.
+YOLO detection dataset format can be found in detail in the [Dataset Guide](../yolov5/tutorials/train_custom_data.md). To convert your existing dataset from other formats( like COCO, VOC etc.) to YOLO format, please use [json2yolo tool](https://github.com/ultralytics/JSON2YOLO) by Ultralytics.
## Val
-Validate trained YOLOv8n model accuracy on the COCO128 dataset. No argument need to passed as the `model` retains it's
-training `data` and arguments as model attributes.
+Validate trained YOLOv8n model accuracy on the COCO128 dataset. No argument need to passed as the `model` retains it's training `data` and arguments as model attributes.
!!! example ""
@@ -158,8 +149,7 @@ Export a YOLOv8n model to a different format like ONNX, CoreML, etc.
yolo export model=path/to/best.pt format=onnx # export custom trained model
```
-Available YOLOv8 export formats are in the table below. You can predict or validate directly on exported models,
-i.e. `yolo predict model=yolov8n.onnx`. Usage examples are shown for your model after export completes.
+Available YOLOv8 export formats are in the table below. You can predict or validate directly on exported models, i.e. `yolo predict model=yolov8n.onnx`. Usage examples are shown for your model after export completes.
| Format | `format` Argument | Model | Metadata | Arguments |
|--------------------------------------------------------------------|-------------------|---------------------------|----------|-----------------------------------------------------|
diff --git a/mkdocs.yml b/mkdocs.yml
index cb85112..302b4ba 100644
--- a/mkdocs.yml
+++ b/mkdocs.yml
@@ -2,6 +2,8 @@
site_name: Ultralytics YOLOv8 Docs
site_url: https://docs.ultralytics.com
+site_description: The official documentation for Ultralytics YOLOv8, providing comprehensive guides, tutorials, and references to get you started on your AI journey.
+site_author: Ultralytics
repo_url: https://github.com/ultralytics/ultralytics
edit_uri: https://github.com/ultralytics/ultralytics/tree/main/docs
repo_name: ultralytics/ultralytics
@@ -107,7 +109,8 @@ markdown_extensions:
- pymdownx.highlight:
anchor_linenums: true
- pymdownx.inlinehilite
- - pymdownx.snippets
+ - pymdownx.snippets:
+ base_path: ./
- pymdownx.emoji:
emoji_index: !!python/name:materialx.emoji.twemoji # noqa
emoji_generator: !!python/name:materialx.emoji.to_svg
@@ -334,6 +337,7 @@ nav:
plugins:
- mkdocstrings
- search
+ - ultralytics
- git-revision-date-localized:
type: timeago
enable_creation_date: true
diff --git a/setup.py b/setup.py
index 82a530d..6418e08 100644
--- a/setup.py
+++ b/setup.py
@@ -24,7 +24,8 @@ setup(
version=get_version(), # version of pypi package
python_requires='>=3.7',
license='AGPL-3.0',
- description='Ultralytics YOLOv8',
+ description=('Ultralytics YOLOv8 for SOTA object detection, multi-object tracking, instance segmentation, '
+ 'pose estimation and image classification.'),
long_description=README,
long_description_content_type='text/markdown',
url='https://github.com/ultralytics/ultralytics',
@@ -47,6 +48,7 @@ setup(
'mkdocstrings[python]',
'mkdocs-redirects', # for 301 redirects
'mkdocs-git-revision-date-localized-plugin', # for created/updated dates
+ 'mkdocs-ultralytics-plugin', # for meta descriptions and images
],
'export': ['coremltools>=6.0', 'openvino-dev>=2022.3', 'tensorflowjs'], # automatically installs tensorflow
},
diff --git a/ultralytics/__init__.py b/ultralytics/__init__.py
index 5a99b5c..1f3f9c8 100644
--- a/ultralytics/__init__.py
+++ b/ultralytics/__init__.py
@@ -1,6 +1,6 @@
# Ultralytics YOLO 🚀, AGPL-3.0 license
-__version__ = '8.0.94'
+__version__ = '8.0.96'
from ultralytics.hub import start
from ultralytics.vit.sam import SAM
diff --git a/ultralytics/yolo/utils/callbacks/clearml.py b/ultralytics/yolo/utils/callbacks/clearml.py
index f188649..094ad10 100644
--- a/ultralytics/yolo/utils/callbacks/clearml.py
+++ b/ultralytics/yolo/utils/callbacks/clearml.py
@@ -84,9 +84,15 @@ def on_pretrain_routine_start(trainer):
def on_train_epoch_end(trainer):
- """Logs debug samples for the first epoch of YOLO training."""
- if trainer.epoch == 1 and Task.current_task():
- _log_debug_samples(sorted(trainer.save_dir.glob('train_batch*.jpg')), 'Mosaic')
+ task = Task.current_task()
+
+ if task:
+ """Logs debug samples for the first epoch of YOLO training."""
+ if trainer.epoch == 1:
+ _log_debug_samples(sorted(trainer.save_dir.glob('train_batch*.jpg')), 'Mosaic')
+ """Report the current training progress."""
+ for k, v in trainer.validator.metrics.results_dict.items():
+ task.get_logger().report_scalar('train', k, v, iteration=trainer.epoch)
def on_fit_epoch_end(trainer):
@@ -119,7 +125,9 @@ def on_train_end(trainer):
task = Task.current_task()
if task:
# Log final results, CM matrix + PR plots
- files = ['results.png', 'confusion_matrix.png', *(f'{x}_curve.png' for x in ('F1', 'PR', 'P', 'R'))]
+ files = [
+ 'results.png', 'confusion_matrix.png', 'confusion_matrix_normalized.png',
+ *(f'{x}_curve.png' for x in ('F1', 'PR', 'P', 'R'))]
files = [(trainer.save_dir / f) for f in files if (trainer.save_dir / f).exists()] # filter
for f in files:
_log_plot(title=f.stem, plot_path=f)
diff --git a/ultralytics/yolo/utils/callbacks/neptune.py b/ultralytics/yolo/utils/callbacks/neptune.py
index ca72b6b..1355d81 100644
--- a/ultralytics/yolo/utils/callbacks/neptune.py
+++ b/ultralytics/yolo/utils/callbacks/neptune.py
@@ -87,7 +87,9 @@ def on_train_end(trainer):
"""Callback function called at end of training."""
if run:
# Log final results, CM matrix + PR plots
- files = ['results.png', 'confusion_matrix.png', *(f'{x}_curve.png' for x in ('F1', 'PR', 'P', 'R'))]
+ files = [
+ 'results.png', 'confusion_matrix.png', 'confusion_matrix_normalized.png',
+ *(f'{x}_curve.png' for x in ('F1', 'PR', 'P', 'R'))]
files = [(trainer.save_dir / f) for f in files if (trainer.save_dir / f).exists()] # filter
for f in files:
_log_plot(title=f.stem, plot_path=f)
diff --git a/ultralytics/yolo/utils/metrics.py b/ultralytics/yolo/utils/metrics.py
index c446600..17ef280 100644
--- a/ultralytics/yolo/utils/metrics.py
+++ b/ultralytics/yolo/utils/metrics.py
@@ -321,17 +321,18 @@ class ConfusionMatrix:
ticklabels = (list(names) + ['background']) if labels else 'auto'
with warnings.catch_warnings():
warnings.simplefilter('ignore') # suppress empty matrix RuntimeWarning: All-NaN slice encountered
- sn.heatmap(array,
- ax=ax,
- annot=nc < 30,
- annot_kws={
- 'size': 8},
- cmap='Blues',
- fmt='.2f',
- square=True,
- vmin=0.0,
- xticklabels=ticklabels,
- yticklabels=ticklabels).set_facecolor((1, 1, 1))
+ sn.heatmap(
+ array,
+ ax=ax,
+ annot=nc < 30,
+ annot_kws={
+ 'size': 8},
+ cmap='Blues',
+ fmt='.2f' if normalize else '%d', # float if normalize else integer
+ square=True,
+ vmin=0.0,
+ xticklabels=ticklabels,
+ yticklabels=ticklabels).set_facecolor((1, 1, 1))
title = 'Confusion Matrix' + ' Normalized' * normalize
ax.set_xlabel('True')
ax.set_ylabel('Predicted')
diff --git a/ultralytics/yolo/utils/tal.py b/ultralytics/yolo/utils/tal.py
index d0ceb6c..0b27ddd 100644
--- a/ultralytics/yolo/utils/tal.py
+++ b/ultralytics/yolo/utils/tal.py
@@ -2,7 +2,6 @@
import torch
import torch.nn as nn
-import torch.nn.functional as F
from .checks import check_version
from .metrics import bbox_iou
@@ -44,9 +43,11 @@ def select_highest_overlaps(mask_pos, overlaps, n_max_boxes):
if fg_mask.max() > 1: # one anchor is assigned to multiple gt_bboxes
mask_multi_gts = (fg_mask.unsqueeze(1) > 1).repeat([1, n_max_boxes, 1]) # (b, n_max_boxes, h*w)
max_overlaps_idx = overlaps.argmax(1) # (b, h*w)
- is_max_overlaps = F.one_hot(max_overlaps_idx, n_max_boxes) # (b, h*w, n_max_boxes)
- is_max_overlaps = is_max_overlaps.permute(0, 2, 1).to(overlaps.dtype) # (b, n_max_boxes, h*w)
- mask_pos = torch.where(mask_multi_gts, is_max_overlaps, mask_pos) # (b, n_max_boxes, h*w)
+
+ is_max_overlaps = torch.zeros(mask_pos.shape, dtype=mask_pos.dtype, device=mask_pos.device)
+ is_max_overlaps.scatter_(1, max_overlaps_idx.unsqueeze(1), 1)
+
+ mask_pos = torch.where(mask_multi_gts, is_max_overlaps, mask_pos).float() # (b, n_max_boxes, h*w)
fg_mask = mask_pos.sum(-2)
# Find each grid serve which gt(index)
target_gt_idx = mask_pos.argmax(-2) # (b, h*w)
@@ -175,21 +176,23 @@ class TaskAlignedAssigner(nn.Module):
(Tensor): A tensor of shape (b, max_num_obj, h*w) containing the selected top-k candidates.
"""
- num_anchors = metrics.shape[-1] # h*w
# (b, max_num_obj, topk)
topk_metrics, topk_idxs = torch.topk(metrics, self.topk, dim=-1, largest=largest)
if topk_mask is None:
- topk_mask = (topk_metrics.max(-1, keepdim=True) > self.eps).tile([1, 1, self.topk])
+ topk_mask = (topk_metrics.max(-1, keepdim=True)[0] > self.eps).expand_as(topk_idxs)
# (b, max_num_obj, topk)
- topk_idxs[~topk_mask] = 0
+ topk_idxs.masked_fill_(~topk_mask, 0)
+
# (b, max_num_obj, topk, h*w) -> (b, max_num_obj, h*w)
- is_in_topk = torch.zeros(metrics.shape, dtype=torch.long, device=metrics.device)
- for it in range(self.topk):
- is_in_topk += F.one_hot(topk_idxs[:, :, it], num_anchors)
- # is_in_topk = F.one_hot(topk_idxs, num_anchors).sum(-2)
+ count_tensor = torch.zeros(metrics.shape, dtype=torch.int8, device=topk_idxs.device)
+ ones = torch.ones_like(topk_idxs[:, :, :1], dtype=torch.int8, device=topk_idxs.device)
+ for k in range(self.topk):
+ # Expand topk_idxs for each value of k and add 1 at the specified positions
+ count_tensor.scatter_add_(-1, topk_idxs[:, :, k:k + 1], ones)
# filter invalid bboxes
- is_in_topk = torch.where(is_in_topk > 1, 0, is_in_topk)
- return is_in_topk.to(metrics.dtype)
+ count_tensor.masked_fill_(count_tensor > 1, 0)
+
+ return count_tensor.to(metrics.dtype)
def get_targets(self, gt_labels, gt_bboxes, target_gt_idx, fg_mask):
"""
@@ -226,7 +229,13 @@ class TaskAlignedAssigner(nn.Module):
# Assigned target scores
target_labels.clamp_(0)
- target_scores = F.one_hot(target_labels, self.num_classes) # (b, h*w, 80)
+
+ # 10x faster than F.one_hot()
+ target_scores = torch.zeros((target_labels.shape[0], target_labels.shape[1], self.num_classes),
+ dtype=torch.int64,
+ device=target_labels.device) # (b, h*w, 80)
+ target_scores.scatter_(2, target_labels.unsqueeze(-1), 1)
+
fg_scores_mask = fg_mask[:, :, None].repeat(1, 1, self.num_classes) # (b, h*w, 80)
target_scores = torch.where(fg_scores_mask > 0, target_scores, 0)